TransGNN: A Transductive Graph Neural Network with Graph Dynamic Embedding
IJCNN(2023)
摘要
Graph Neural Networks (GNNs) have become a rapidly growing field, due to their ability to capture the relationship among data, instead of only learning from the attribute of the data. The core of any GNN is the graph embedding generation by message passing mechanisms. In this work we propose a new message passing technique based on the Particle Competition and Cooperation (PCC) model, originally developed for community detection in graphs. The proposed framework performs a transductive learning in the network and passes the learned information to the nodes, prior to the inductive learning performed by traditional GNN schemes. The new GNN presents attractive features which overcomes the over-smoothing problem of traditional GNNs and shows promising results in terms of classification accuracy, computational cost and learning with very small quantity of labeled data.
更多查看译文
关键词
community detection,transductive learning,graph neural network,inductive learning
AI 理解论文
溯源树
样例
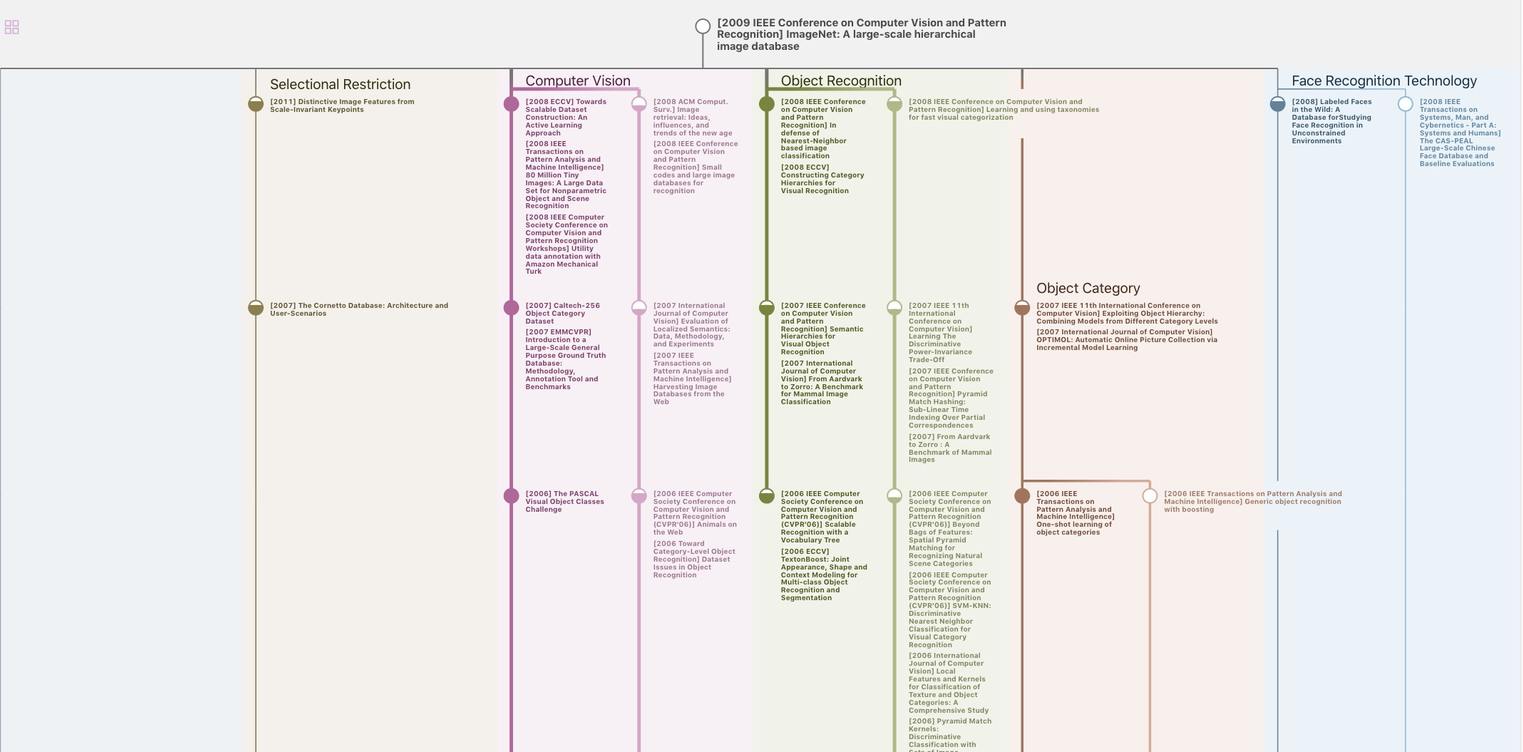
生成溯源树,研究论文发展脉络
Chat Paper
正在生成论文摘要