Zero-shot Object Detection Based on Dynamic Semantic Vectors.
ICRA(2023)
摘要
Zero-shot object detection has shown its ability to overcome the problems of data scarcity and novel classes. Existing methods generally utilize static semantic vectors to classify objects and guide the network to map visual features to semantic vectors. However, the distribution of semantic vectors cannot adequately represent visual features, which makes migration from seen to unseen classes difficult. This work explores the dynamic semantic vector method to align the distributions of semantic vectors and visual features. The main challenge is to get a more reasonable distribution of semantic vectors. To address this issue, we proposed a two-way classification branch network and introduce N-pair loss into the dynamic semantic vector optimization process. Experiments on the MS-COCO dataset and SiTi (a real-world autonomous driving dataset collected by us) demonstrate the effectiveness and generalization of our method. Our code is available at https://github.com/HaoyuLizju/ZSD tcb
更多查看译文
关键词
dynamic semantic vector method,dynamic semantic vector optimization process,dynamic semantic vectors,map visual features,static semantic vectors,zero-shot object detection
AI 理解论文
溯源树
样例
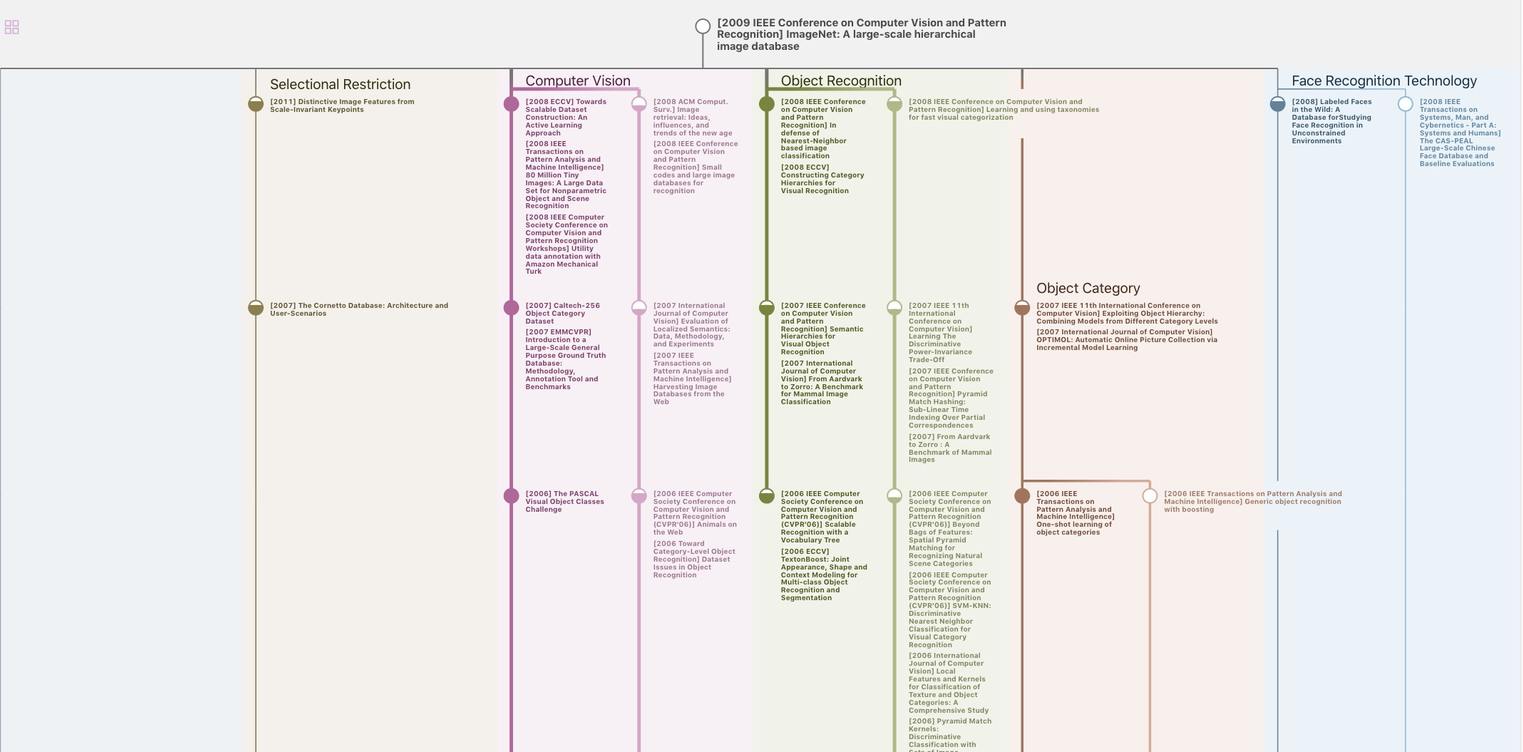
生成溯源树,研究论文发展脉络
Chat Paper
正在生成论文摘要