Resource Allocation Mechanism of Federated Learning System for Highly Dynamic and Changeable Businesses.
BMSB(2023)
摘要
As an emerging distributed machine learning architecture, federated learning (FL) allows multiple vehicle terminals in the Internet-of-Vehicles (IoV) to conduct local model training and achieve global aggregation of models under the condition of data privacy protection, thus providing reliable IoV service. However, in the process of FL training, due to the variability of the business requirements of vehicle terminals in the IoV, the terminal model within the coverage of each edge node changes dynamically, resulting in low accuracy of the regional model. Therefore, we propose a training node dynamic selection algorithm based on user behavior prediction, which comprehensively considers the movement trajectory, number of terminals, terminal reliability and business type which improving the training accuracy of the regional model. The edge resource allocation model is designed to improve the resource utilization of edge nodes. In the dual-layer model of FL, the edge-terminal collaborative aggregation layer (ETCAL) and cloud-edge collaborative aggregation layer (CECAL) are designed. In the ETCAL, the asynchronous federated learning model aggregation mechanism based on the business intensity model which comprehensively considering the number, the type, the reliability, as well as the arrival rate of business. While, in the CECAL, the weighted asynchronous aggregation mechanism based on raw data volume is adopted, which improves the aggregation efficiency of the FL system. The simulation results show that the proposed mechanism is superior to local, FL-NonUBP and FL-UBP algorithm in terms of model accuracy.
更多查看译文
关键词
Federated learning,Task migration,Highly dynamic,Changeable business,Internet of Vehicles
AI 理解论文
溯源树
样例
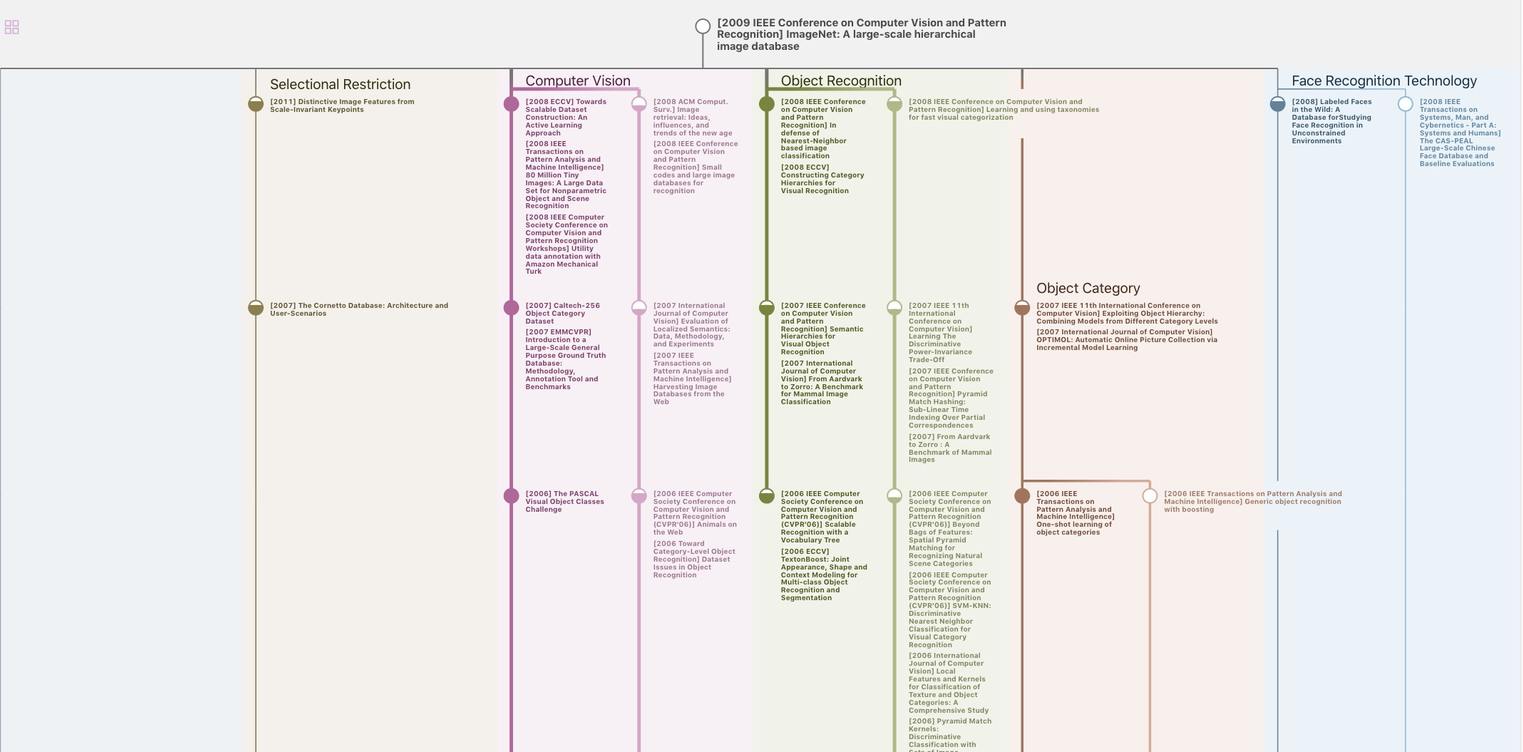
生成溯源树,研究论文发展脉络
Chat Paper
正在生成论文摘要