Improving Complex Knowledge Base Question Answering with Relation-Aware Subgraph Retrieval and Reasoning Network.
2023 INTERNATIONAL JOINT CONFERENCE ON NEURAL NETWORKS, IJCNN(2023)
关键词
knowledge base question answering,graph rea-soning,information retrieval
AI 理解论文
溯源树
样例
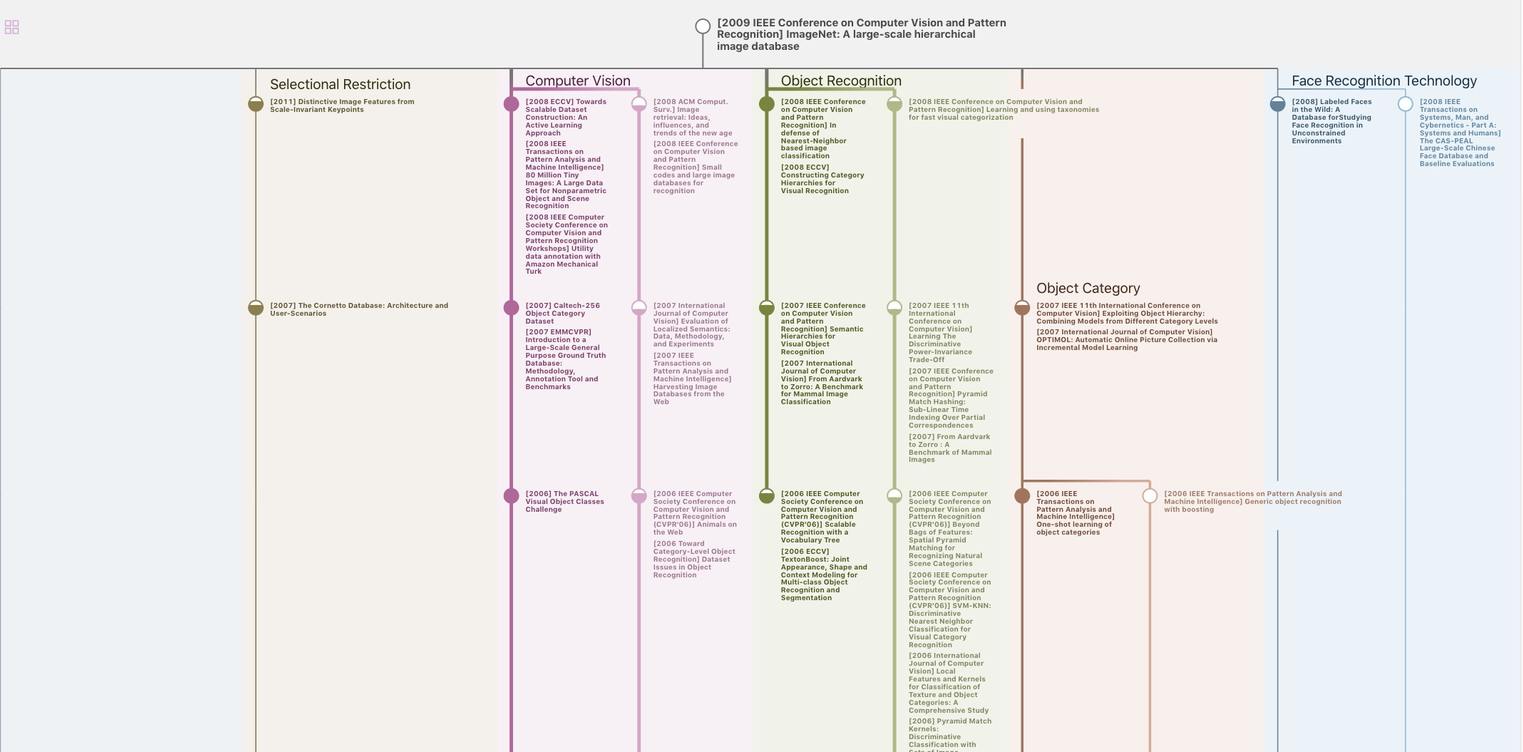
生成溯源树,研究论文发展脉络
Chat Paper
正在生成论文摘要