Age Feature Enhanced Neural Network for RUL Estimation of Power Electronic Devices.
ICPHM(2023)
摘要
Like other deep learning problems, critical features are critical to enable effective estimation of Remaining Useful Lifetime (RUL) for power electronic devices using Neural Networks (NNs). However, these critical features are often indirectly obtained after data pre-processing, complicated either in form (high dimension) or in computation (computation-intensive pre-processing). In the paper, we suggest adding a simple direct feature, age, into the NN based RUL estimation technique. The rationale for incorporating this feature is that the device lifetime is a sum of past time (age) plus RUL. Thus it has a strong correlation to RUL. In our experiments using accelerated aging tests, we show that the new age feature enhanced Recurrent Neural Network (RNN) model can significantly improve estimation accuracy and shorten training convergence time. It also outperforms a state-of-the-art RNN model using derived time-domain statistical features.
更多查看译文
关键词
Terms power electronic device, remaining useful lifetime (RUL), neural network, feature selection, prognostic and health management (PHM), reliability
AI 理解论文
溯源树
样例
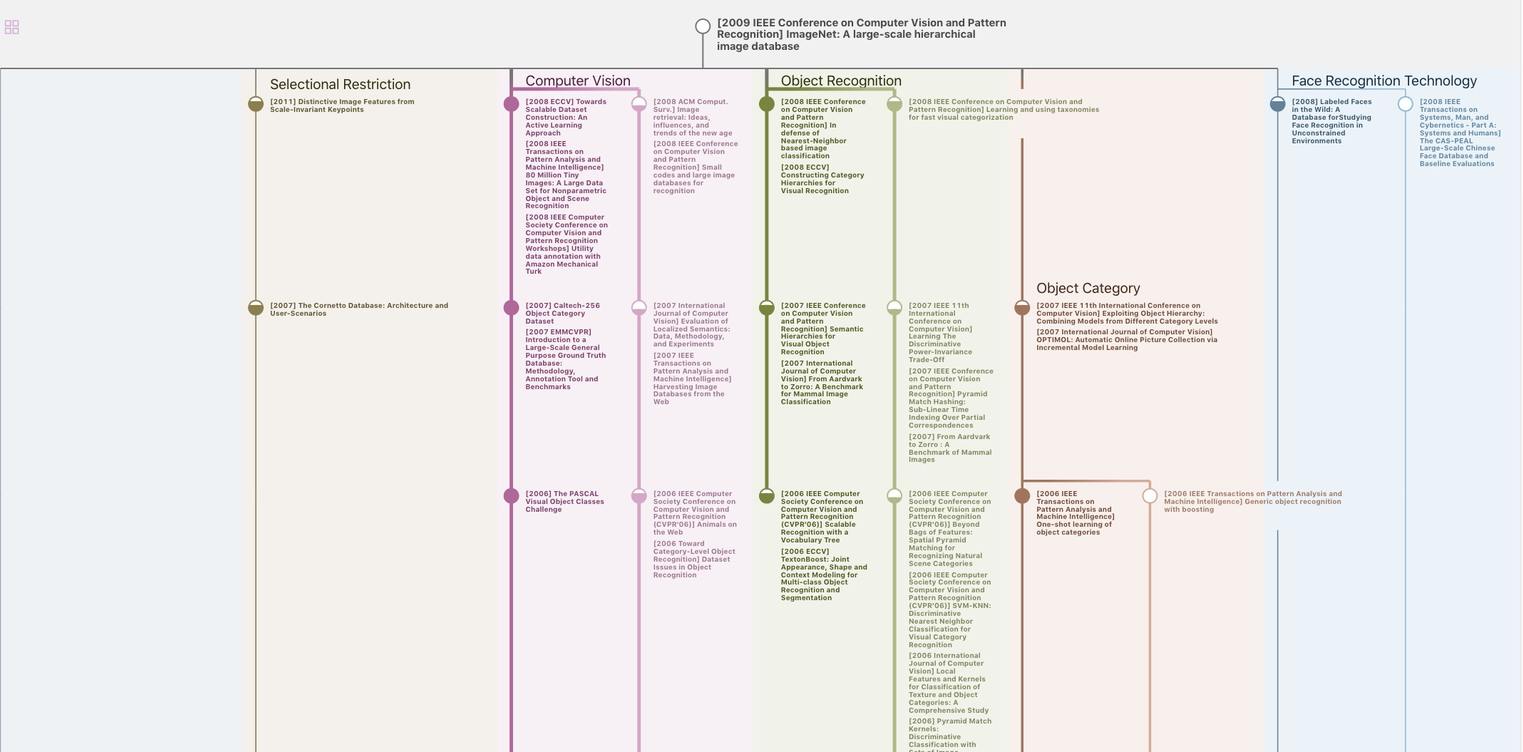
生成溯源树,研究论文发展脉络
Chat Paper
正在生成论文摘要