Adapt-to-Learn Policy Network for Abstractive Multi-document Summarization
IJCNN(2023)
摘要
Abstractive multi-document summarization (MDS) aims to generate a summary for a set of topic-related documents, in which different documents may contain trivial and redundant information and present complementary or contradictory content. It is essential to extract salient information and detect redundancy across documents for abstractive MDS compared with single-document summarization. However, it is challenging for models to exploit salient information and generate concise summaries. In this paper, we propose an Adapt-to-Learn Policy (ALP) network to seamlessly adapt the key sentence selection and word generation over retrieval and generative agents, guided by the multi-document summarization reward. Moreover, our model learns latent dependencies among textual units and explicitly takes advantage of critical information by focusing on semantic similarity or discourse relations. Extensive experiments on WikiSum and Multi-News datasets confirm that our method is superior to prior competitive baselines, and experimental analyses show that higher-quality summaries and more fluent word order can be generated in our ALP network.
更多查看译文
关键词
Adapt-to-Learn Policy,Abstractive Multi-document Summarization,Reinforcement Learning
AI 理解论文
溯源树
样例
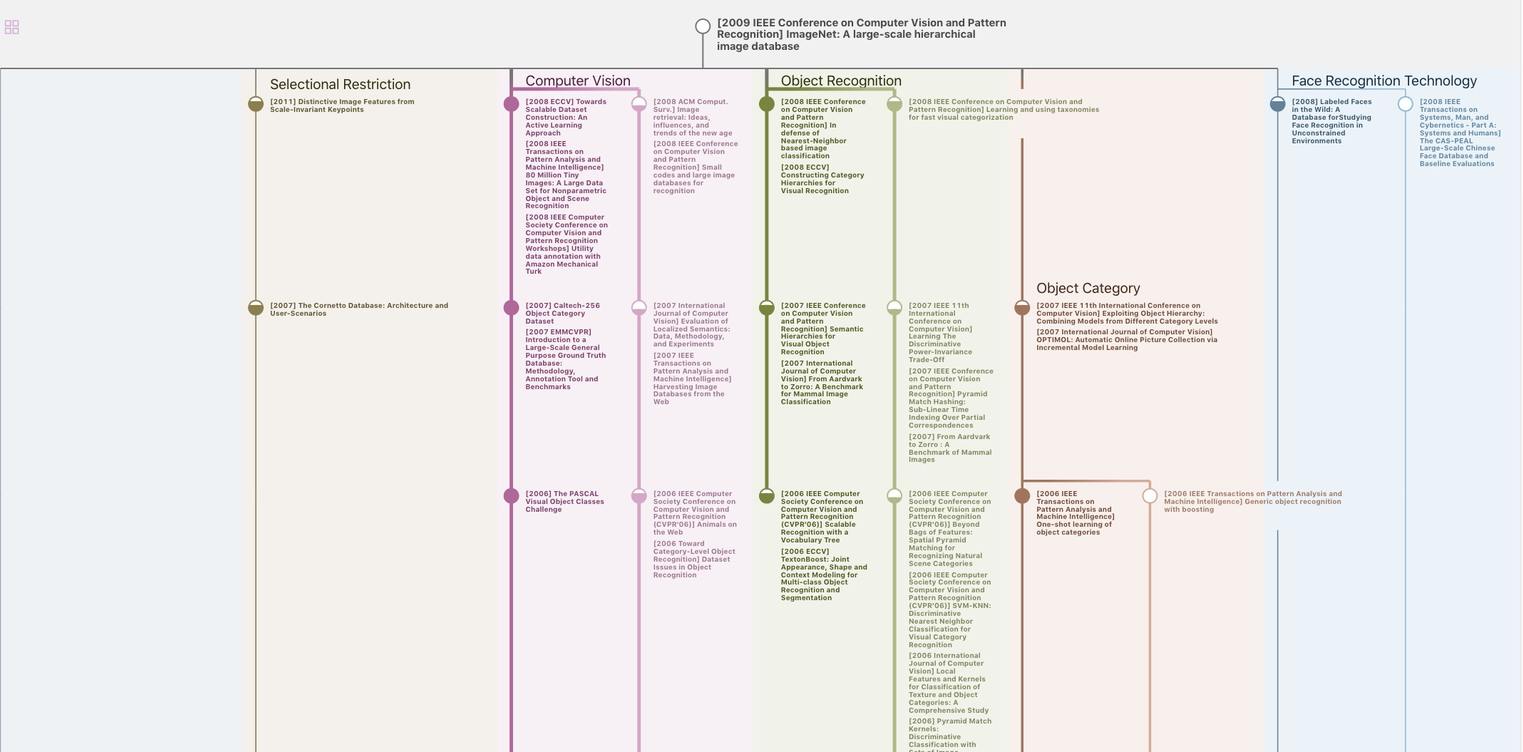
生成溯源树,研究论文发展脉络
Chat Paper
正在生成论文摘要