On the Application of Q-learning for Mobility Load Balancing in Realistic Vehicular Scenarios.
VTC2023-Spring(2023)
摘要
One of the applications where fifth generation (5G) networks are expected to have a greatest impact is vehicular-to-everything (V2X) communications. The exchange of data among different vehicles on the network will make roads a safer environment. However, the massive usage of V2X communications leads to an increase of data traffic and resources consumption. Moreover, the mobility associated to vehicles may drain the available resources in particular cells, compromising the required quality of service (QoS) of connected users. To avoid these situations, the introduction of machine learning algorithms to perform mobility load balancing between neighboring cells arises as a promising tool to ensure to all connected vehicles their demanded resources. In this paper, we address the cell overload problem by proposing an O-RAN compliant Q-learning algorithm that dynamically adapts the handover offset between two neighboring cells to mitigate a network overload situation. The algorithm performance is assessed using realistic vehicular traces. Results show that the network overload appearing during rush hour can be successfully mitigated and the load is fairly distributed between cells.
更多查看译文
关键词
Mobility load balancing, V2X, Reinforcement Learning
AI 理解论文
溯源树
样例
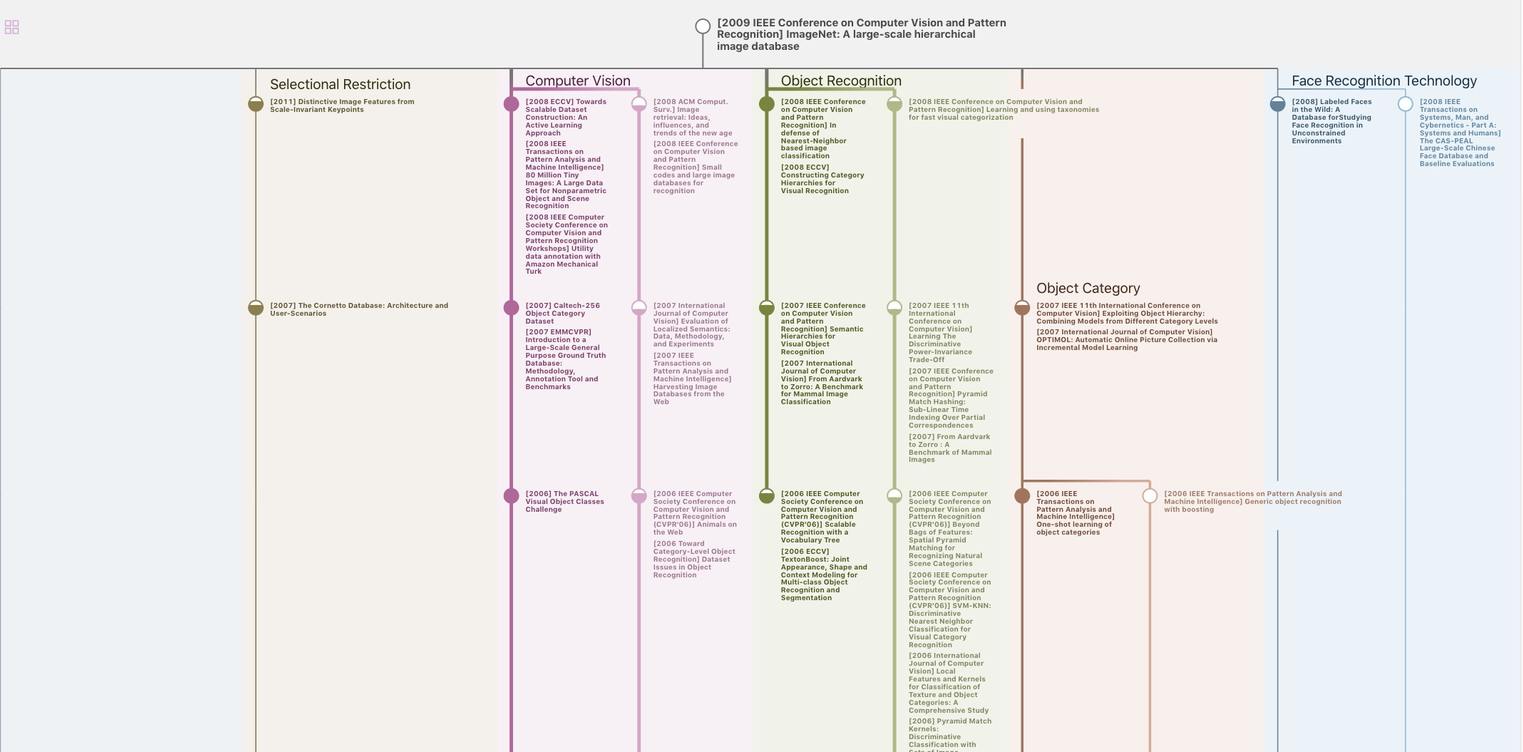
生成溯源树,研究论文发展脉络
Chat Paper
正在生成论文摘要