Towards infrared human pose estimation via Transformer.
IJCNN(2023)
摘要
Due to the limited color information and low hierarchy present in infrared images, traditional CNN-based pose estimation networks designed for visible light often exhibit weak performance when applied to infrared images for human pose estimation. In order to overcome the inherent shortcomings of infrared images and improve the accuracy of human pose estimation in this domain, we propose a novel model called FEPose.Our model incorporates the Transformer Encoder architecture to establish correlation dependencies in the infrared image space, enhancing the network's ability to sense spatial distance and mitigating the impact of low hierarchy on detection accuracy. Additionally, we introduce a specially-designed FELayer layer for infrared images, which enhances the network's response to human grayscale values while reducing the impact of background interference factors.To evaluate the effectiveness of our model, we conduct experiments on a self-built IR multi-person pose estimation dataset comprising 7621 training instances and 1082 test instances. Our most complex model achieves a PCKm of 77.5, while the simplified model achieves 75.1 PCKm.
更多查看译文
关键词
estimation dataset,estimation networks,human grayscale values,infrared human pose estimation,infrared image space,infrared images
AI 理解论文
溯源树
样例
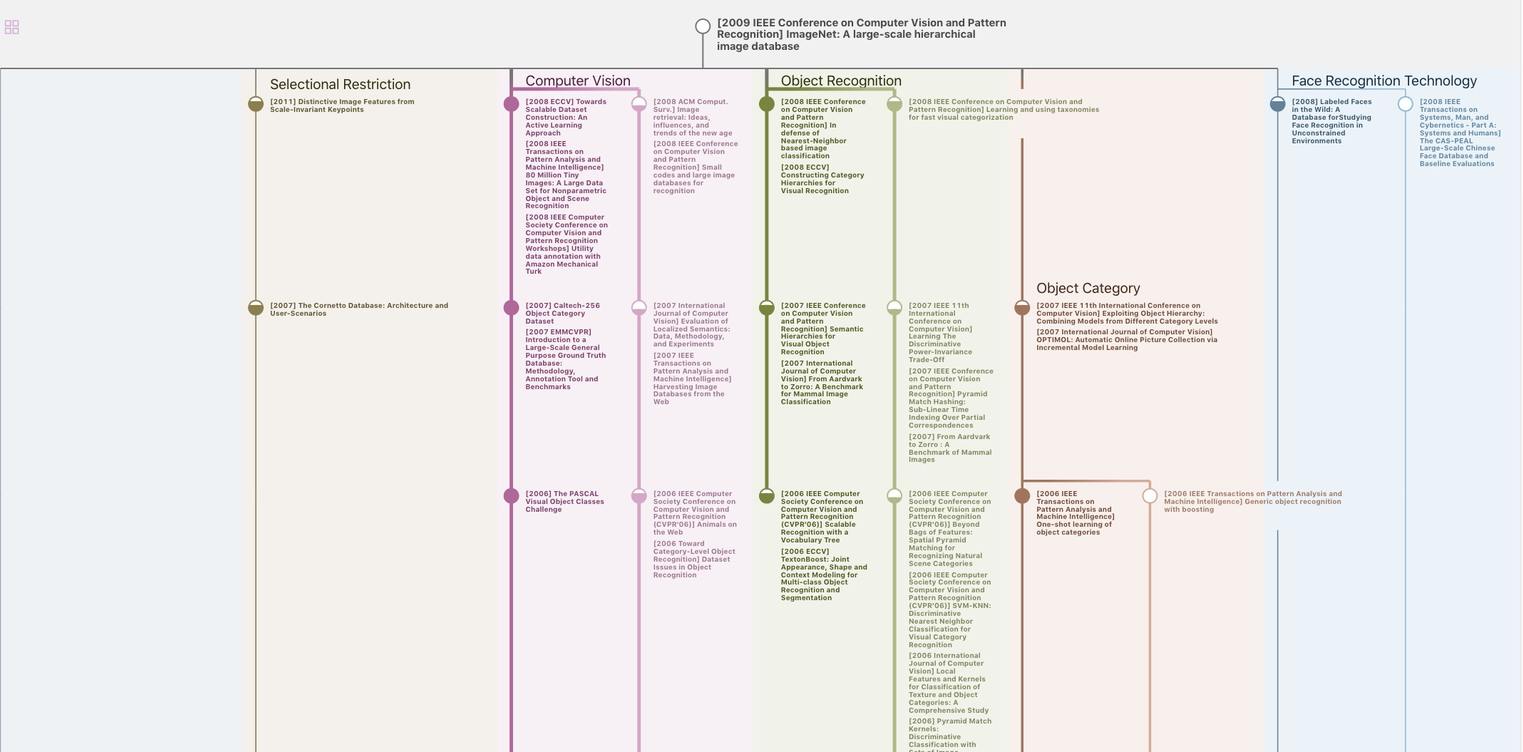
生成溯源树,研究论文发展脉络
Chat Paper
正在生成论文摘要