GSMR-CNN: An End-to-End Trainable Architecture for Grasping Target Objects from Multi-Object Scenes
2023 IEEE INTERNATIONAL CONFERENCE ON ROBOTICS AND AUTOMATION, ICRA(2023)
摘要
We present an end-to-end trainable multi-task model that locates and retrieves target objects from multi-object scenes. The model is an extension of the Siamese Mask R-CNN, which combines the components of Siamese Neural Networks (SNNs) and Mask R-CNN for performing one-shot instance segmentation. The proposed network, called Grasping Siamese Mask R-CNN (GSMR-CNN), extends Siamese Mask R-CNN by adding an additional branch for grasp detection in parallel to the previous object detection head branches. This allows our model to identify a target object with a suitable grasp simultaneously, as opposed to other approaches that require the training of separate models to achieve the same task. The inherent SNN properties enable the proposed model to generalize and recognize new object categories that were not present during training, which is beyond the capabilities of standard object detectors. Moreover, an end-to-end solution uses shared features entailing less model parameters. The model achieves grasp accuracy scores of 92.1% and 90.4% on the OCID grasp dataset on image-wise and object-wise splits. Physical experiments show that the model achieves a grasp success rate of 76.4% when correctly identifying the object. Code and models are available at https://github.com/valerija-h/grasping_siamese_mask_rcnn.
更多查看译文
AI 理解论文
溯源树
样例
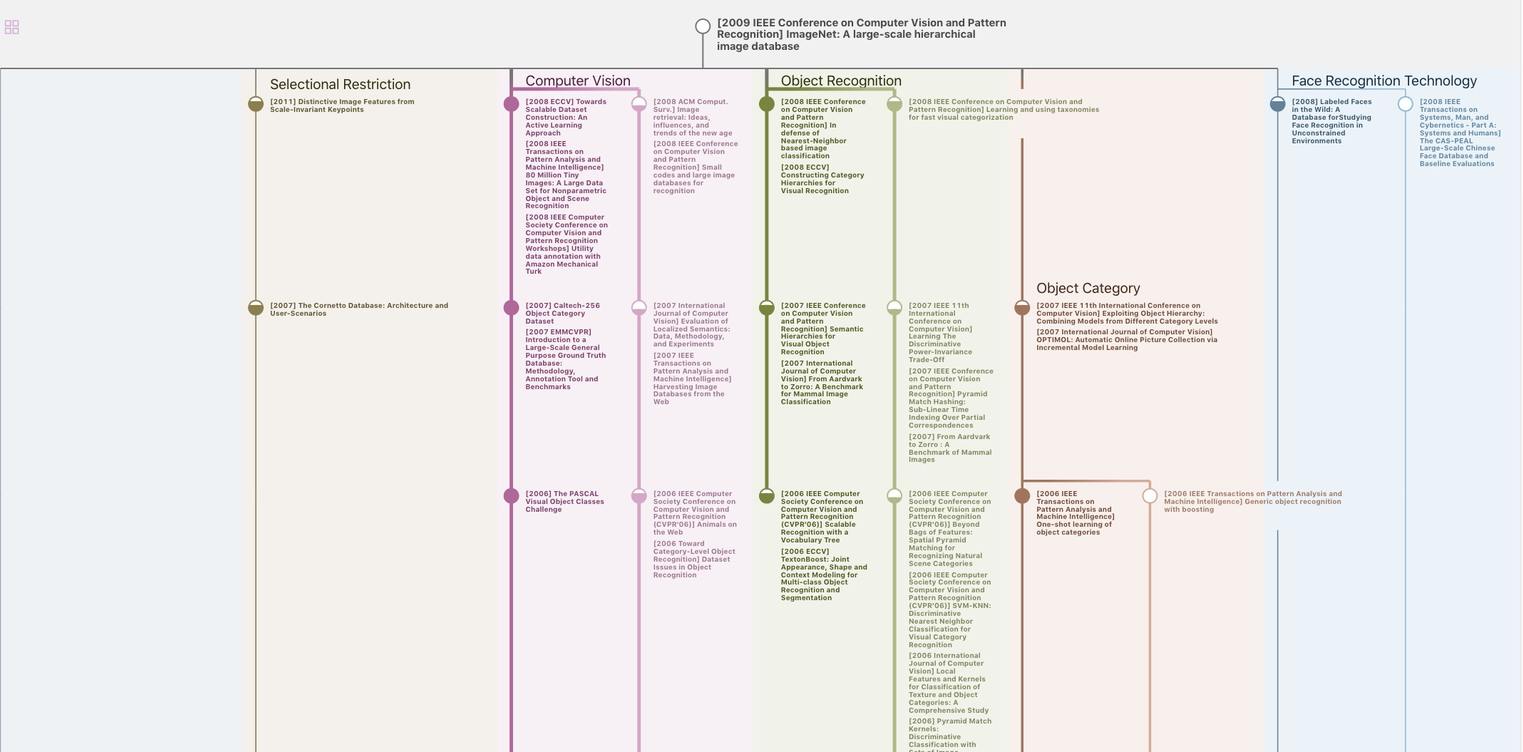
生成溯源树,研究论文发展脉络
Chat Paper
正在生成论文摘要