Underexplored Subspace Mining for Sparse-Reward Cooperative Multi-Agent Reinforcement Learning.
IJCNN(2023)
摘要
Learning cooperation in sparse-reward multi-agent reinforcement learning is challenging, since agents need to explore in the large joint-state space with sparse feedback. However, in cooperative games, the cooperative target is often related to partial attributes, hence there is no need to treat the whole state space equally. Therefore, we propose Underexplored Subspace Mining (USM), a novel type of intrinsic reward that encourages agents to selectively explore partial attributes instead of wasting time on the whole state space to accelerate learning. Specially, considering that the target-related attributes are varying in different games and hard to predefine, we choose to focus on the underexplored subspace as an alternative, which is an automatic aggregation of the underexplored bottom-level dimensions without any human design or learning parameters. We evaluate our method in cooperative games with discrete and continuous state space separately. Results demonstrate that USM consistently outperforms existing state-of-the-art methods, and becomes the only method that has succeeded in sparse-reward games evaluated with larger state space or more complicated cooperation dynamics.
更多查看译文
关键词
sparse-reward cooperation,multi-agent system,reinforcement learning,selective exploration
AI 理解论文
溯源树
样例
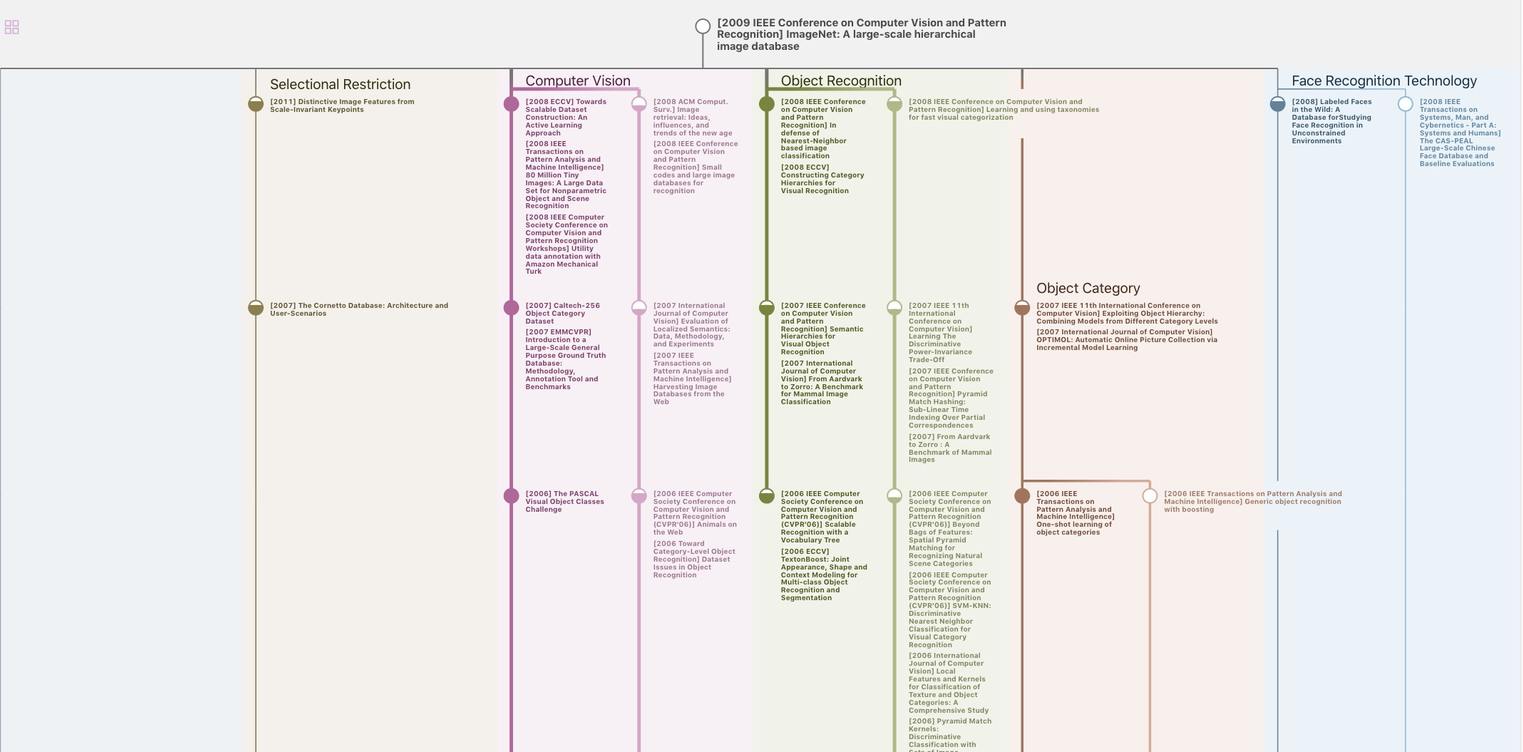
生成溯源树,研究论文发展脉络
Chat Paper
正在生成论文摘要