Few Shot Object Detection with Incompletely Annotated Samples.
IJCNN(2023)
摘要
Few shot object detection aims to generalize the model to previously unseen classes with only a few training samples, which has been attached great attention due to its practicability in real scenes. Many existing methods hold the missed detection issue, mainly due to the problem of incompletely annotated samples. Specifically, only partial objects in a sample are labeled, resulting in unlabeled objects being used as the background for training. This problem is especially serious for few-shot learning. To solve this noisy label problem, we first propose a label calibration method based on confidence to correct potential incorrect labels, and then introduce the class center library to eliminate the negative impact of unlabeled objects. We conduct extensive experiments on PASCAL VOC and MS-COCO benchmarks, which proves the effectiveness of our approach and achieves the state-of-the-art results.
更多查看译文
AI 理解论文
溯源树
样例
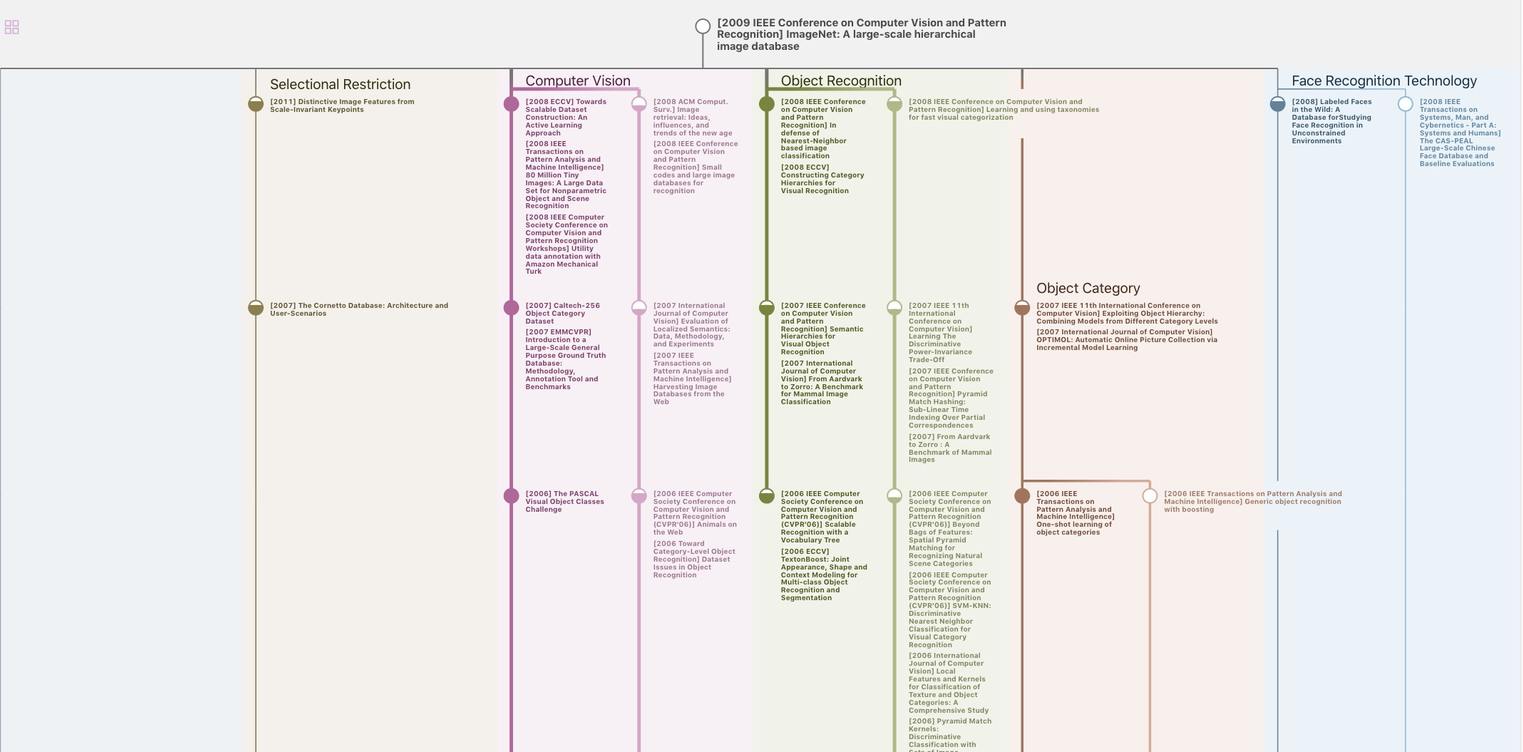
生成溯源树,研究论文发展脉络
Chat Paper
正在生成论文摘要