Using Learning Curve Predictions to Learn from Incorrect Feedback.
ICRA(2023)
摘要
Robots can incorporate data from human teachers when learning new tasks. However, this data can often be noisy, which can cause robots to learn slowly or not at all. One method for learning from human teachers is Human-in-the-loop Reinforcement Learning (HRL), which can combine information from both an environmental reward and external feedback from human teachers. However, many HRL methods assume near-perfect information from teachers or must know the skill level of each teacher before starting the learning process. Our algorithm, Classification for Learning Erroneous Assessments using Rewards (CLEAR), is a feedback filter for Reinforcement Learning (RL) algorithms, enabling learning agents to learn from imperfect teachers without prior modeling. CLEAR is able to determine whether human feedback is correct based on observations of the RL learning curve. Our results suggest that CLEAR improves the quality of human feedback - from 57.5% to 65% correct in a human study - and performs more reliably than baselines by matching or outperforming RL without human teachers in all tested cases.
更多查看译文
关键词
classification for learning erroneous assessments using rewards,CLEAR,HRL,human-in-the-loop reinforcement learning,incorrect feedback,learning curve predictions,reinforcement learning algorithms,RL learning curve
AI 理解论文
溯源树
样例
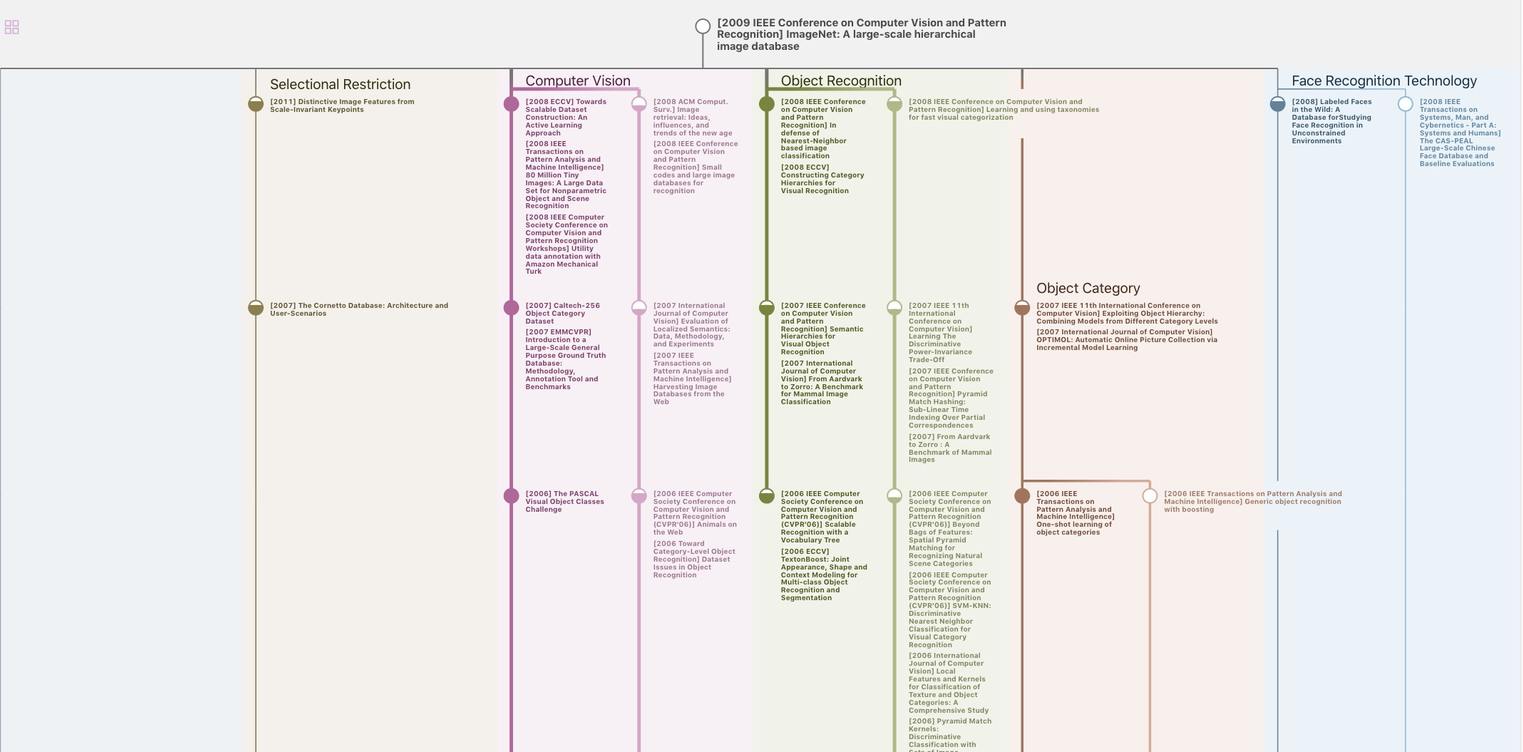
生成溯源树,研究论文发展脉络
Chat Paper
正在生成论文摘要