Random Walking Snakes for Decentralized Learning at Edge Networks.
LANMAN(2023)
摘要
Random walk learning (RWL) has recently gained a lot of attention thanks to its potential for reducing communication and computation over edge networks in a decentralized fashion. In RWL, each node in a graph updates a global model with its local data, selects one of its neighbors randomly, and sends the updated global model. The selected neighbor becomes a newly activated node, so it updates the global model using its local data. This continues until convergence. Despite its promise, RWL has two challenges: (i) training time is long, and (ii) nodes should have the complete model. Thus, in this paper, we design Random Walking Snakes (RWS), where a set of nodes instead of one node is activated for model update, and each node in the set trains a part of the model. Thanks to model partitioning and parallel processing in the set of activated nodes, RWS reduces both the training time and the amount of the model that needs to be stored. We also design a novel policy that determines the set of activated nodes by taking into account the computing power of nodes. Simulation results show that RWS significantly reduces the convergence time as compared to RWL.
更多查看译文
关键词
Decentralized learning, random walk learning, model distributed learning
AI 理解论文
溯源树
样例
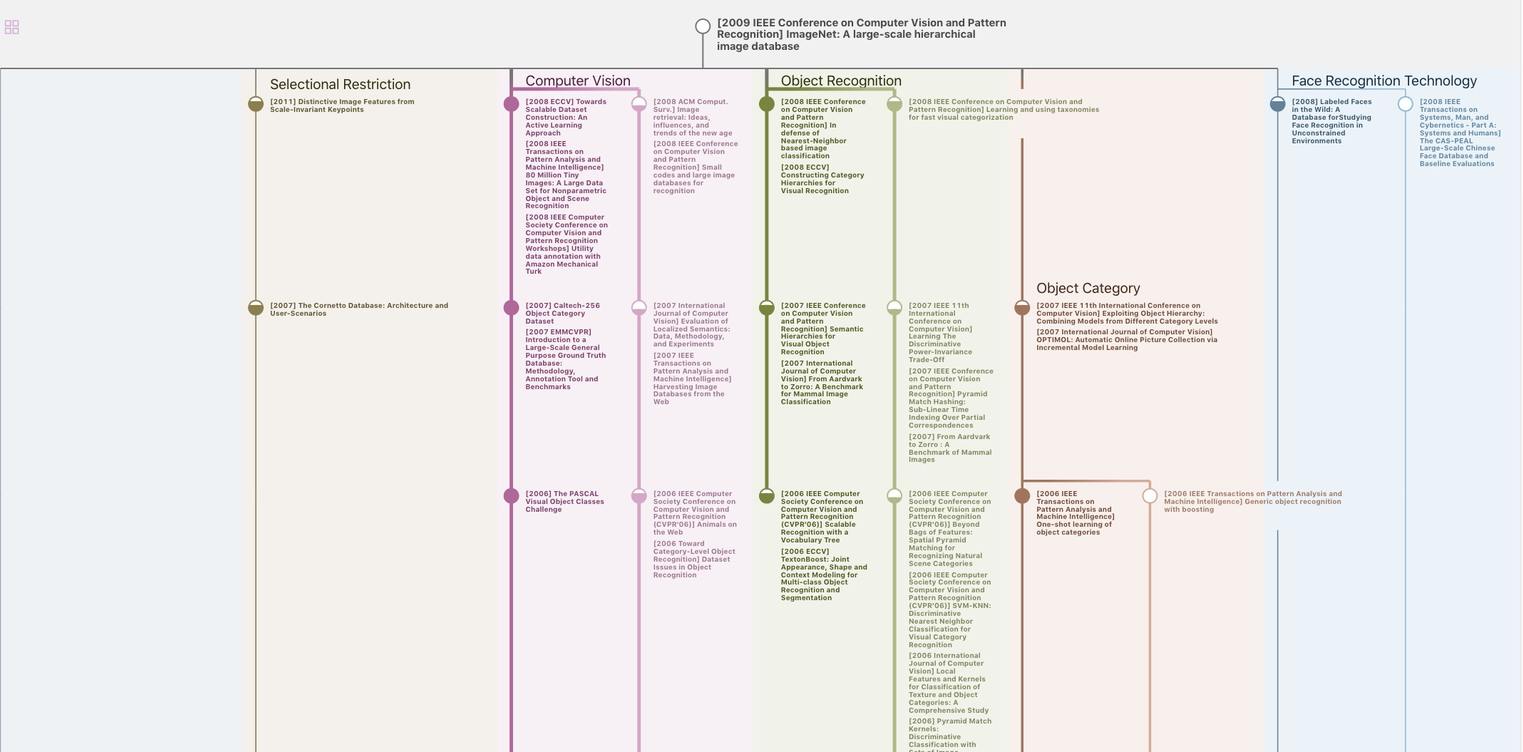
生成溯源树,研究论文发展脉络
Chat Paper
正在生成论文摘要