Credible Online Dynamics Learning for Hybrid UAVs
2023 IEEE INTERNATIONAL CONFERENCE ON ROBOTICS AND AUTOMATION, ICRA(2023)
摘要
Hybrid unmanned aerial vehicles (H-UAVs) are highly versatile platforms with the ability to transition between rotary- and fixed-wing flight. However, their (aero)dynamics tend to be highly nonlinear which increases the risk of introducing safety-critical modeling errors in a controller. Designing a safe, yet not too cautious controller, requires a credible model which provides accurate dynamics uncertainty quantification. We present a data-efficient, probabilistic semi-parametric dynamics modeling approach that allows for online, filter-based inference. The proposed model leverages prior knowledge using a nominal parametric model, and combines it with residuals in the form of sparse Gaussian processes to account for possibly unmodeled forces and moments. Uncertain nominal and residual parameters are jointly estimated using Bayesian filtering. The resulting model accuracy and the reliability of its predicted uncertainty are analyzed for both a simulated and a real example, where we learn the 6DoF nonlinear dynamics of a tiltwing H-UAV from a few minutes of flight data. Compared to a residual-free nominal model, the proposed semi-parametric approach provides increased model accuracy in relevant parts of the flight envelope and substantially higher credibility overall.
更多查看译文
AI 理解论文
溯源树
样例
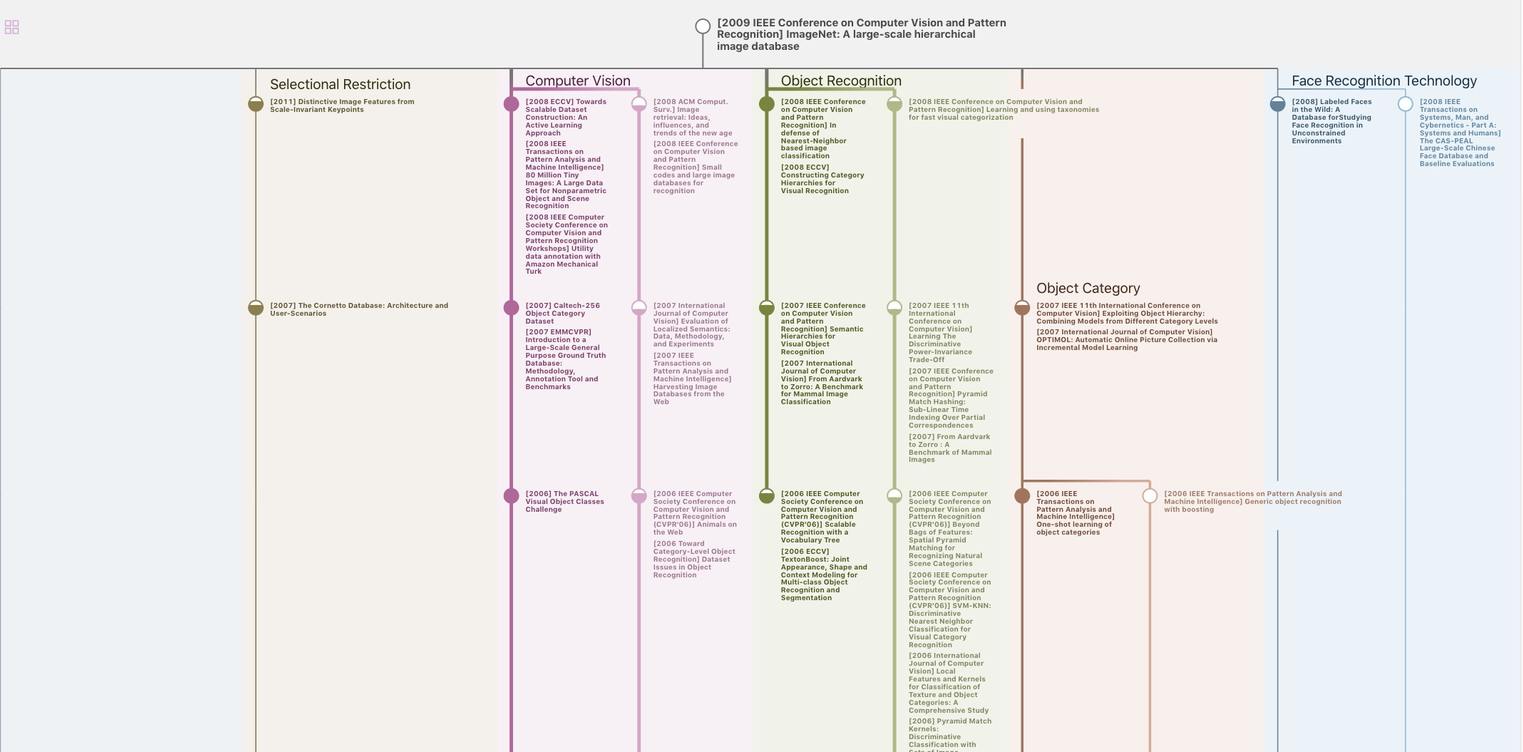
生成溯源树,研究论文发展脉络
Chat Paper
正在生成论文摘要