Cooperation Skill Motivated Reinforcement Learning for Traffic Signal Control.
IJCNN(2023)
摘要
Recently, Reinforcement Learning (RL) has shown superior performance in traffic signal control (TSC) and can effectively mitigate traffic congestion. However, two key challenges still need to be addressed. First, a clear mechanism to coordinate different intersections is essential for efficient TSC, and the investigation of diverse coordination mechanisms is needed in various traffic scenarios, which remains unsolved in current works. Second, existing research tries to optimize area traffic efficiency by manually designing rewards with local short-time-horizon metrics based on different expert experiences, which requires cumbersome work and may not align with the area control objective. To address these challenges, a novel cooperation skill motivated TSC algorithm (CoST) with a modular framework is proposed in this paper. Cooperation skill in CoST is an innovative method to directly investigate diverse coordination mechanisms between neighboring intersections without manually reward design. The cooperation skill is formulated in a hierarchical structure with a cooperative policy and an execution policy, which are used to determine the cooperative probabilities between intersections and to realize such cooperation, respectively. Besides, a distinguishable feature extraction network is introduced to encourage the learning of diverse functionalities. Then based on learned cooperation skills, a meta policy is formulated to choose skills of different intersections to achieve optimum area transportation efficiency. We evaluate our method on two synthetic networks and two real-world networks using SUMO, and the results show the superiority of our method over the state-of-the-art methods.
更多查看译文
关键词
Traffic Signal Control,Skill Discovery,Deep Reinforcement Learning,Coordination Mechanism
AI 理解论文
溯源树
样例
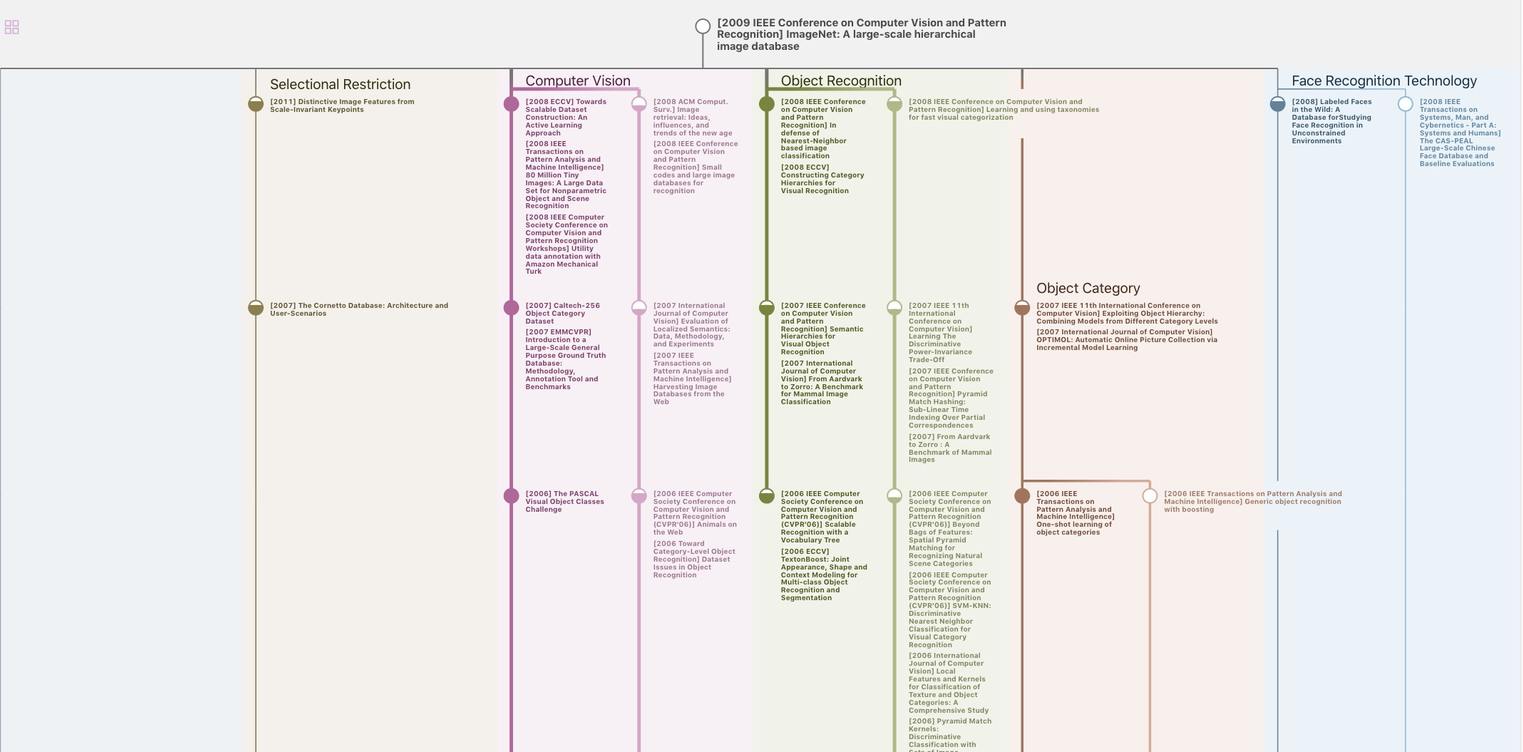
生成溯源树,研究论文发展脉络
Chat Paper
正在生成论文摘要