A deep learning anomaly detection framework with explainability and robustness
18TH INTERNATIONAL CONFERENCE ON AVAILABILITY, RELIABILITY & SECURITY, ARES 2023(2023)
摘要
The prevalence of encrypted Internet traffic has resulted in a pressing need for advanced analysis techniques for traffic analysis and classification. Traditional rule-based and signature-based approaches have been hindered by the introduction of network encryption methods. With the emergence of machine learning (ML) and deep learning (DL), several preliminary works have been developed for anomaly detection in encrypted network traffic. However, complex Artificial Intelligence (AI) models like neural networks lack explainability, limiting the understanding of their predictions. To address this limitation, eXplainable Artificial Intelligence (XAI) has emerged, aiming to provide users with a rationale for understanding AI system outputs and fostering trust. However, existing explainable frameworks still lack comprehensive support for adversarial attacks and defenses. In this paper, we present Montimage AI Platform (MAIP), a new GUI-based deep learning framework for malicious traffic detection and classification combined with its ability of explaining the decision of the model. We employ popular XAI methods to interpret the prediction of the developed deep learning model. Furthermore, we perform adversarial attacks to assess the accountability and robustness of our model via different quantifiable metrics. We perform extensive experiments with both public and private network traffic. The experimental results demonstrate that our model achieves high performance and robustness, and its outcomes align closely with the domain knowledge.
更多查看译文
关键词
Network Security,Encrypted Traffc Analysis,Malware Detection,Deep Learning,Explainable AI,Adversarial Attacks
AI 理解论文
溯源树
样例
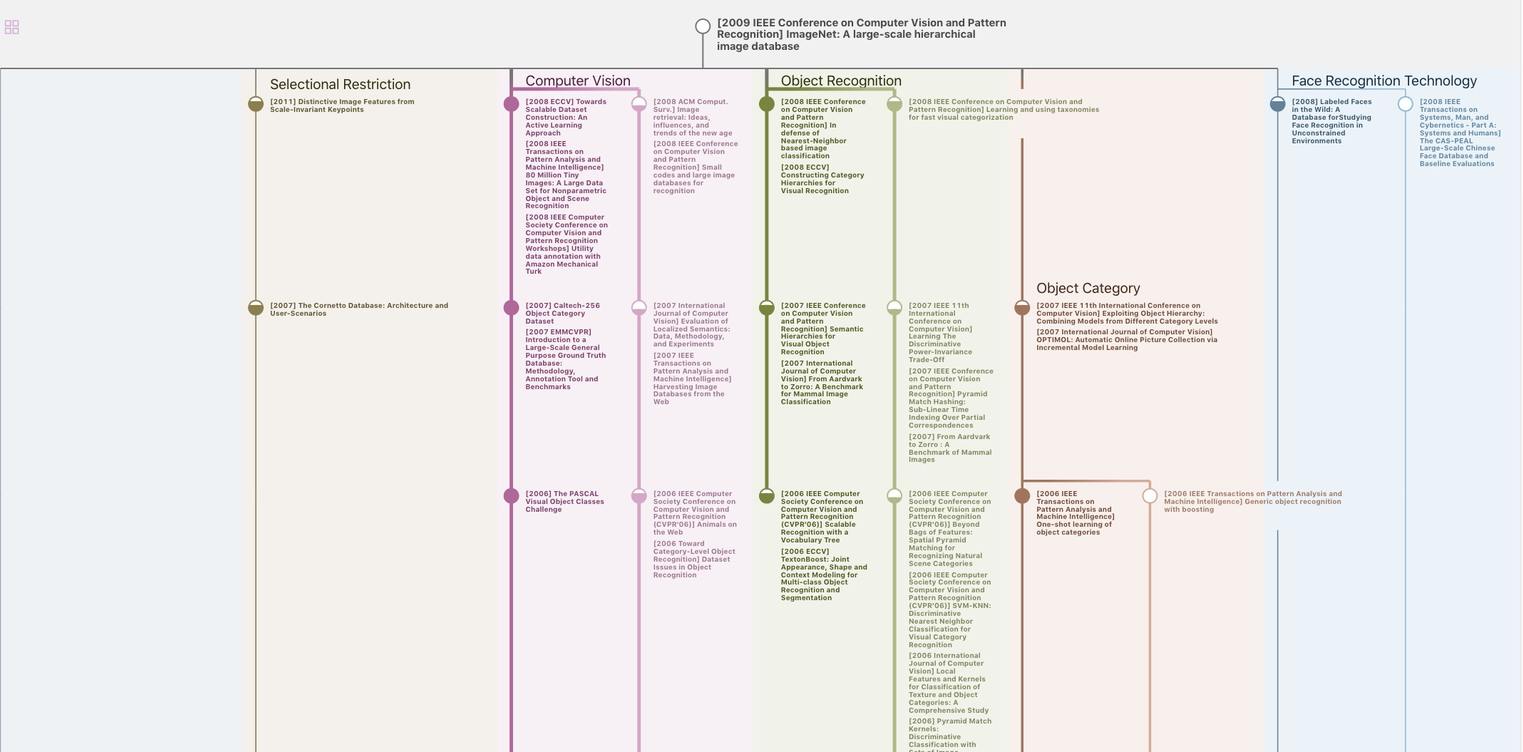
生成溯源树,研究论文发展脉络
Chat Paper
正在生成论文摘要