A General Heterogeneous Hypergraph Neural Network for Node Classification.
IJCNN(2023)
摘要
Recently, there has been a surge of interest in hypergraph neural networks (HNN) due to their excellent ability to represent non-pair-wise interactions, such as communities in social networks, partnerships in academic cooperation networks, and biochemical interactions in biological networks. Existing works, which classify nodes of hypergraphs, mainly focus on the homogeneous hypergraph. However, the non-uniform and heterogeneous hypergraph is more common in realistic scenarios. Worse yet, homogeneous hypergraph approaches show limited accuracy on the heterogeneous hypergraph. In this paper, we propose a multi-channel hypergraph convolution framework (HHNN) to classify the nodes in the large, non-uniform, and heterogeneous hypergraph. Our framework is composed of two modules: The first decomposition module transforms arbitrary heterogeneous hypergraph to bipartite-form hypergraph (bfHG) so that we can introduce hypergraph structure more efficiently than the other methods. Second, we devise a multi-channel attention hypergraph convolution module (HHCNN), aiming at fusing various information from different kinds of nodes. We conduct experiments on four real-world heterogeneous hypergraph datasets, and the results show that our framework significantly improves the accuracy compared with six state-of-the-art approaches.
更多查看译文
关键词
hypergraph, heterogeneous, non-pair-wise
AI 理解论文
溯源树
样例
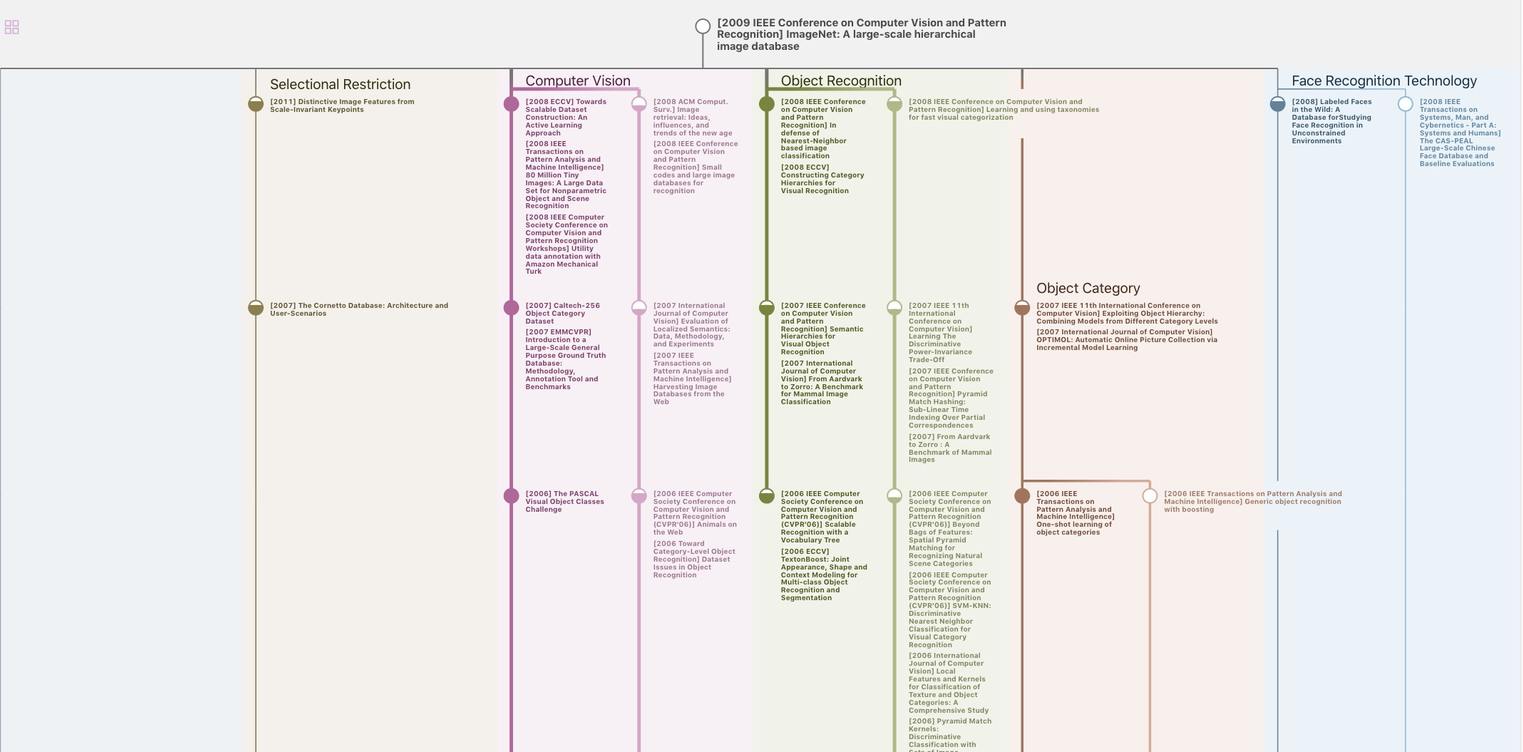
生成溯源树,研究论文发展脉络
Chat Paper
正在生成论文摘要