Risk-Aware Neural Navigation From BEV Input for Interactive Driving
2023 IEEE INTERNATIONAL CONFERENCE ON ROBOTICS AND AUTOMATION, ICRA(2023)
摘要
Safety has been a key goal for autonomous driving since its inception, and we believe recognizing and responding to risk is a key component of safety. In this work, we aim to answer the question, "How can explainable risk representations be generated and used to produce risk-averse trajectories?" To answer this question, previous work uses risk metrics to formulate an optimization problem. In contrast, our work is based on research showing the usefulness of grids as a representation to generate image-based risk maps through a trained neural network. We propose a method of determining risk from a bird's eye view (BEV) of an autonomous vehicle's surroundings. Our method consists of (1) a risk map generator, which is trained to recognize risk associated with nearby agents and the map, (2) differentiable value iteration using the risk map to learn a policy, and (3) a trajectory sampler, which samples from this policy to generate a trajectory. We evaluate our planner in a close-loop manner and find improvements in its overall ability to mimic human driving while maintaining comparable safety statistics. Self-ablation also reveals the potential for fine-tuning the behavior of the planner given a designer's needs.
更多查看译文
AI 理解论文
溯源树
样例
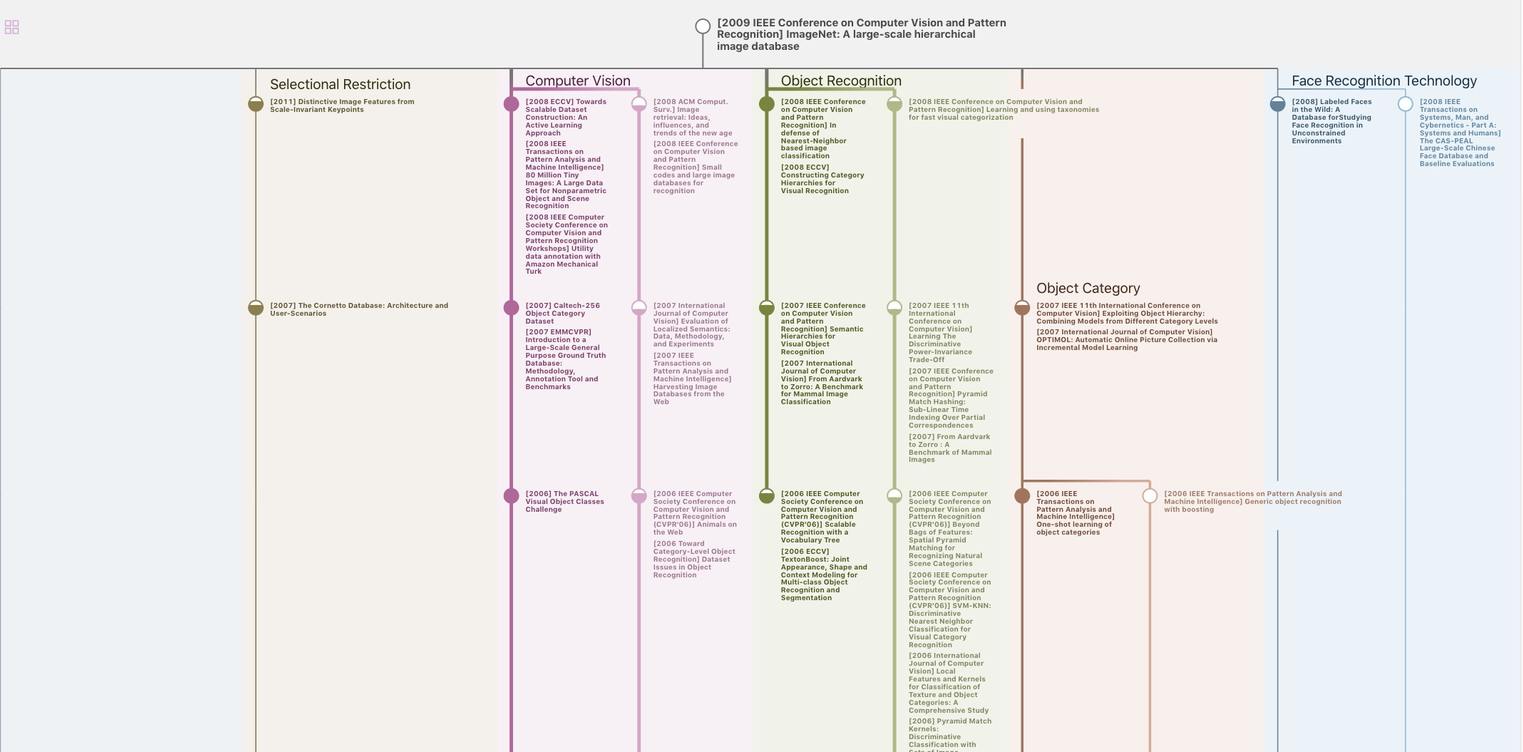
生成溯源树,研究论文发展脉络
Chat Paper
正在生成论文摘要