Using SHAP Values to Validate Model's Uncertain Decision for ML-based Lightpath Quality-of-Transmission Estimation.
ICTON(2023)
摘要
We apply Quantile Regression (QR) for lightpath quality-of-transmission (QoT) estimation with the aim of identifying uncertain decisions and then exploit Shapley Additive Explanations (SHAP) to quantify lightpath features’ importance by means of SHAP values and validate the model’s decisions in a post-processing phase. Numerical results show that our approach can eliminate more than 98% of false predictions and that SHAP values can validate up to 90% of the model's uncertain decisions.
更多查看译文
关键词
lightpath QoT estimation,uncertainty quantification,explainable artificial intelligence
AI 理解论文
溯源树
样例
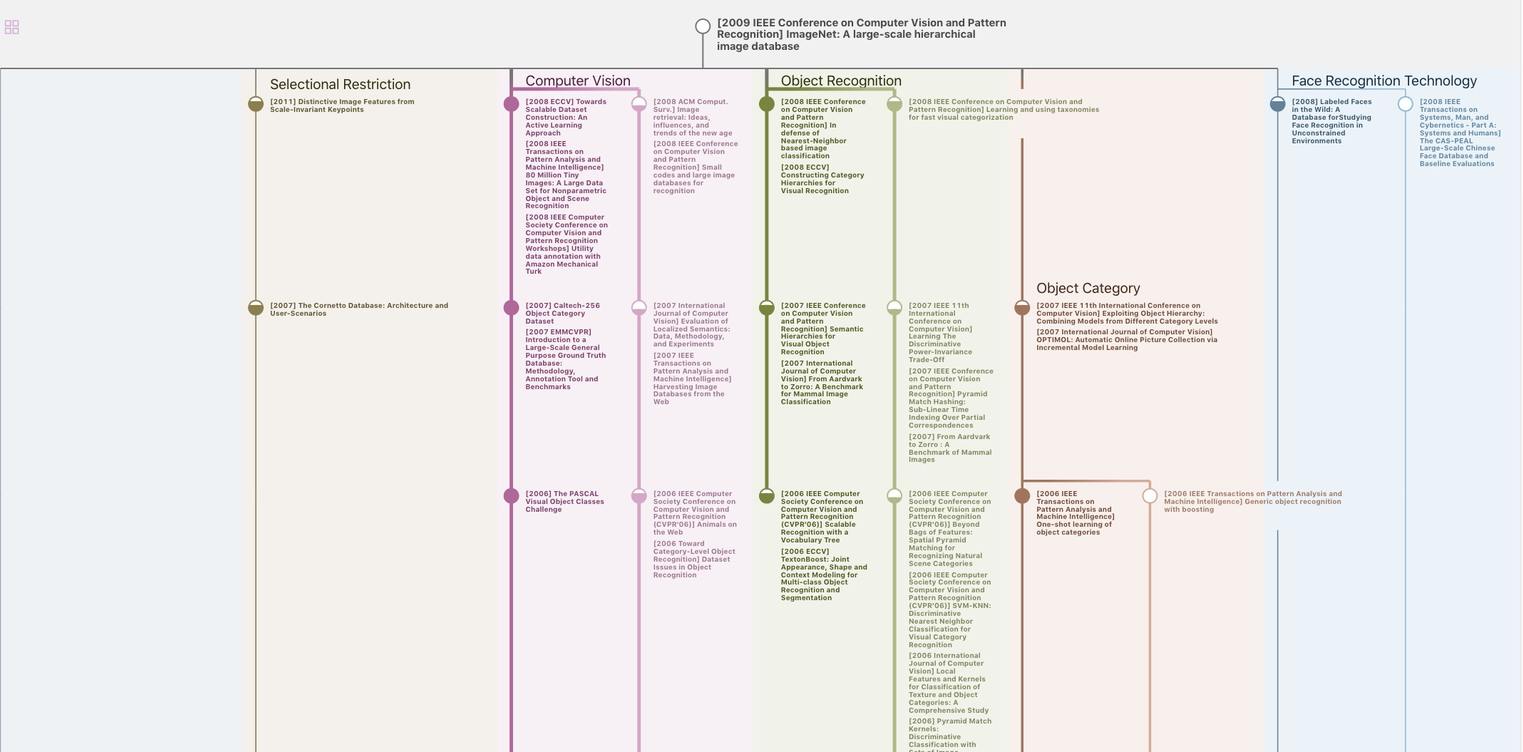
生成溯源树,研究论文发展脉络
Chat Paper
正在生成论文摘要