NASEI: Neural Architecture Search-Based Specific Emitter Identification Method.
VTC2023-Spring(2023)
摘要
Specific emitter identification (SEI) extracts the fingerprint characteristics of emitters according to the subtle differences of transmitted signals, to distinguish different emitter individuals and prevent unauthorized network access. Deep learning (DL) based SEI methods have been proposed to achieve a good identification performance in recent years. However, these methods highly rely on expert experience to design network structures. These hand-designed fixed network structures lack flexibility, which often leads to insufficient model generalization. Neural architecture search (NAS) can be seen as a subdomain of automatic machine learning (AutoML), which can automatically adjust network structure and parameters according to a specific task. In this paper, we propose a neural architecture search-based SEI method, which can achieve an efficient search of the architecture with the use of a gradient descent algorithm. Experimental results show that the proposed NASEI method both improves the accuracy and reduces the parameter quantity when compared with state-of-the-art methods. Code available at https://github.com/huangyuxuan11/NASEI.git.
更多查看译文
关键词
Specific emitter identification,automatic machine learning,neural architecture search,deep learning
AI 理解论文
溯源树
样例
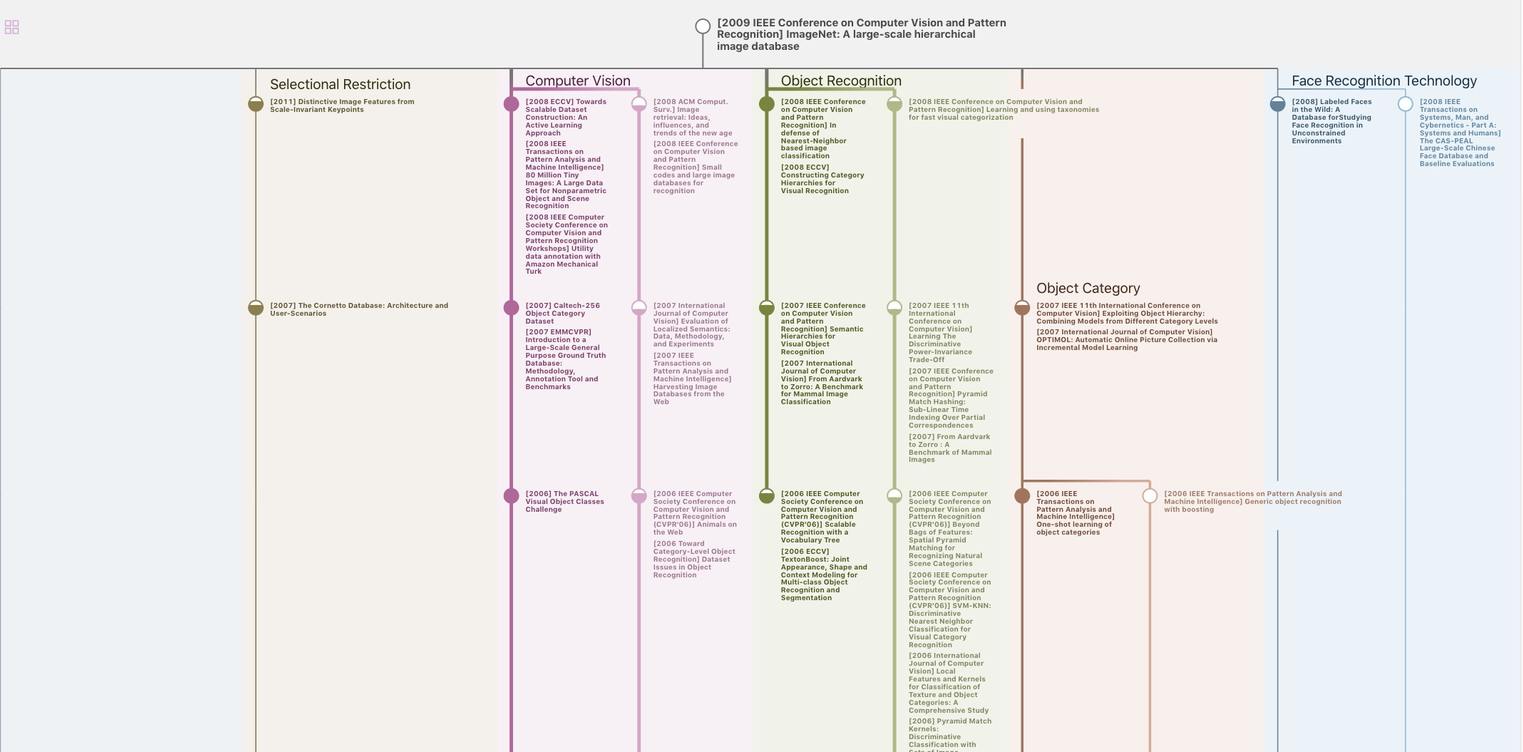
生成溯源树,研究论文发展脉络
Chat Paper
正在生成论文摘要