Unsupervised Learning of Depth and Pose Based on Monocular Camera and Inertial Measurement Unit (IMU).
ICRA(2023)
摘要
The main content of the research in this paper is the estimation of depth and pose based on monocular vision and Inertial Measurement Unit (IMU). The usual depth estimation network and pose estimation network require depth ground truth or pose ground truth as a supervised signal for training, while the depth ground truth and pose ground truth are hard to obtain, and monocular vision based depth estimation cannot predict absolute depth. In this paper, with the help of IMU, which is inexpensive and widely used, we can obtain angular velocity and acceleration information. Two new supervision signals are proposed and the calculation expressions are given. Among them, the model trained with acceleration constraint shows a good ability to estimate the absolute depth during the test. It can be considered that the model can estimate the absolute depth. We also derive the method of estimating the scale factor during the test from the acceleration constraint, and also achieve good results as the acceleration constraint does. In addition, this paper also studies the method of using IMU information as pose network input and as selecting conditions. Moreover, it analyzes and discusses the experimental results. At the same time, we also evaluate the effect of the pose estimation of the relevant models. This article starts by reviewing the achievements and deficiencies of the work in this field, combines the use of IMU, puts forward three new methods such as a new loss function, and conducts a test analysis and discussion of relevant indicators on the KITTI data set.
更多查看译文
关键词
absolute depth,acceleration constraint,acceleration information,angular velocity,depth ground truth,IMU information,Inertial Measurement Unit,monocular camera,monocular vision based depth estimation,pose estimation,pose network input,supervised signal,supervision signals,usual depth estimation network
AI 理解论文
溯源树
样例
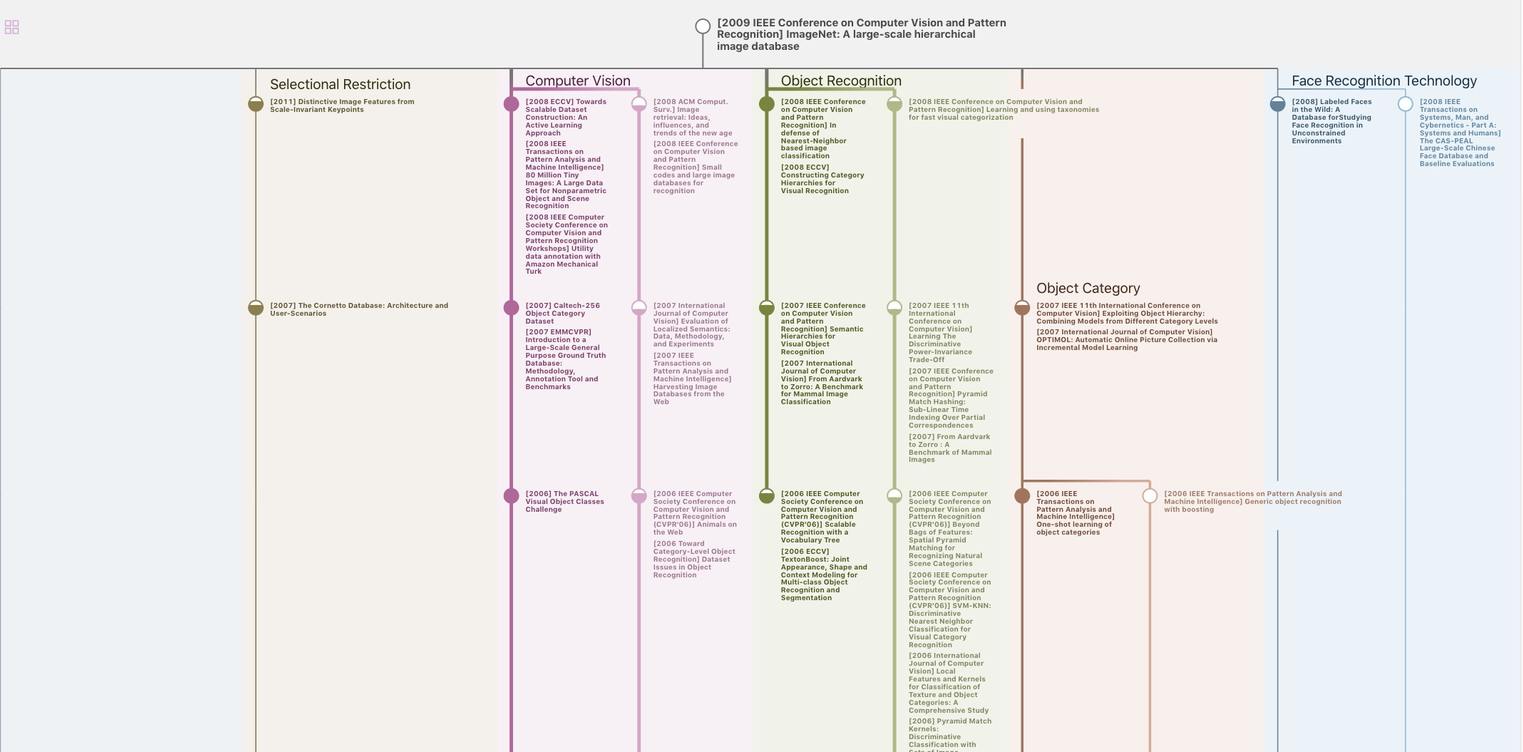
生成溯源树,研究论文发展脉络
Chat Paper
正在生成论文摘要