Suitability of Forward-Forward and PEPITA Learning to MLCommons-Tiny benchmarks.
COINS(2023)
摘要
On-device learning challenges the restricted memory and computation requirements imposed by its deployment on tiny devices. Current training algorithms are based on backpropagation which requires storing intermediate activations to compute the backward pass and to update the weights into the memory. Recently “Forward-only algorithms” have been proposed as biologically plausible alternatives to backpropagation. At the same time, they remove the need to store the intermediate activations which potentially lower the power consumption due to memory read and write operations, thus, opening to new opportunities for further savings. This paper investigates quantitatively the improvements in terms of complexity and memory usage brought by PEPITA and Forward-Forward computing approaches with respect to backpropagation on the MLCommons-Tiny benchmarks set as case studies. It was observed that the reduction in activations' memory provided by “Forward-only algorithms” does not affect total RAM in Fully-connected networks. On the other hand, Convolutional neural networks benefit the most from such reduction due to lower parameters-activations ratio. In the context of the latter, a memory-efficient version of PEPITA reduces, on average, one third of the total RAM with respect to backpropagation, introducing only a third more complexity. Forward-Forward brings average memory reduction to 40%, and it involves additional computation at inference that, depending on the benchmarks studied, can be costly on micro-controllers.
更多查看译文
关键词
on-device learning,backpropagation,forward learning,PEPITA,tiny devices,MLCommons-tiny
AI 理解论文
溯源树
样例
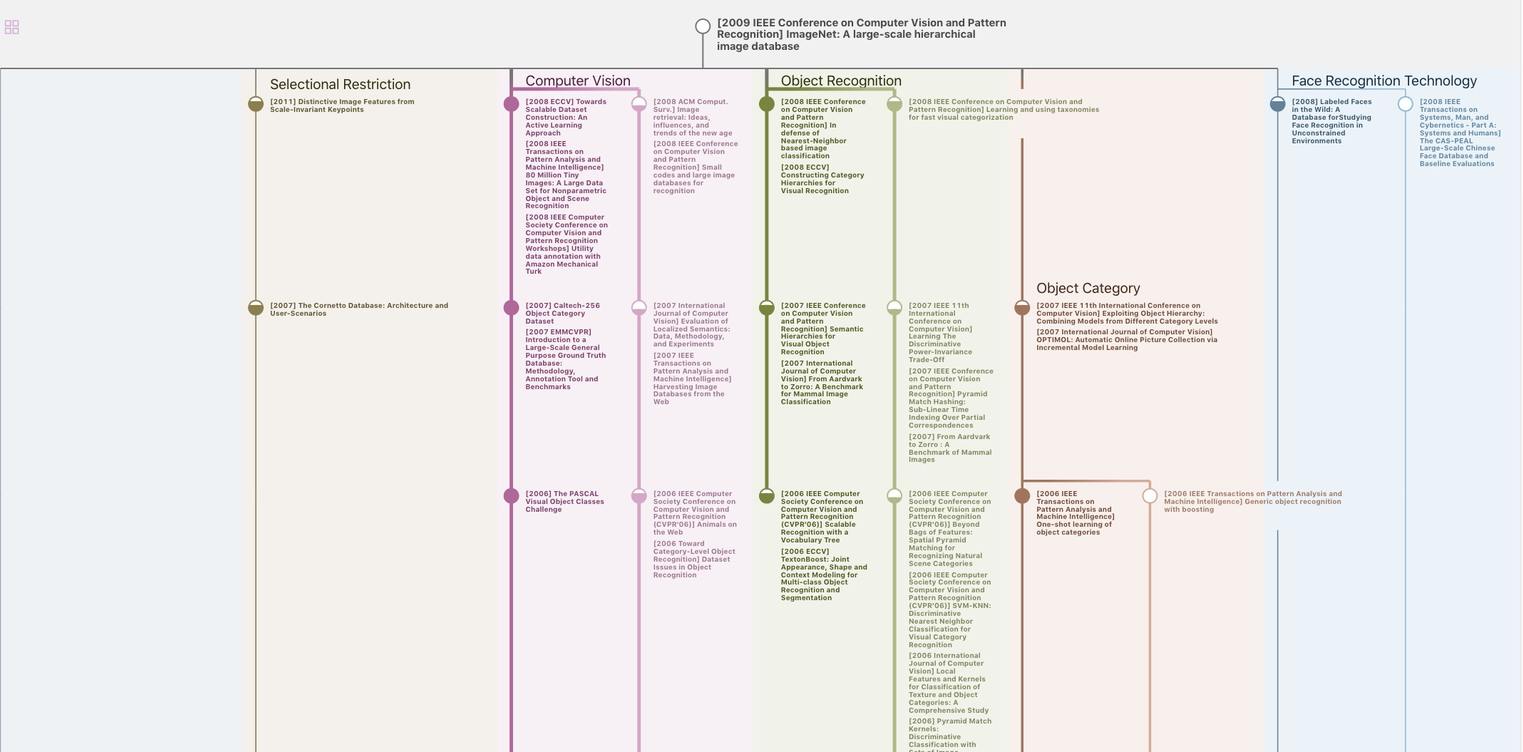
生成溯源树,研究论文发展脉络
Chat Paper
正在生成论文摘要