Split Learning Assisted Multi-UAV System for Image Classification Task
VTC2023-Spring(2023)
摘要
Due to its ease of deployment and high mobility, unmanned aerial vehicles (UAVs) have gained great popularity for a variety of applications. To conduct high-level and complicated tasks such as search/rescue missions and target identification, deep learning functions at UAVs are required. To this end, distributed learning methods such as federated learning (FL) and split learning (SL) have been proposed. In this paper, we investigate the SL assisted image classification task in a multi-UAV system for applications such as area exploration and object detection. Specifically, the whole deep learning model is cut into the UAV-side model and BS (base station)-side model. Each UAV performs forward propagation on UAV-side model by using the locally gathered images, and sends the smashed data to the BS. The BS performs forward and backward propagation based on the smashed data, and sends back the gradients of the cut layer to the UAVs, which is used for the backward propagation of the UAV-side model. The performance was evaluated using an aerial perspective geographic dataset, and the effectiveness of the proposed system was validated by comparing with FL-based and centralized learning methods. It was found that SL can significantly reduce computation time at UAV compared with FL, and is particularly effective with non-IID (independent and identically distributed) dataset. SL also requires less data during the training initial phase and has a faster convergence speed compared to centralized learning.
更多查看译文
关键词
split learning,federated learning,Unmanned aerial vehicles,convolutional neural network,image classification
AI 理解论文
溯源树
样例
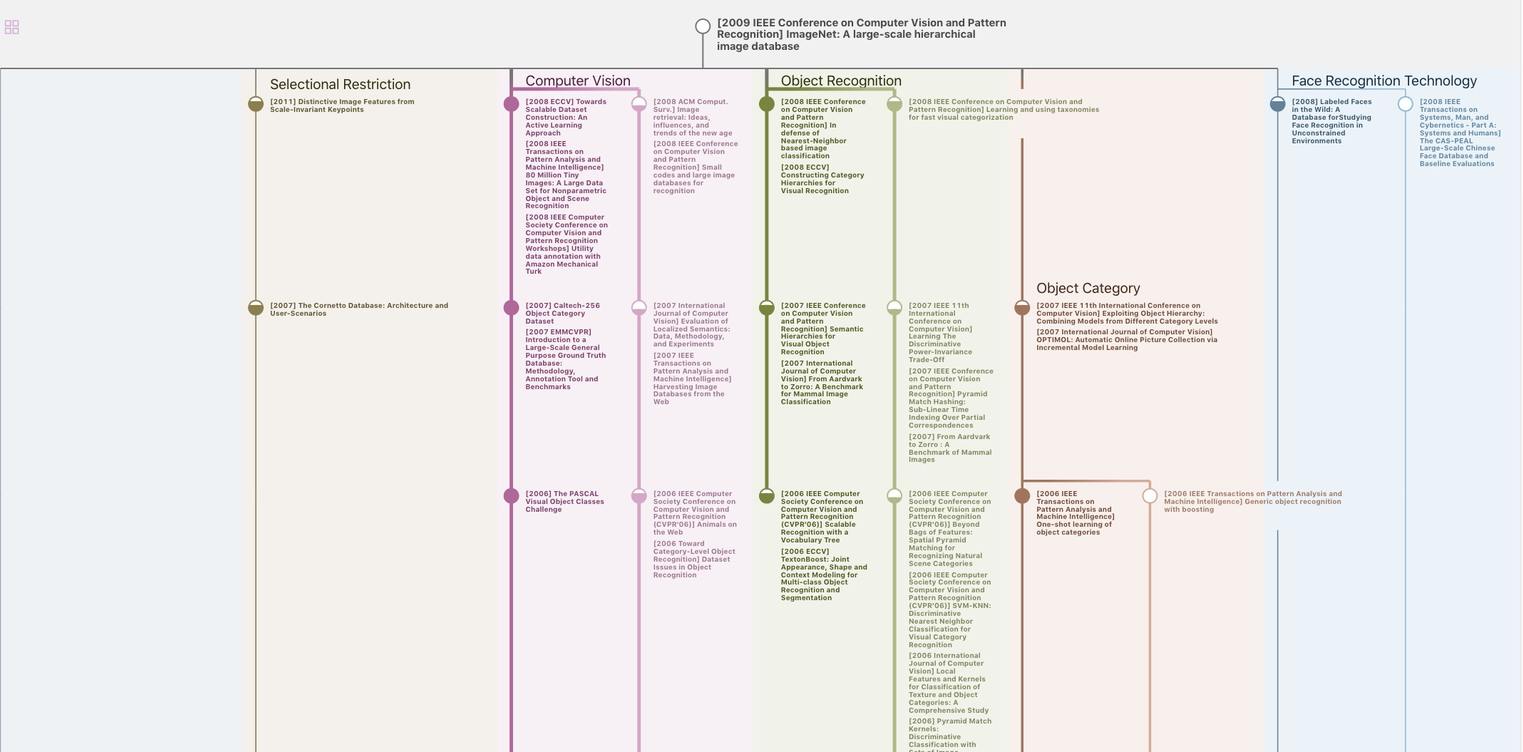
生成溯源树,研究论文发展脉络
Chat Paper
正在生成论文摘要