Style Augmentation and Domain-Aware Parametric Contrastive Learning for Domain Generalization.
KSEM (4)(2023)
摘要
The distribution shift between training data and test data degrades the performance of deep neural networks (DNNs), and domain generalization (DG) alleviates this problem by extracting domain-invariant features explicitly or implicitly. With limited source domains for training, existing approaches often generate samples of new domains. However, most of these approaches confront the issue of losing class-discriminative information. To this end, we propose a novel domain generalization framework containing style augmentation and Domain-aware Parametric Contrastive Learning (DPCL). Specifically, features are first decomposed into high-frequency and low-frequency components, which contain shape and style information, respectively. Since the shape cues contain class information, the high-frequency components remain unchanged. Then Exact Feature Distribution Mixing (EFDMix) is used for diversifying the low-frequency components, which fully uses each order statistic of the features. Finally, both components are re-merged to generate new features. Additionally, DPCL is proposed, based on supervised contrastive learning, to enhance domain invariance by ignoring negative samples from different domains and introducing a set of parameterized class-learnable centers. The effectiveness of the proposed style augmentation method and DPCL is confirmed by experiments. On the PACS dataset, our method improves the state-of-art average accuracy by 1.74% using ResNet-50 backbone and even achieves excellent performance in the single-source DG task.
更多查看译文
关键词
generalization,learning,style,domain-aware
AI 理解论文
溯源树
样例
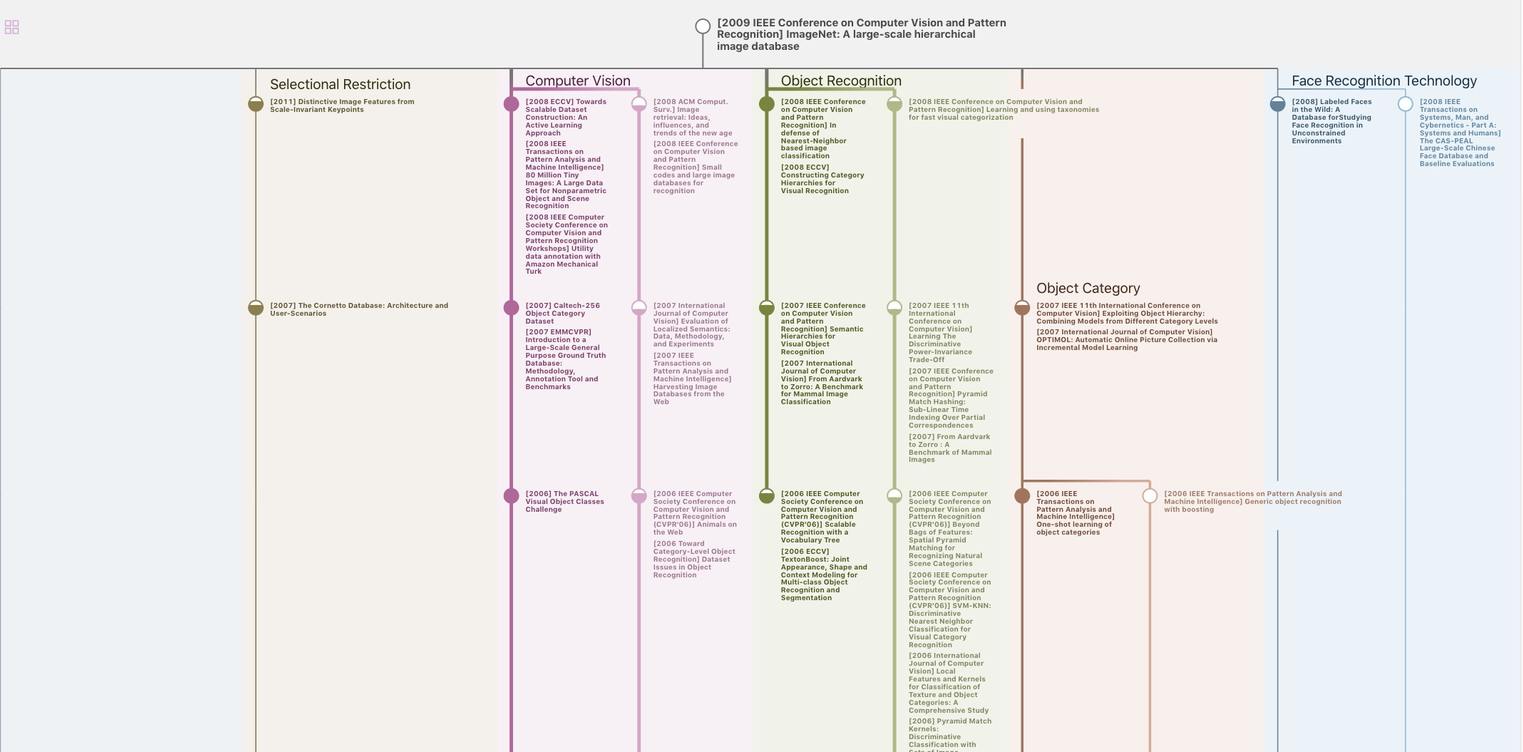
生成溯源树,研究论文发展脉络
Chat Paper
正在生成论文摘要