Secure Embedding Aggregation for Federated Representation Learning
ISIT(2023)
Abstract
We consider a federated representation learning framework, where with the assistance of a central server, a group of N distributed clients train collaboratively over their private data, for the representations (or embeddings) of a set of entities (e.g., users in a social network). Under this framework, for the key step of aggregating local embeddings trained privately at the clients, we develop a secure embedding aggregation protocol named SecEA, which leverages all potential aggregation opportunities among all the clients, while providing privacy guarantees for the set of local entities and corresponding embeddings simultaneously at each client, against a curious server and up to T < N/2 colluding clients.
MoreTranslated text
Key words
federated representation learning,aggregation
AI Read Science
Must-Reading Tree
Example
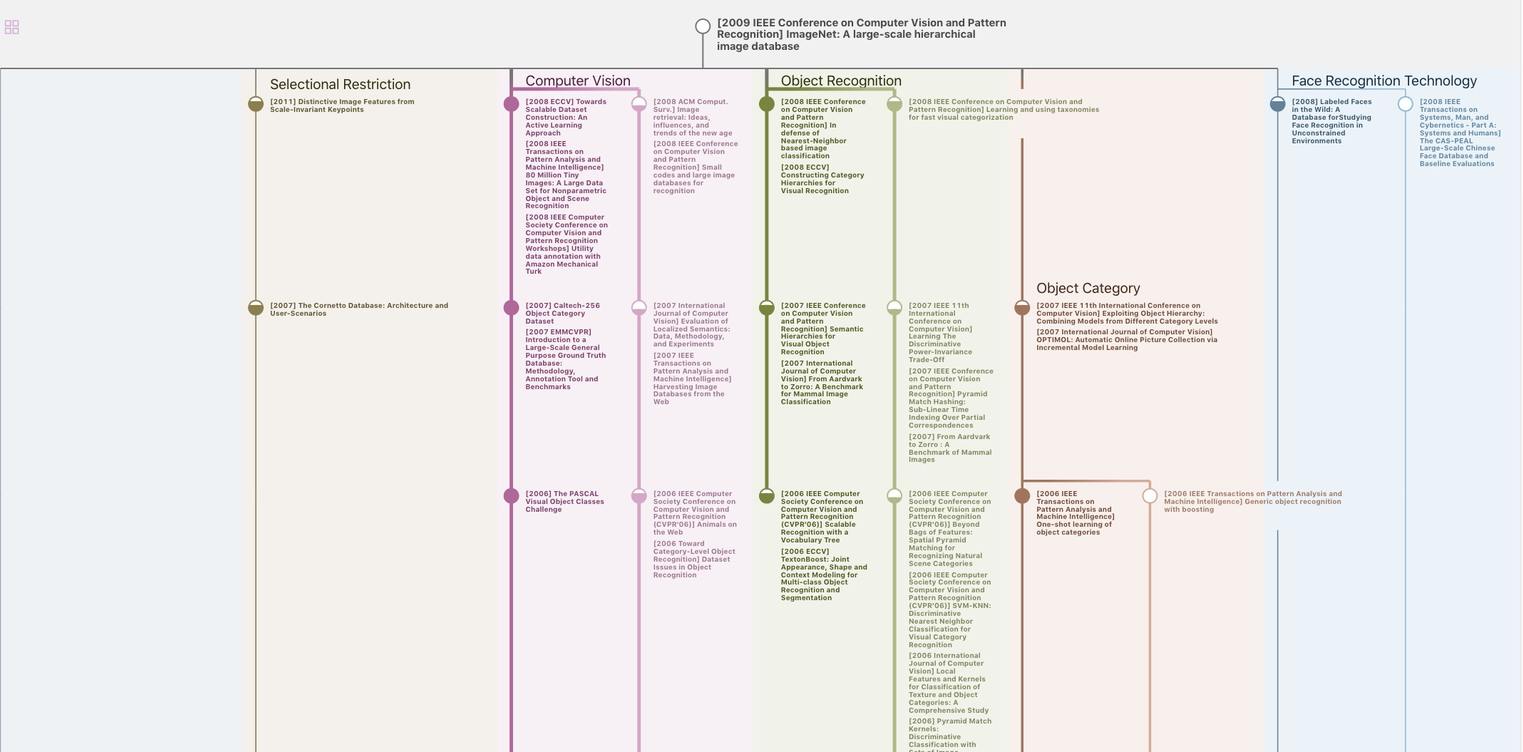
Generate MRT to find the research sequence of this paper
Chat Paper
Summary is being generated by the instructions you defined