Compacting MocapNET-based 3D Human Pose Estimation via Dimensionality Reduction
PROCEEDINGS OF THE 16TH ACM INTERNATIONAL CONFERENCE ON PERVASIVE TECHNOLOGIES RELATED TO ASSISTIVE ENVIRONMENTS, PETRA 2023(2023)
摘要
MocapNETs are state of the art Neural Network (NN) ensembles that estimate 3D human pose based on visual input in the form of an RGB image. They do so by deriving a 3D Bio Vision Hierarchy (BVH) skeleton from estimated 2D human body joint projections. BVH output makes MocapNETs directly compatible with a large variety of 3D graphics engines, where virtual avatars can be directly animated from RGB sources and off-the-shelf webcam input. MocapNETs have satisfactory accuracy and state of the art computational performance that, however, prior to this work was not sufficient for their deployment on embedded devices. In this paper we explore dimensionality reduction via the use of Principal Components Analysis (PCA) as a means to optimize their size and make them applicable to mobile and edge devices. PCA allows (a) reduction of input dimensionality, (b) fine-grained control over the variance covered by the maintained dimensions and, (c) drastic reduction of the total number of model/network parameters without compromising regression accuracy. Extensive experiments on the CMU BVH dataset provide insight on the effective receptive fields for densely connected networks. Moreover, PCA-based dimensionality reduction results in a 35% smaller NN compared to the baseline (original NN without any dimension reduction) and derives BVH skeletons without accuracy degradation. As such, the proposed compact NN solution becomes deployable on the Raspberry Pi 4 ARM CPU @ 23Hz.
更多查看译文
关键词
3D Human Pose Estimation,Mobile Devices,VR,Neural Networks,Dimensionality Reduction,MocapNET
AI 理解论文
溯源树
样例
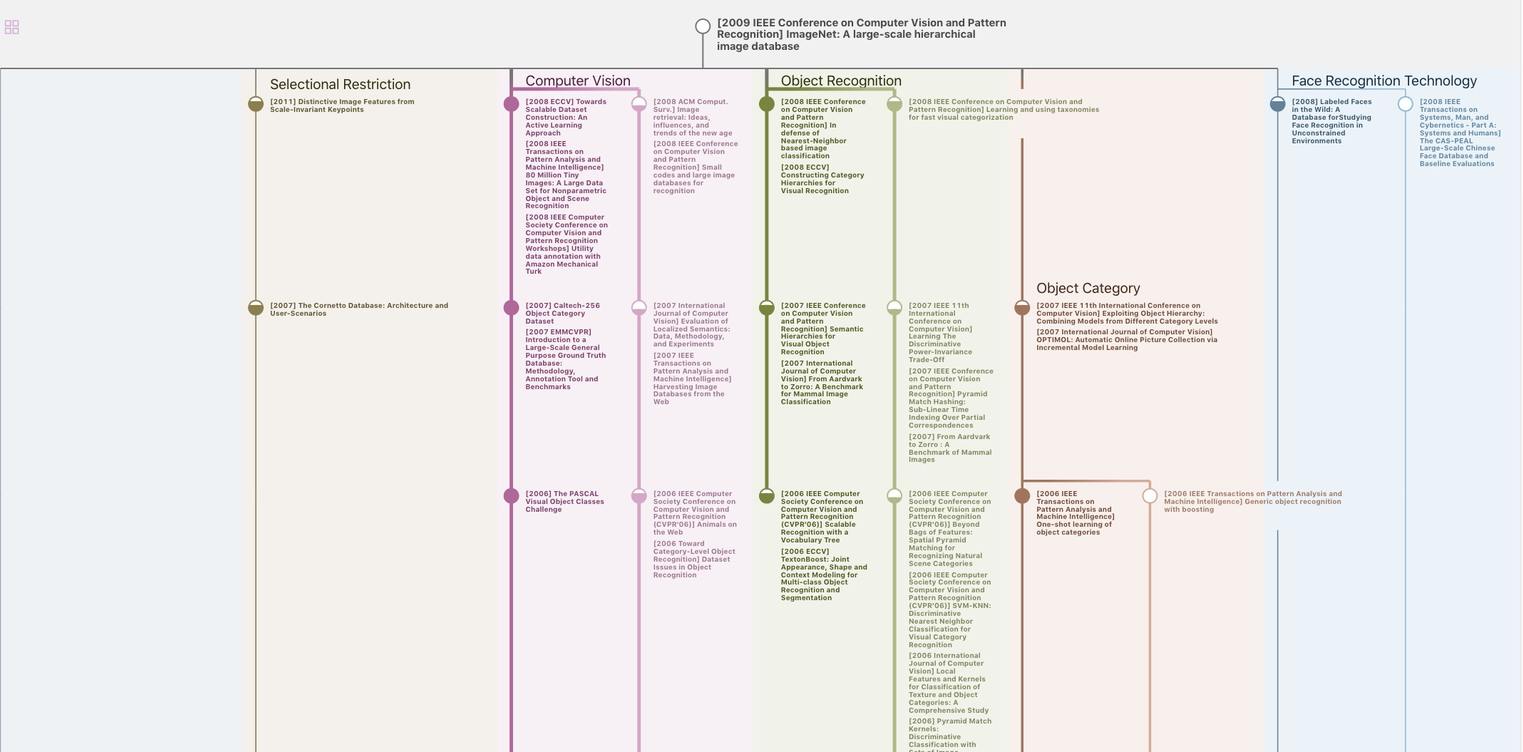
生成溯源树,研究论文发展脉络
Chat Paper
正在生成论文摘要