Federated Learning with Unsourced Random Access
VTC2023-Spring(2023)
摘要
A large number of new applications are emerging in the future sixth-generation (6G) communication systems. Federated learning (FL) enables massive user equipments (UEs), such as mobile phones and Internet of Things (IoT) devices, to cooperatively learn a shared model for prediction in various applications, while keeping the training data local. However, in practical scenarios, there are still some problems in deploying FL systems, including serving a large number of active UEs, longtime delay, and the risk of UEs' privacy leakage. To tackle these issues, we introduce unsourced random access (URA) into the FL systems. URA can support massive connectivity and its unsourced property can protect the UEs' identity privacy. Moreover, considering the trade-off between communication and computation performance and the various importance of different UEs' local models in training epochs, two importance metrics are designed. The UEs can decide their own active probability according to the metrics among the communication rounds, which avoids the additional cost of being scheduled by the base station (BS) and maximums the use of the limited communication resources to ensure UEs with higher priority can upload trained models, thus improving the training efficiency. Simulation results verify the remarkable communication and computation performance of the proposed schemes.
更多查看译文
关键词
Unsourced random access (URA),federated learning (FL),importance sampling
AI 理解论文
溯源树
样例
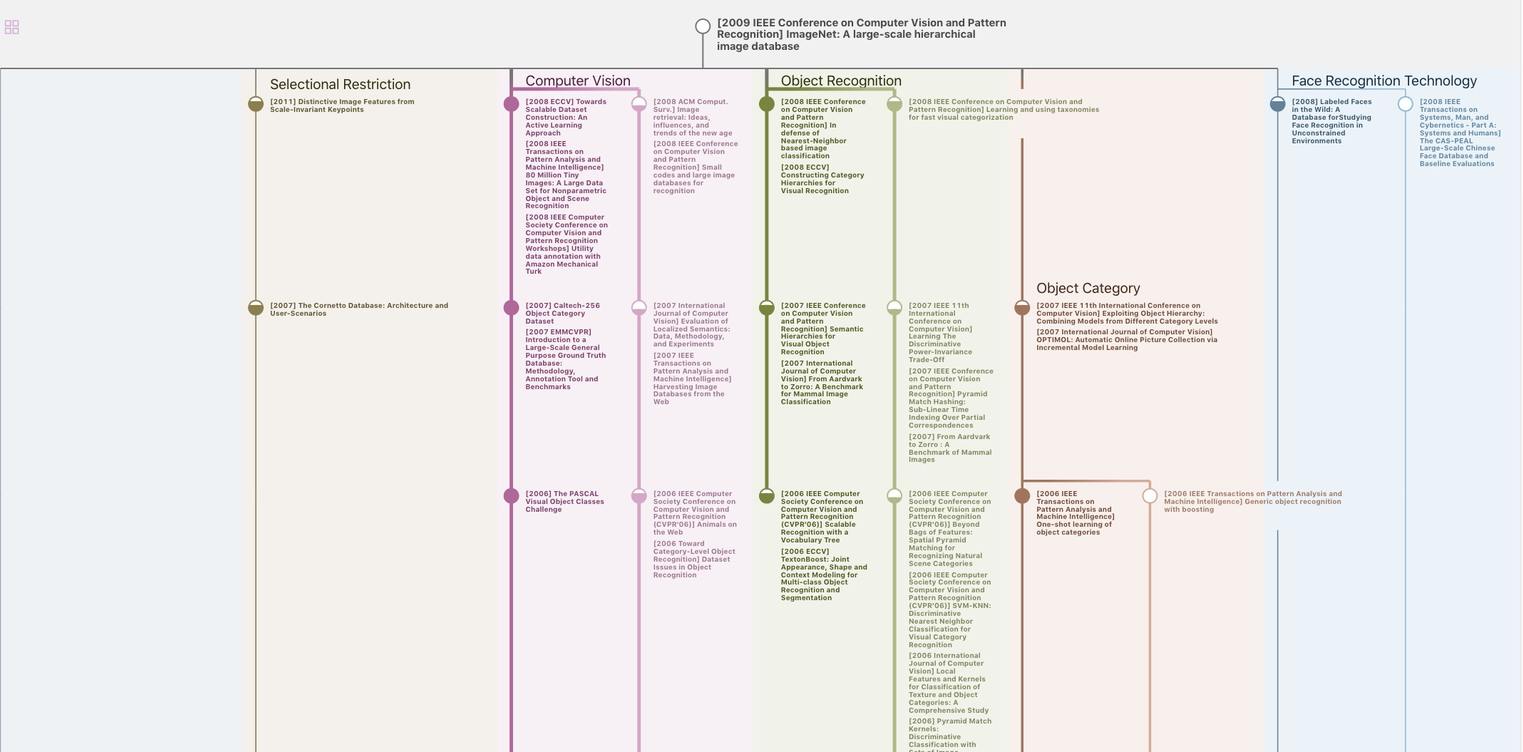
生成溯源树,研究论文发展脉络
Chat Paper
正在生成论文摘要