Grasp Planning with CNN for Log-loading Forestry Machine.
2023 IEEE INTERNATIONAL CONFERENCE ON ROBOTICS AND AUTOMATION (ICRA 2023)(2023)
关键词
CNN,grasp planning pipeline,grasping rate,high quality grasps,intelligent grasping,large-scale log-loading test-bed,localization uncertainties,log characterization,log-loading forestry machine,log-loading machine,simulated grasping trials,virtual depth camera,wood logs
AI 理解论文
溯源树
样例
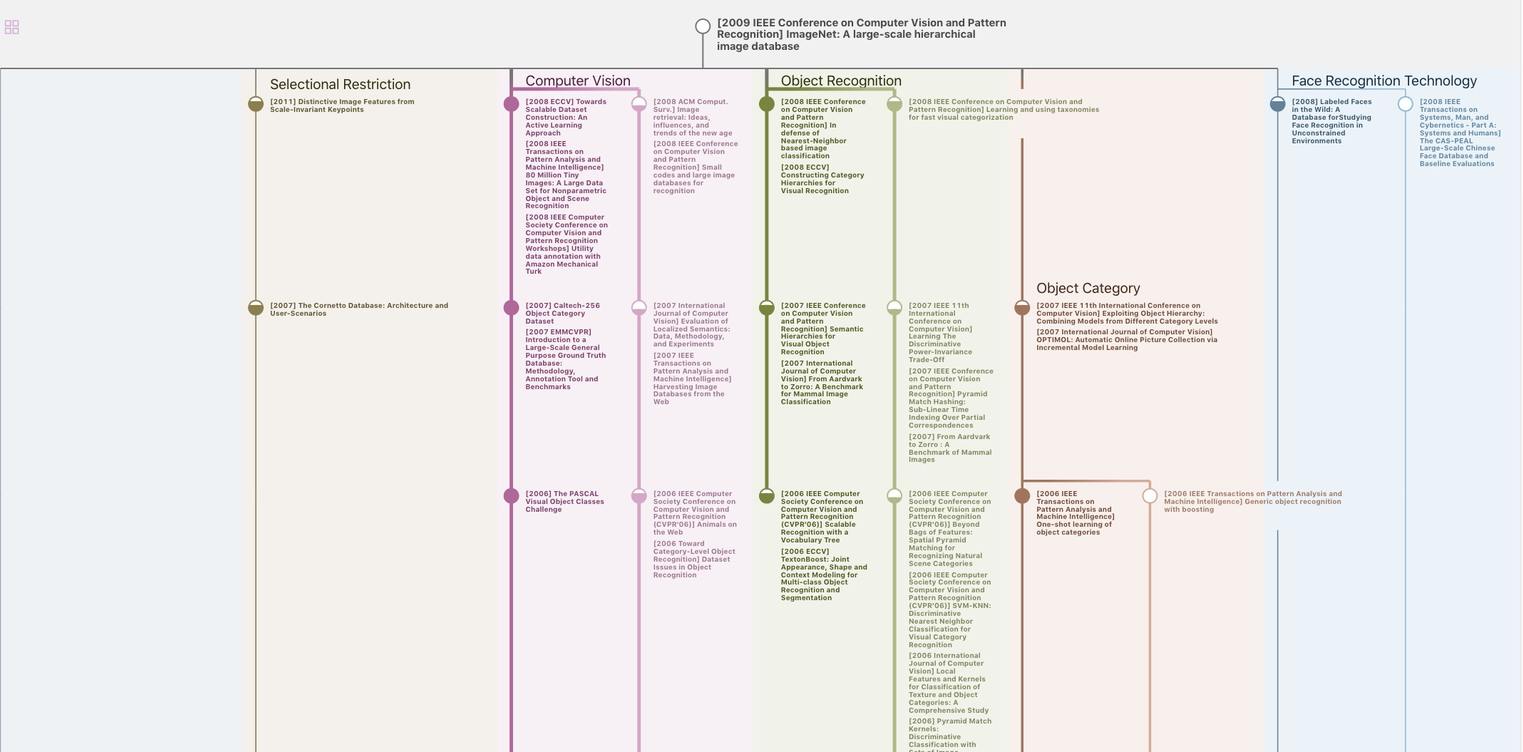
生成溯源树,研究论文发展脉络
Chat Paper
正在生成论文摘要