Enhancing Adversarial Robustness via Anomaly-aware Adversarial Training.
KSEM (1)(2023)
Abstract
Adversarial training (AT) is one of the most promising solutions for defending adversarial attacks. By exploiting the adversarial examples generated in the maximization step of AT, a large improvement on the robustness can be brought. However, by analyzing the original natural examples and the corresponding adversarial examples, we observe that a certain part of them are abnormal. In this paper, we propose a novel AT framework called anomaly-aware adversarial training (A $$^3$$ T), which utilizes different learning strategies for handling the one normal case and two abnormal cases of generating adversarial examples. Extensive experiments on three publicly available datasets with classifiers in three major network architectures demonstrate that A $$^3$$ T is effective in robustifying networks to adversarial attacks in both white/black-box settings and outperforms the state-of-the-art AT methods.
MoreTranslated text
Key words
adversarial robustness,anomaly-aware
AI Read Science
Must-Reading Tree
Example
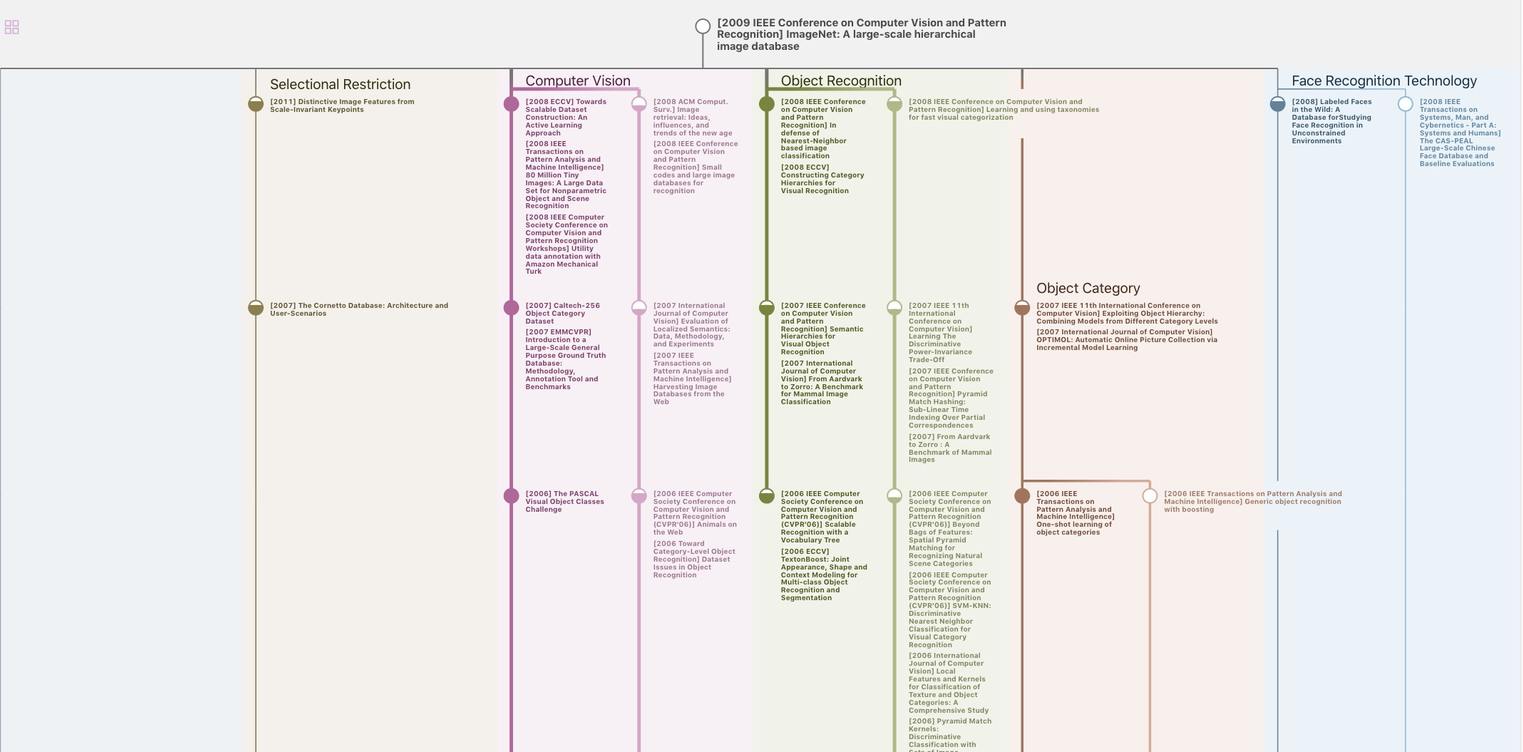
Generate MRT to find the research sequence of this paper
Chat Paper
Summary is being generated by the instructions you defined