Prototype-Augmented Contrastive Learning for Few-Shot Unsupervised Domain Adaptation.
KSEM (4)(2023)
Abstract
Unsupervised domain adaptation aims to learn a classification model from the source domain with much-supervised information, which is applied to the utterly unsupervised target domain. However, collecting enough labeled source samples is difficult in some scenarios, decreasing the effectiveness of previous approaches substantially. Therefore, a more challenging and applicable problem called few-shot unsupervised domain adaptation is considered in this work, where a classifier trained with only a few source labels needs to show strong generalization on the target domain. The prototype-based self-supervised learning method has presented superior performance improvements in addressing this problem, while the quality of the prototype could be further improved. To mitigate this situation, a novel Prototype-Augmented Contrastive Learning is proposed. A new computation strategy is utilized to rectify the source prototypes, which are then used to improve the target prototypes. To better learn semantic information and align features, both in-domain prototype contrastive learning and cross-domain prototype contrastive learning are performed. Extensive experiments are conducted on three widely used benchmarks: Office, OfficeHome, and DomainNet, achieving accuracy improvement of over 3%, 1%, and 0.5%, respectively, demonstrating the effectiveness of the proposed method.
MoreTranslated text
Key words
adaptation,learning,domain,prototype-augmented,few-shot
AI Read Science
Must-Reading Tree
Example
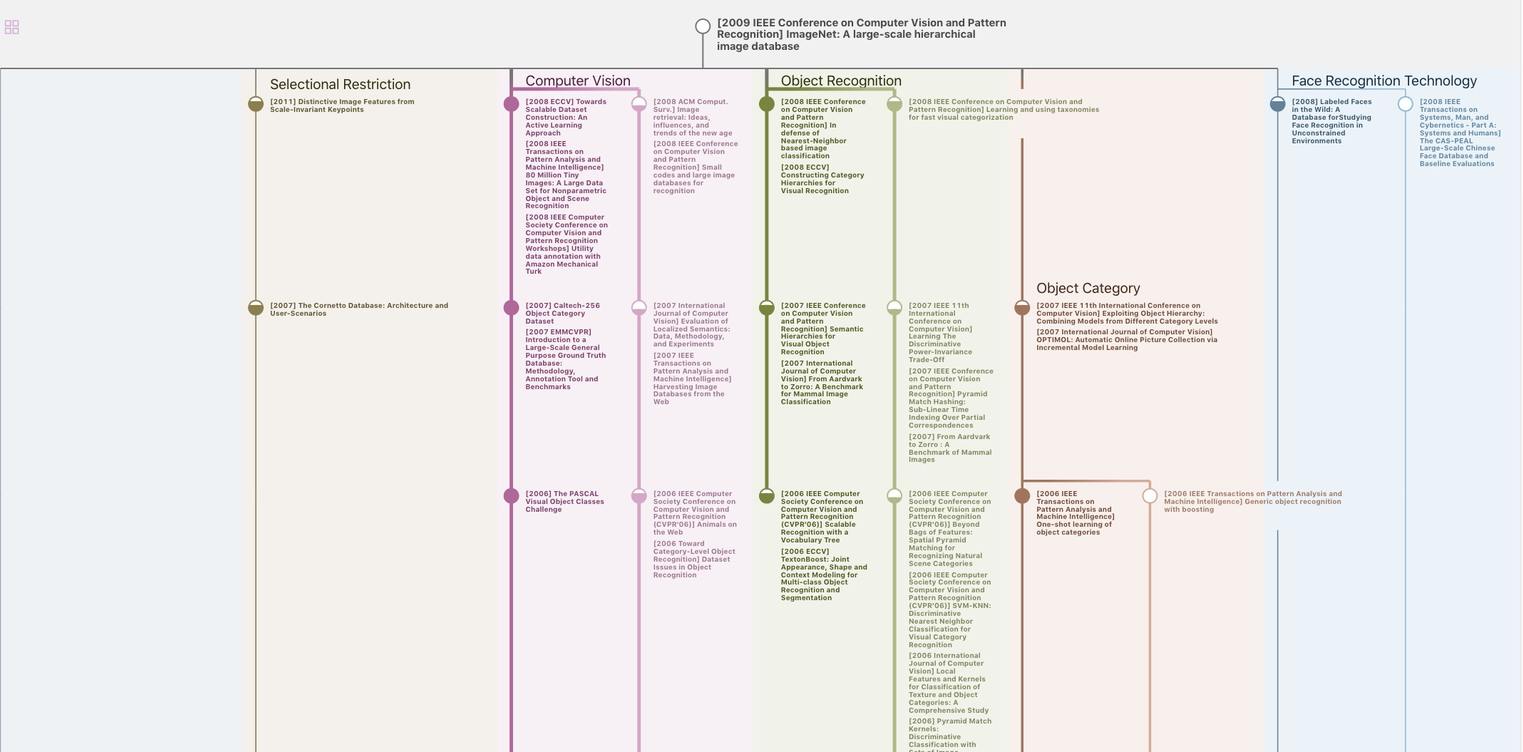
Generate MRT to find the research sequence of this paper
Chat Paper
Summary is being generated by the instructions you defined