WEATHERGOV+: A Table Recognition and Summarization Dataset to Bridge the Gap Between Document Image Analysis and Natural Language Generation
DocEng(2023)
摘要
Tables, ubiquitous in data-oriented documents like scientific papers and financial statements, organize and convey relational information. Automatic table recognition from document images, which involves detection within the page, structural segmentation into rows, columns, and cells, and information extraction from cells, has been a popular research topic in document image analysis (DIA). With recent advances in natural language generation (NLG) based on deep neural networks, data-to-text generation, in particular for table summarization, offers interesting solutions to time-intensive data analysis. In this paper, we aim to bridge the gap between efforts in DIA and NLG regarding tabular data: we propose WEATHERGOV+, a dataset building upon the WEATHERGOV dataset, the standard for tabular data summarization techniques, that allows for the training and testing of end-to-end methods working from input document images to generate text summaries as output. WEATHERGOV+ contains images of tables created from the tabular data of WEATHERGOV using visual variations that cover various levels of difficulty, along with the corresponding human-generated table summaries of WEATHERGOV. We also propose an end-to-end pipeline that compares state-of-the-art table recognition methods for summarization purposes. We analyse the results of the proposed pipeline by evaluating WEATHERGOV+ at each stage of the pipeline to identify the effects of error propagation and the weaknesses of the current methods, such as OCR errors. With this research (dataset and code available here1), we hope to encourage new research for the processing and management of inter- and intra-document collections.
更多查看译文
AI 理解论文
溯源树
样例
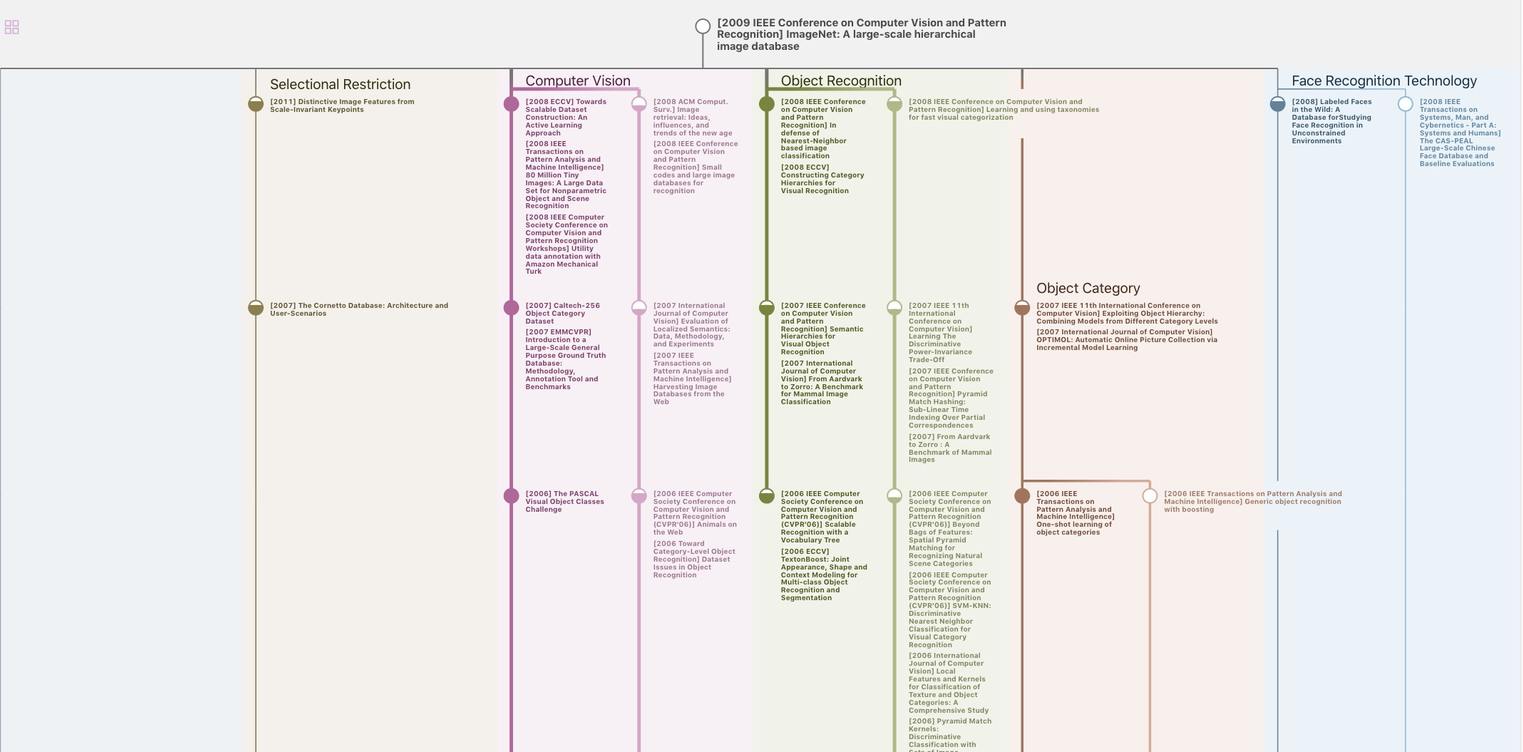
生成溯源树,研究论文发展脉络
Chat Paper
正在生成论文摘要