A Bayesian Interpretation of Fuzzy C-Means.
EUSFLAT/AGOP(2023)
摘要
In Explainable Artificial Intelligence, the interpretation of the decisions provided by a model is of primary importance. In this context, we consider Fuzzy C-Means (FCM), which is a clustering algorithm that induces a model from data by assigning, to each data-point, a degree of membership to each cluster such that the sum of memberships is one. A fuzzification parameter is also used to tune the degree of fuzziness of clusters. The distribution of membership degrees suggests an interpretation of membership degrees within the Probability Theory. This paper shows that the membership degrees resulting from FCM can be interpreted as posterior probabilities derived from a Bayesian model, which assumes that data are generated through a specific probability density function. The results give a clear interpretation of the membership degrees of FCM, as well as its fuzzification parameter, within a sound theoretical framework, and shed light on possible extensions of the algorithm.
更多查看译文
关键词
fuzzy,bayesian interpretation,c-means
AI 理解论文
溯源树
样例
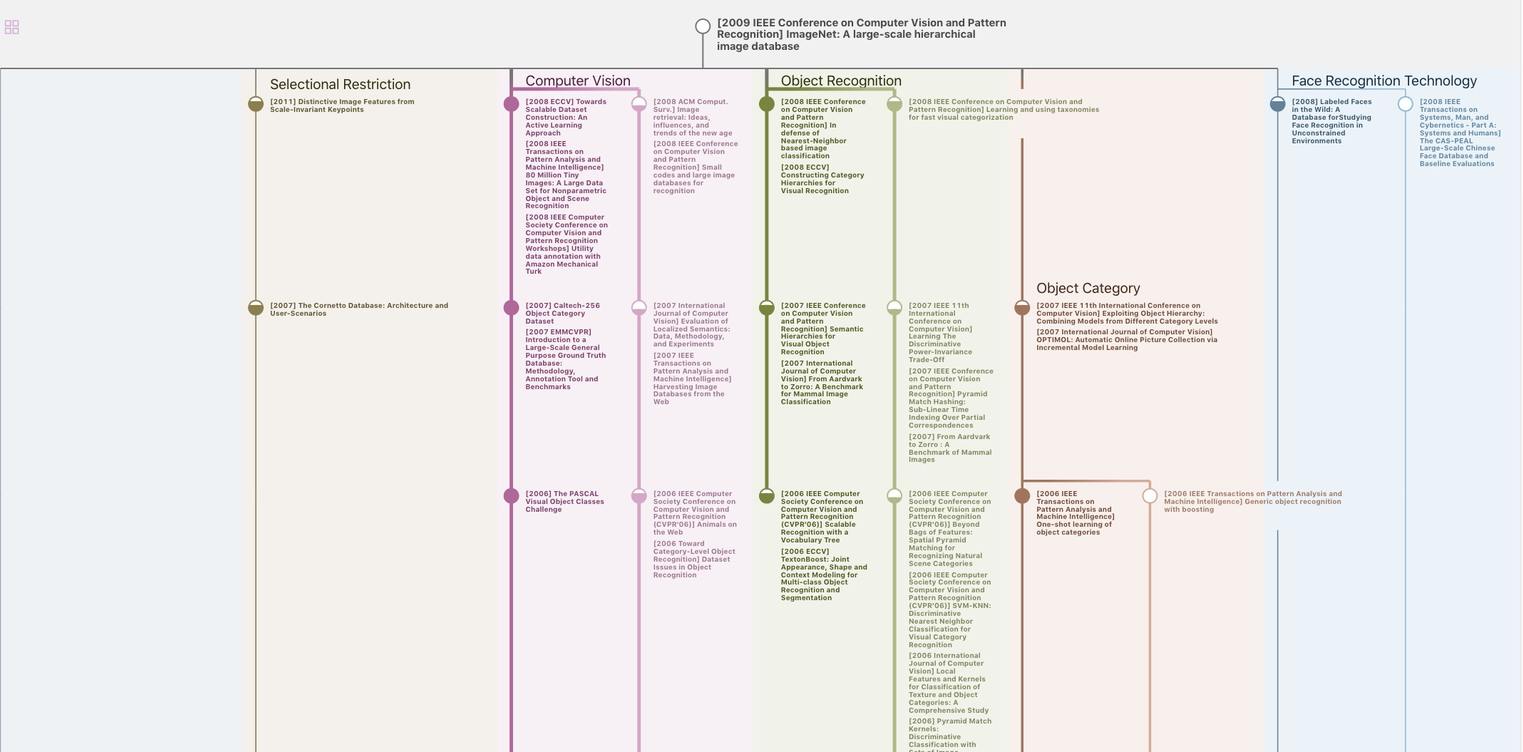
生成溯源树,研究论文发展脉络
Chat Paper
正在生成论文摘要