A Semi-Supervised Learning Method for Spiking Neural Networks Based on Pseudo-Labeling.
IJCNN(2023)
摘要
Supervised learning methods have demonstrated state-of-the-art performance for spiking neural network (SNN) but require a large amount of annotated training data, which may be expensive to obtain. To address this, we propose a semi-supervised learning method based on pseudo-labeling that enables SNN training using a small number of annotated training samples. Our proposed method outperforms the existing semisupervised learning approaches for SNN by more than 17% on the MNIST dataset when 100 annotated samples are used. Our proposed spike-based method is hardware friendly, can be incorporated to various SNN models, and does not involve intensive computations, which is desirable for applications on internet of things (IoT) devices that have tight constraints on hardware resources and energy consumption.
更多查看译文
关键词
Spiking Neural Networks, Semi-Supervised Learning, Pseudo-Labeling
AI 理解论文
溯源树
样例
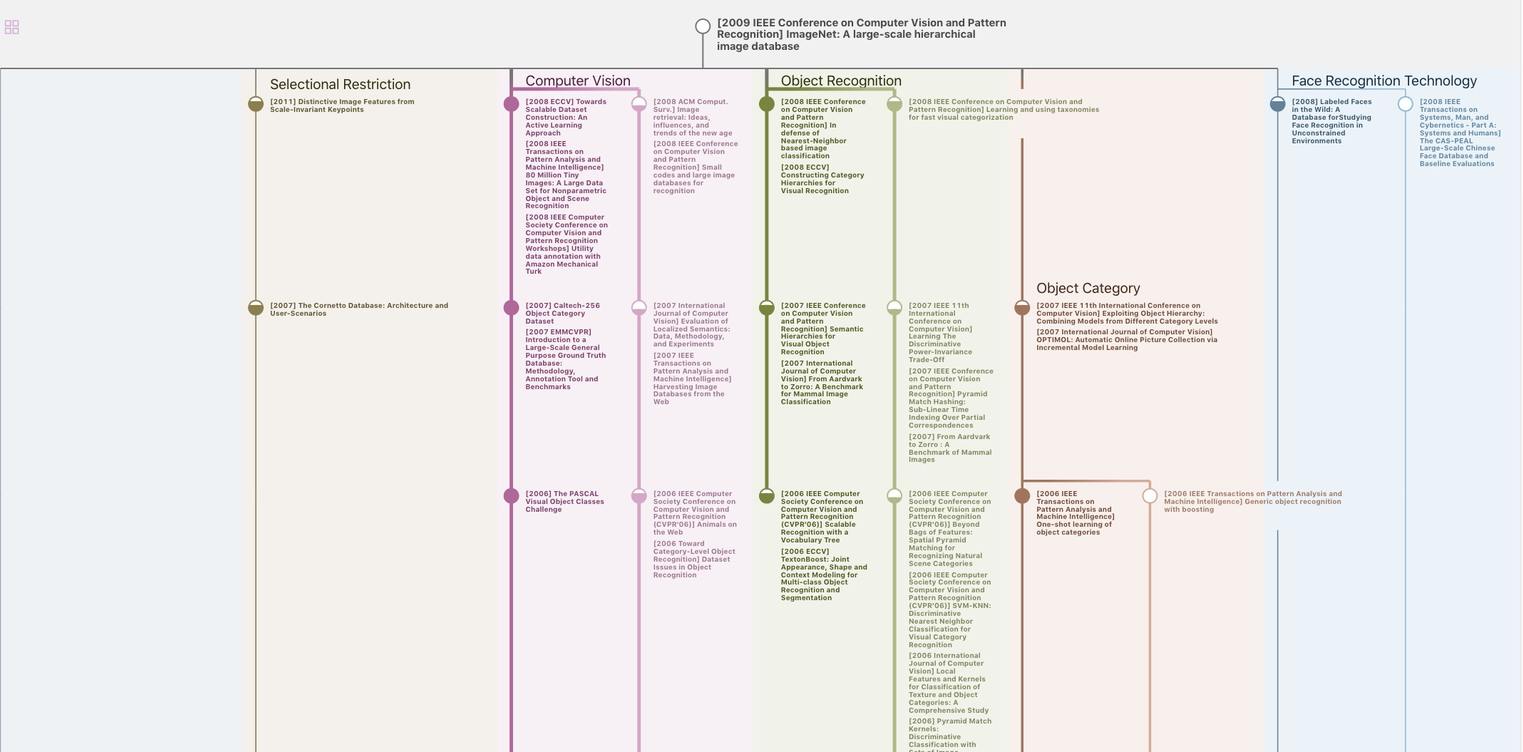
生成溯源树,研究论文发展脉络
Chat Paper
正在生成论文摘要