CasieNet: Category-aware and Side Information Enhanced Pre-training Network for Carbon Emission Prediction.
ICIAI(2023)
摘要
In the background of low carbon power, accurate prediction of carbon emission intensity and carbon emission source percentage of the power system can provide data support for carbon emission optimization strategy to achieve effective reduction of carbon emission of the power system. Most of the current methods adopt statistical methods and power flow analysis, etc. However, these approaches cannot make effective carbon emission prediction for specific generation patterns, and they are not suitable for use in real power systems that are affected by weather, wire state and energy storage element state, etc. under lossy conditions. For that reason, this paper proposes the CasieNet (Category-aware and side information enhanced) pre-training network for carbon emission prediction tasks. In order to fully learn the valid information in the raw power data, the model is pre-trained using a pre-training module, the local and global temporal feature information of the data is extracted using 1D CNN and Transformer, feature learning is performed by Local-Global window attention, and feature fusion is performed. Then, a Category-aware Attention module is proposed to assign weights to power data features in different generation modes and learn to contextual information, and then perform self-supervised learning. Finally, the prediction of carbon emission and carbon emission source percentage situation is carried out by combining the generation side information such as weather information, wire status information and energy storage element status information. The experiments show that the model can improve the accuracy of carbon emission prediction more effectively than the baseline model.
更多查看译文
AI 理解论文
溯源树
样例
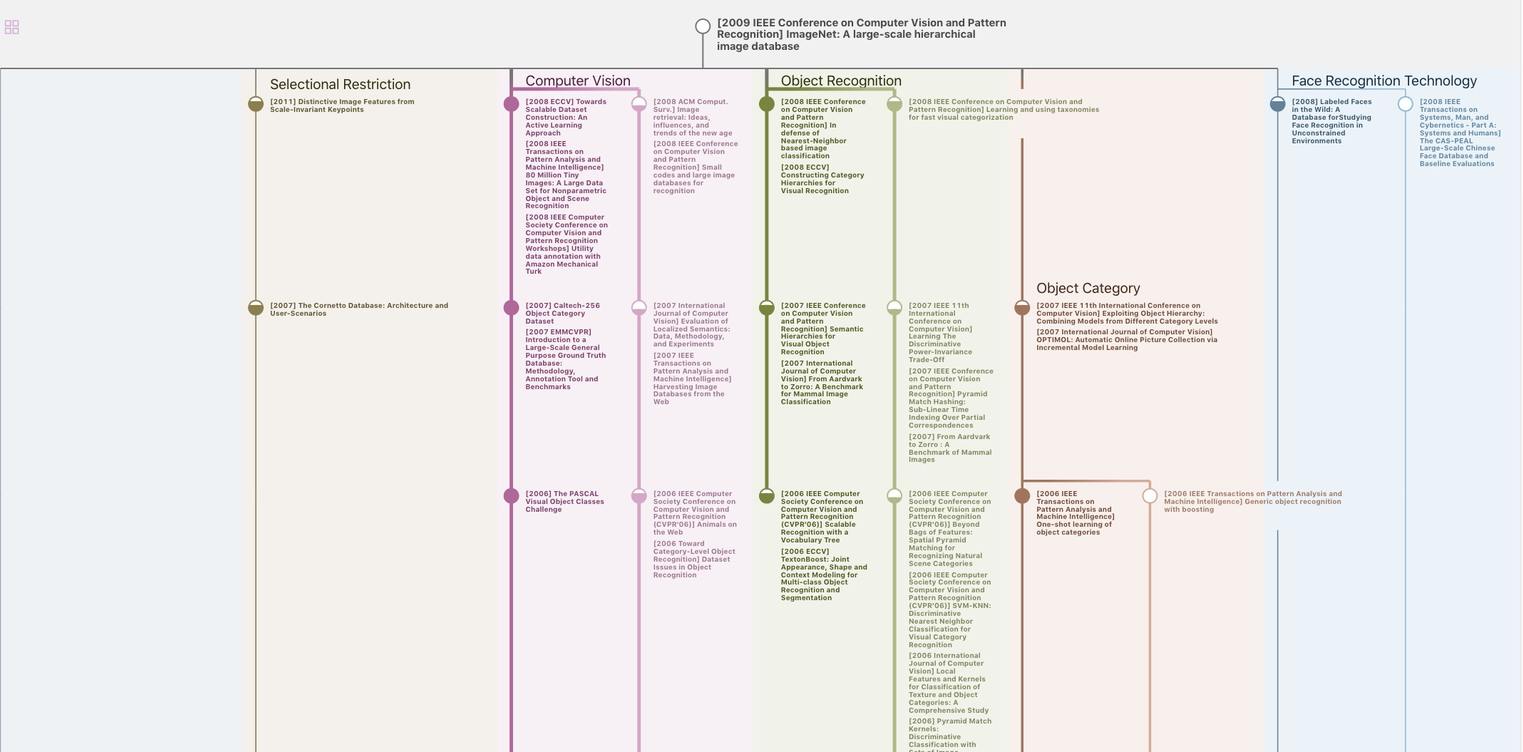
生成溯源树,研究论文发展脉络
Chat Paper
正在生成论文摘要