A Graph Neural Network for Cross-domain Recommendation Based on Transfer and Inter-domain Contrastive Learning.
KSEM (3)(2023)
摘要
Cross-domain recommendation (CDR) is an effective method to deal with the problem of data sparsity in recommender systems. However, most of the existing CDR methods belong to single-target CDR, which only improve the recommendation effect of the target domain without considering the effect of the source domain. Meanwhile, the existing dual-target or multi-target CDR methods do not consider the differences between domains during the feature transfer. To address these problems, this paper proposes a graph neural network for CDR based on transfer and inter-domain contrastive learning (TCLCDR). Firstly, user-item graphs of two domains are constructed, and data from both domains are used to alleviate the problem of data sparsity. Secondly, a graph convolutional transfer layer is introduced to make the information of the two domains transfer bidirectionally and alleviate the problem of negative transfer. Finally, contrastive learning is performed on the overlapping users or items in the two domains, and the self-supervised contrastive learning task and supervised learning task are jointly trained to alleviate the differences between the two domain.
更多查看译文
关键词
graph neural network,contrastive learning,transfer,cross-domain,inter-domain
AI 理解论文
溯源树
样例
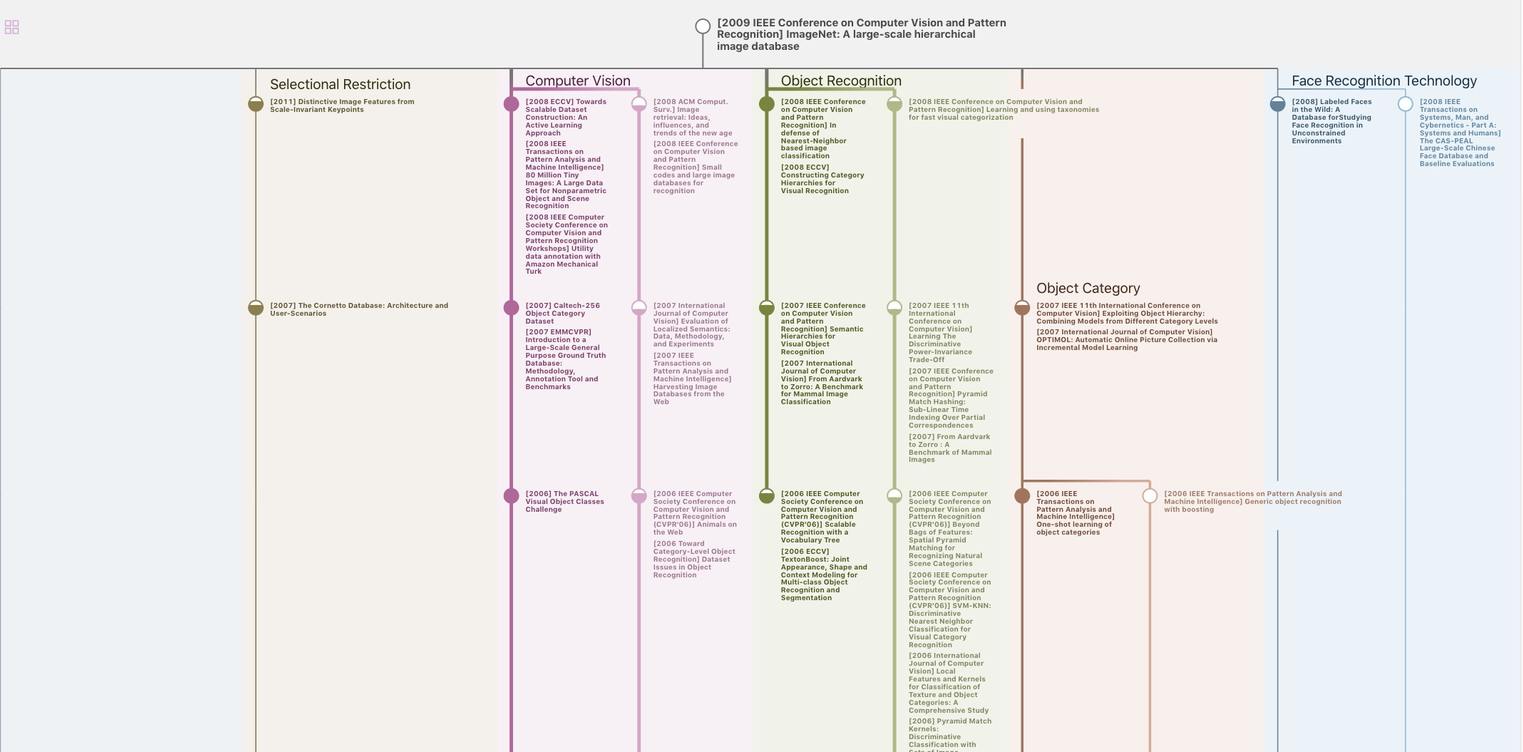
生成溯源树,研究论文发展脉络
Chat Paper
正在生成论文摘要