Accounting for Imputation Uncertainty During Neural Network Training.
DaWaK(2023)
摘要
In this paper we are interested in dealing with missing values in a machine learning context, and more especially when training a neural network. We focus on improving neural network training by reducing the potential biases that can occur during the training phase on artificially imputed datasets. We do so by taking into account the between-variance that can be observed between multiple imputations. We propose two new imputation frameworks, S-HOT and M-HOT , that can be used to train neural networks on completed data in a less biased way, leading to models able of more generalization, and so, to better inference results. We perform extensive comparative experiments and statistically assess the results on both benchmark and real-world datasets. We show that our frameworks compete against and even outperform existing imputation frameworks, while being both useful in different settings. We make our entire code publicly accessible to facilitate reproduction of our experimental results.
更多查看译文
关键词
imputation uncertainty,neural network training
AI 理解论文
溯源树
样例
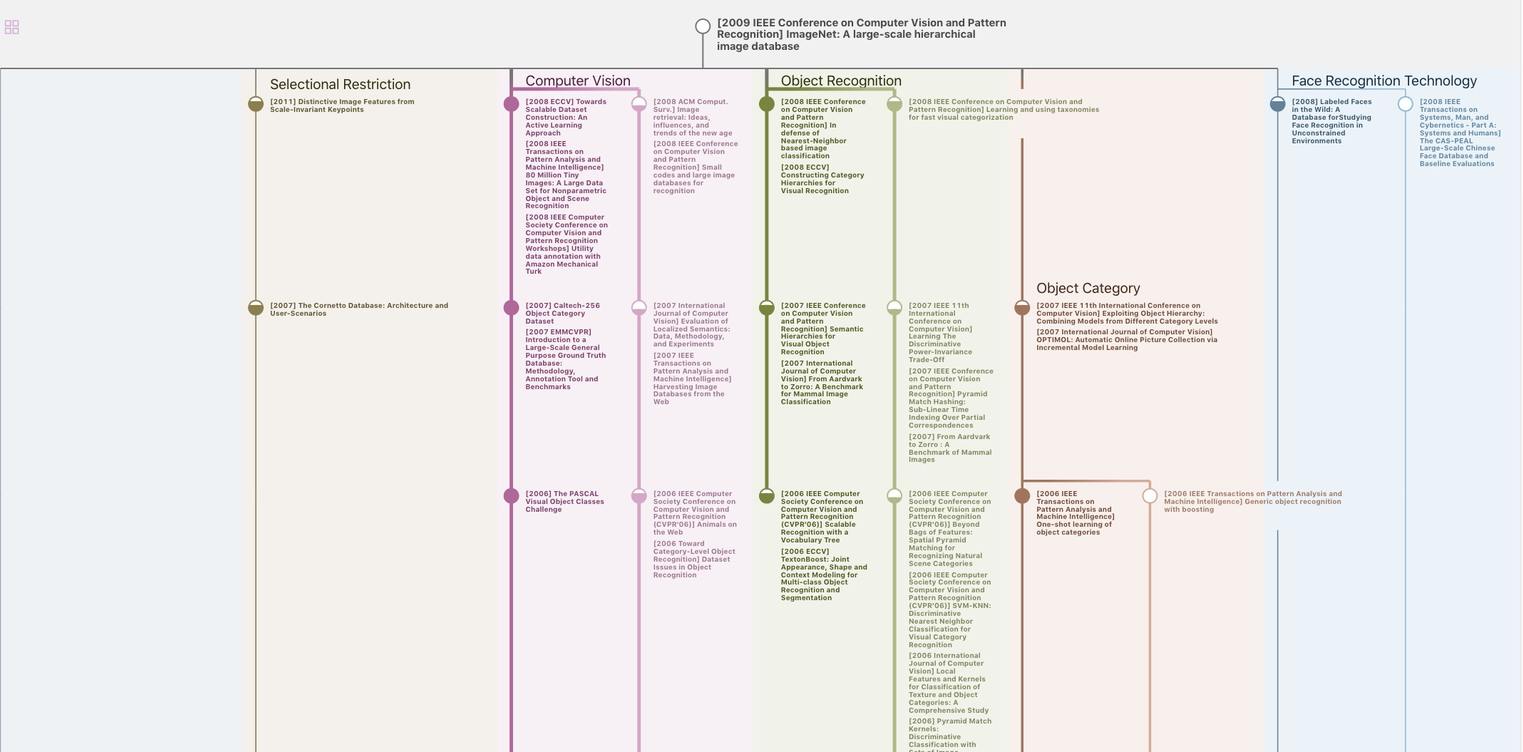
生成溯源树,研究论文发展脉络
Chat Paper
正在生成论文摘要