SegQNAS: Quantum-inspired Neural Architecture Search applied to Medical Image Semantic Segmentation.
IJCNN(2023)
Abstract
Semantic segmentation can be applied to a wide range of applications. One of the most interesting is medical image analysis. Applying semantic segmentation techniques in that field has great potential to assist physicians in diagnosing and analysing medical scans for the patient's benefit. Traditionally, several techniques have been applied in order to perform semantic segmentation. However, with the development of Deep Learning methods, there was a paradigm shift. Deep learning techniques are able to achieve great results, comparable to human-level performance. In order to achieve state-of-the-art results, researchers have to lean on the task of designing novel deep neural network architectures. That process is very time-consuming and heavily relies on experience and expert knowledge. Neural architecture search is the process of automatising the search for new deep neural network architectures. Quantum-Inspired Neural Architecture Search is a neural architecture search algorithm that leverages the benefits of quantum-inspired computing to search for neural network architectures efficiently. In this work, we adapt this to search for semantic segmentation neural networks and apply it to medical image analysis. The Spleen and Prostate datasets from the Medical Segmentation Decathlon challenge were used. Results show that our work was able to find better-performing semantic segmentation architectures for both datasets: 0.9583 +/- 0.006 in comparison to ResU-Net 0.9525 +/- 0.008 for the spleen dataset, and 0.6887 +/- 0.067 in comparison to 0.6529 +/- 0.070 for the prostate dataset.
MoreTranslated text
Key words
Neural Architecture Search, Semantic Segmentation, Medical Image Analysis, Quantum-inspired Evolutionary Algorithms
AI Read Science
Must-Reading Tree
Example
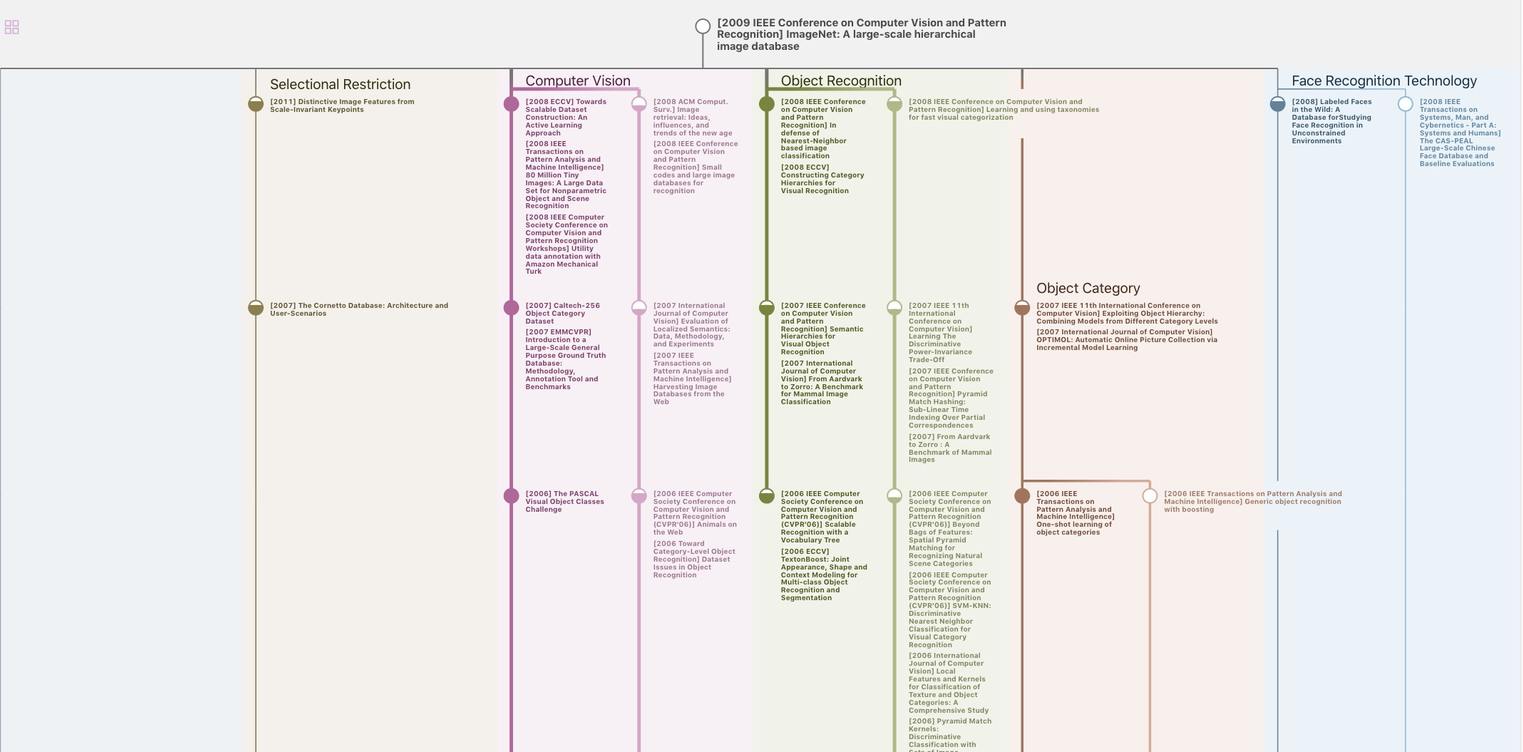
Generate MRT to find the research sequence of this paper
Chat Paper
Summary is being generated by the instructions you defined