A Deep Learning-Based Spectral Efficiency Maximization in Multiple Users Multiple STAR-RISs Massive MIMO-NOMA Networks.
ICUFN(2023)
摘要
In this paper, we investigate a deep learning-based spectral efficiency maximization in multiple users multiple simultaneously transmitting and reflecting reconfigurable intelligent surfaces (STAR-RISs) massive multiple-input multiple-output (MIMO)-non-orthogonal multiple access (NOMA) networks, where multiple STAR-RISs are deployed to assist a base station (BS) in communicating with multiple near and far users simultaneously. To improve spectral efficiency (SE), we formulate a joint optimization of the precoding matrix, the phase shift of reflection and transmission of the STAR-RIS problem subject to the individual quality of services (QoS) of each user, maximum power budget at BS and the discrete phase shift. The formulated problem belongs to the class of mixed-integer non-convex optimization problem, which is difficult to solve optimally. We first relax the formulated problem and then transform it into an equivalent non-convex one but with a more tractable form. We then apply the inner approximation method to approximate the non-convex parts iteratively. Towards real-time optimization, we propose a novel deep learning framework with a convolutional neural network (CNN) model to predict the downlink SE based on users’ position information and channel gains. Numerical results show that the proposed approach improves the SE compared to the massive MIMO systems with conventional RIS. Besides, the DL framework for the proposed scheme predicts the optimal SE within a short time compared to the conventional approach.
更多查看译文
关键词
Deep convolutional neural networks,inner approximation,massive MIMO,NOMA,non-convex optimization,phase shift,spectral efficiency,STAR-RIS
AI 理解论文
溯源树
样例
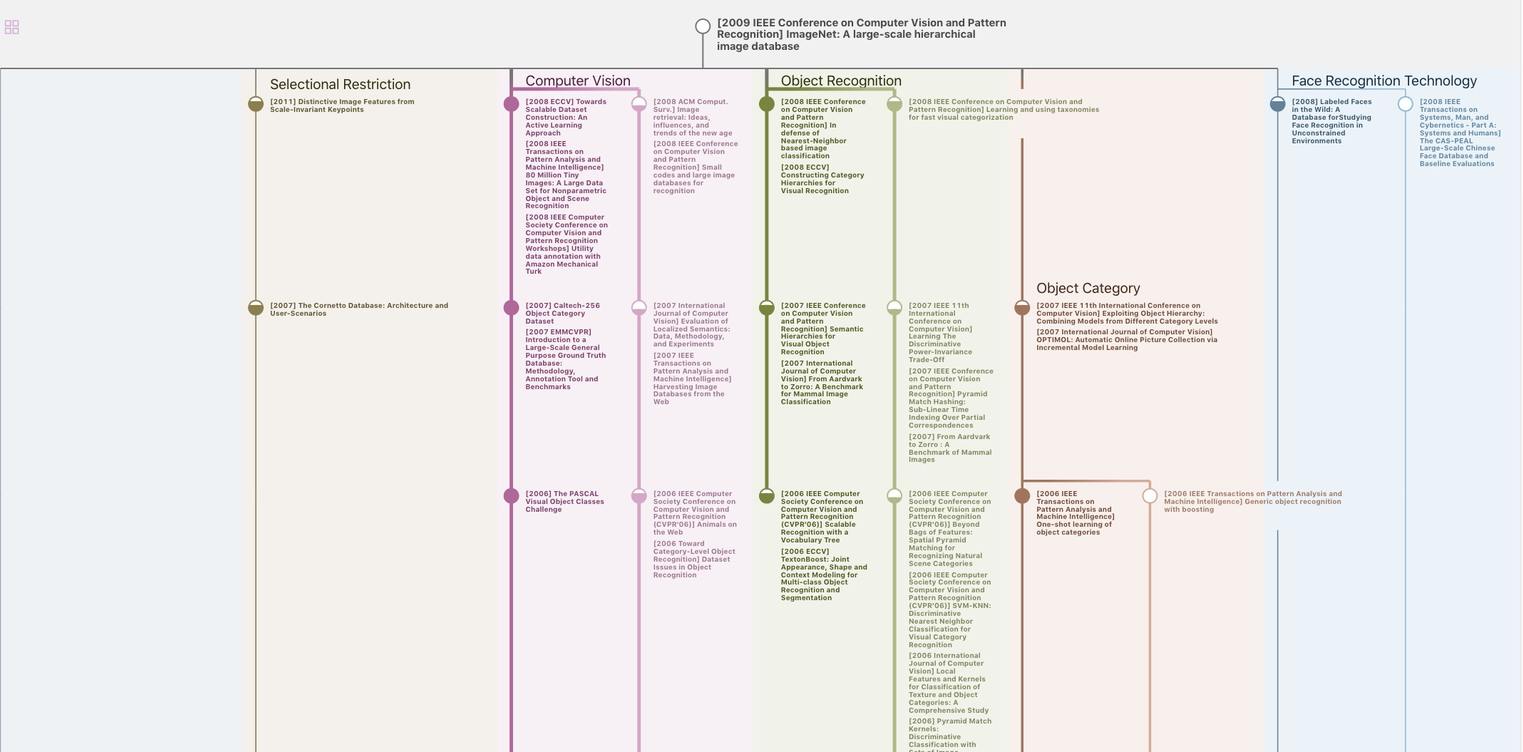
生成溯源树,研究论文发展脉络
Chat Paper
正在生成论文摘要