Pipeline-based Optimization Method for Large-Scale End-to-End Inference.
AI2A(2023)
摘要
Enhancing the utilization of computing resources is a crucial technical challenge within the realm of deep learning model deployment and application. It holds significant importance in effectively leveraging various deep learning models. However, when it comes to actual deployment and operation, deep learning models face an urgent task—processing large-scale data. This processing flow is an end-to-end procedure that typically involves three essential steps: preprocessing, model inference, and postprocessing. Presently, existing research mainly focuses on the optimization of deep learning model algorithms, and rarely considers the coordinated utilization of CPU and accelerator resources after model deployment, resulting in low resource utilization and execution efficiency. In order to solve this problem, in this study, we comprehensively analyzed the demand for computing resources and the mutual adaptation relationship between the end-to-end processing flow in the model application and designed a general algorithm based on the pipeline idea to Realize the overlapping of CPU processing and accelerator operation process. Through this scheme, the serial execution flow of the end-to-end processing can be performed in parallel, resulting in a significant reduction in accelerator latency. We extensively conducted experiments on two specific tasks, and the outcomes demonstrated that our proposed method considerably enhances the accelerator’s utilization rate and program execution efficiency. Specifically, the utilization rate of the accelerator surged from 26% to over 97%, while the program’s execution efficiency witnessed a remarkable improvement of 3.41 to 5.54 times.
更多查看译文
AI 理解论文
溯源树
样例
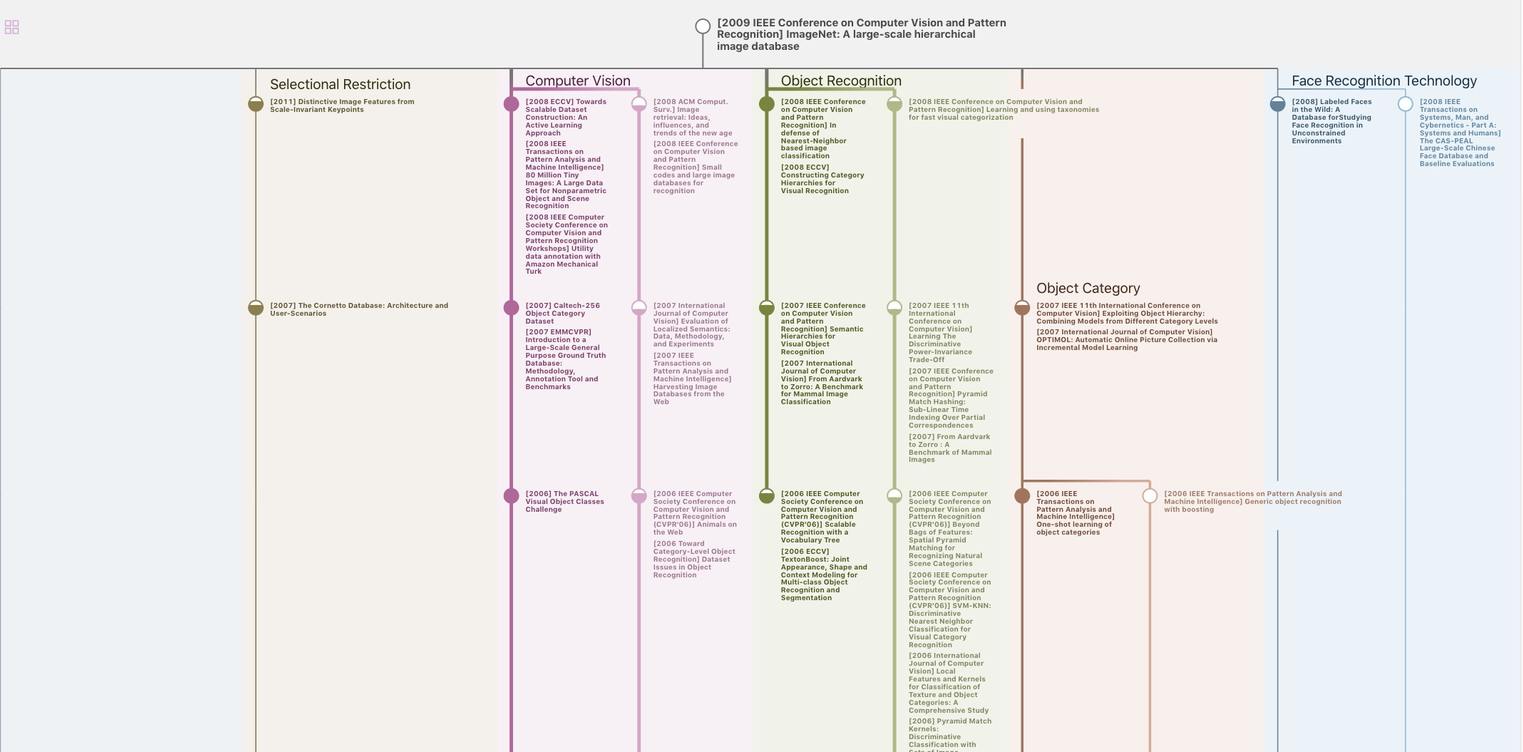
生成溯源树,研究论文发展脉络
Chat Paper
正在生成论文摘要