CAKT: Coupling contrastive learning with attention networks for interpretable knowledge tracing
IJCNN(2023)
摘要
In intelligent systems, knowledge tracing (KT) plays a vital role in providing personalized education. Existing KT methods often rely on students' learning interactions to trace their knowledge states by predicting future performance on the given questions. While deep learning-based KT models have achieved improved predictive performance compared with traditional KT models, they often lack interpretability into the captured knowledge states. Furthermore, previous works generally neglect the multiple semantic information contained in knowledge states and sparse learning interactions. In this paper, we propose a novel model named CAKT that couples contrastive learning with attention networks for interpretable knowledge tracing. Specifically, we use three attention-based encoders to model three dynamic factors of the Item Response Theory (IRT) model, based on designed learning sequences. Then, we identify two key properties related to the knowledge states and learning interactions: consistency and separability. We utilize contrastive learning to incorporate the semantic information of the above properties into the representations of knowledge states and learning interactions. With the training goal of contrastive learning, we can obtain more representative representations of them. Extensive experiments demonstrate the excellent predictive performance of CAKT and the positive effects of considering the two properties. Additionally, CAKT can exhibit high interpretability for captured knowledge states.
更多查看译文
关键词
knowledge tracing, consistency, separability, contrastive learning, attention networks, IRT
AI 理解论文
溯源树
样例
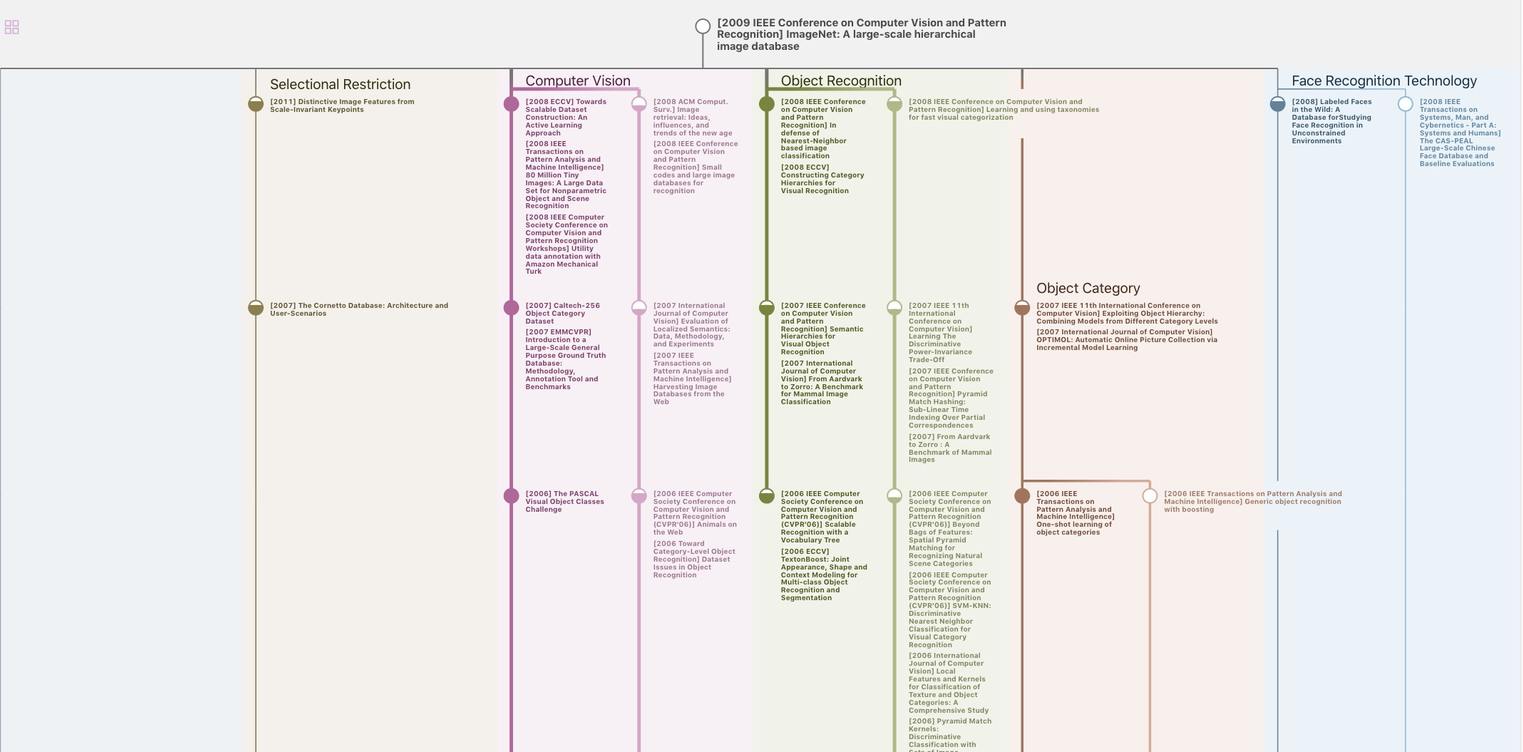
生成溯源树,研究论文发展脉络
Chat Paper
正在生成论文摘要