Optimal Scheduling of Models and Horizons for Model Hierarchy Predictive Control.
ICRA(2023)
摘要
Model predictive control (MPC) is a powerful tool to control systems with non-linear dynamics and constraints, but its computational demands impose limitations on the dynamics model used for planning. Instead of using a single complex model along the MPC horizon, model hierarchy predictive control (MHPC) reduces solve times by planning over a sequence of models of varying complexity within a single horizon. Choosing this model sequence can become intractable when considering all possible combinations of reduced order models and prediction horizons. We propose a framework to systematically optimize a model schedule for MHPC. We leverage trajectory optimization (TO) to approximate the accumulated cost of the closed-loop controller. We trade off performance and solve times by minimizing the number of decision variables of the MHPC problem along the horizon while keeping the approximate closed-loop cost near optimal. The framework is validated in simulation with a planar humanoid robot as a proof of concept. We find that the approximated closed-loop cost matches the simulated one for most of the model schedules, and show that the proposed approach finds optimal model schedules that transfer directly to simulation, and with total horizons that vary between 1.1 and 1.6 walking steps.
更多查看译文
关键词
approximate closed-loop cost,choosing this model sequence,closed-loop controller,dynamics model,model hierarchy predictive control,model predictive control,model schedule,MPC horizon,optimal model schedules,optimal scheduling,prediction horizons,reduced order models,single complex model,single horizon
AI 理解论文
溯源树
样例
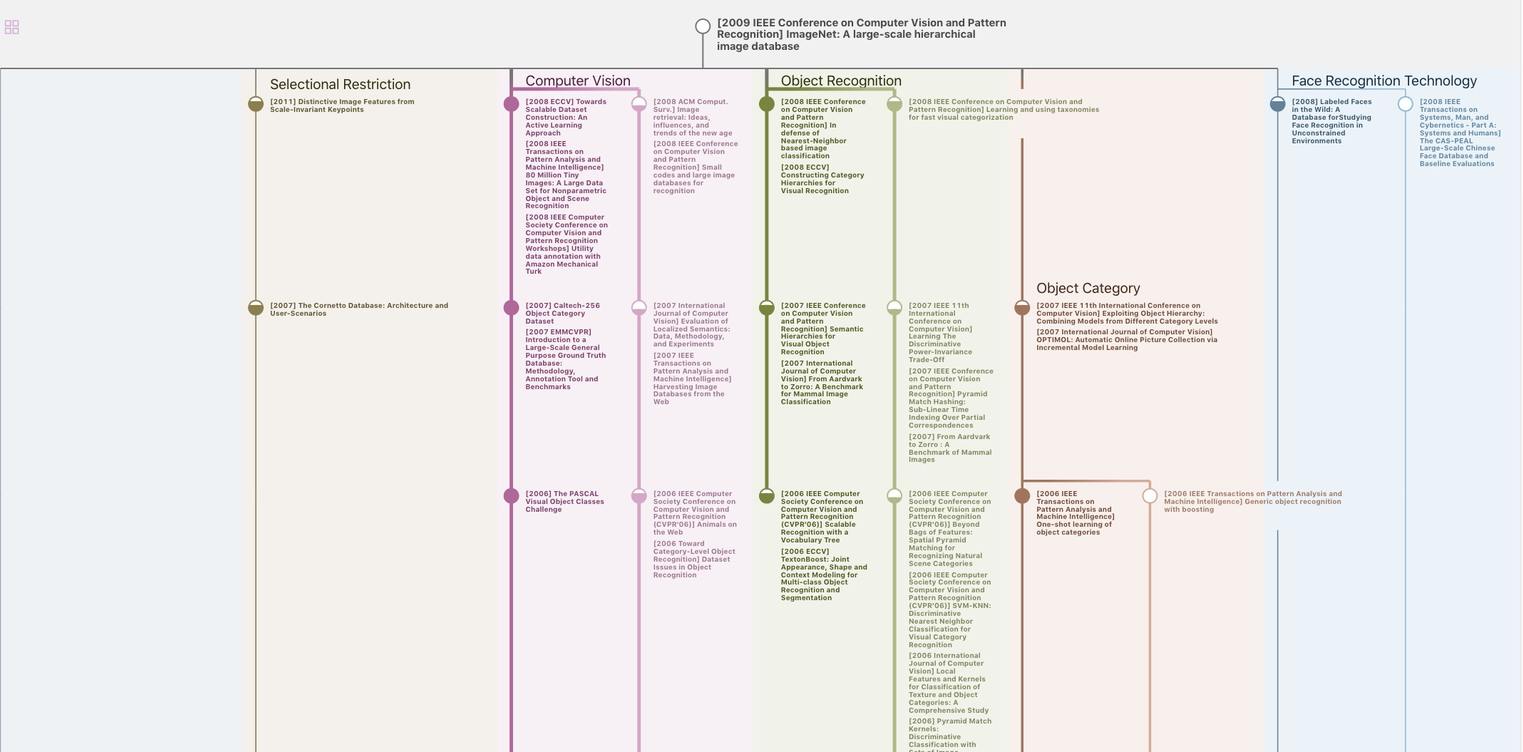
生成溯源树,研究论文发展脉络
Chat Paper
正在生成论文摘要