Interaction-Focused Anomaly Detection on Bipartite Node-and-Edge-Attributed Graphs.
IJCNN(2023)
Abstract
Many anomaly detection applications naturally produce datasets that can be represented as bipartite graphs (userinteraction-item graphs). These graph datasets are usually supplied with rich information on both the entities (nodes) and the interactions (edges). Unfortunately, previous graph neural network anomaly models are unable to fully capture the rich information and produce high-performing detections on these graphs, as they mostly focus on homogeneous graphs and node attributes only. To overcome the problem, we propose a new graph anomaly detection model that focuses on the rich interactions in bipartite graphs. Specifically, our model takes a bipartite node-and-edge-attributed graph and produces anomaly scores for each of its edges and then for each of its bipartite nodes. We design our model as an autoencoder-type model with a customized encoder and decoder to facilitate the compression of node features, edge features, and graph structure into node-level latent representations. The reconstruction errors of each edge and node are then leveraged to spot the anomalies. Our network architecture is scalable, enabling large real-world applications. Finally, we demonstrate that our method significantly outperforms previous anomaly detection methods in the experiments.
MoreTranslated text
Key words
Anomaly Detection, Graph Neural Networks
AI Read Science
Must-Reading Tree
Example
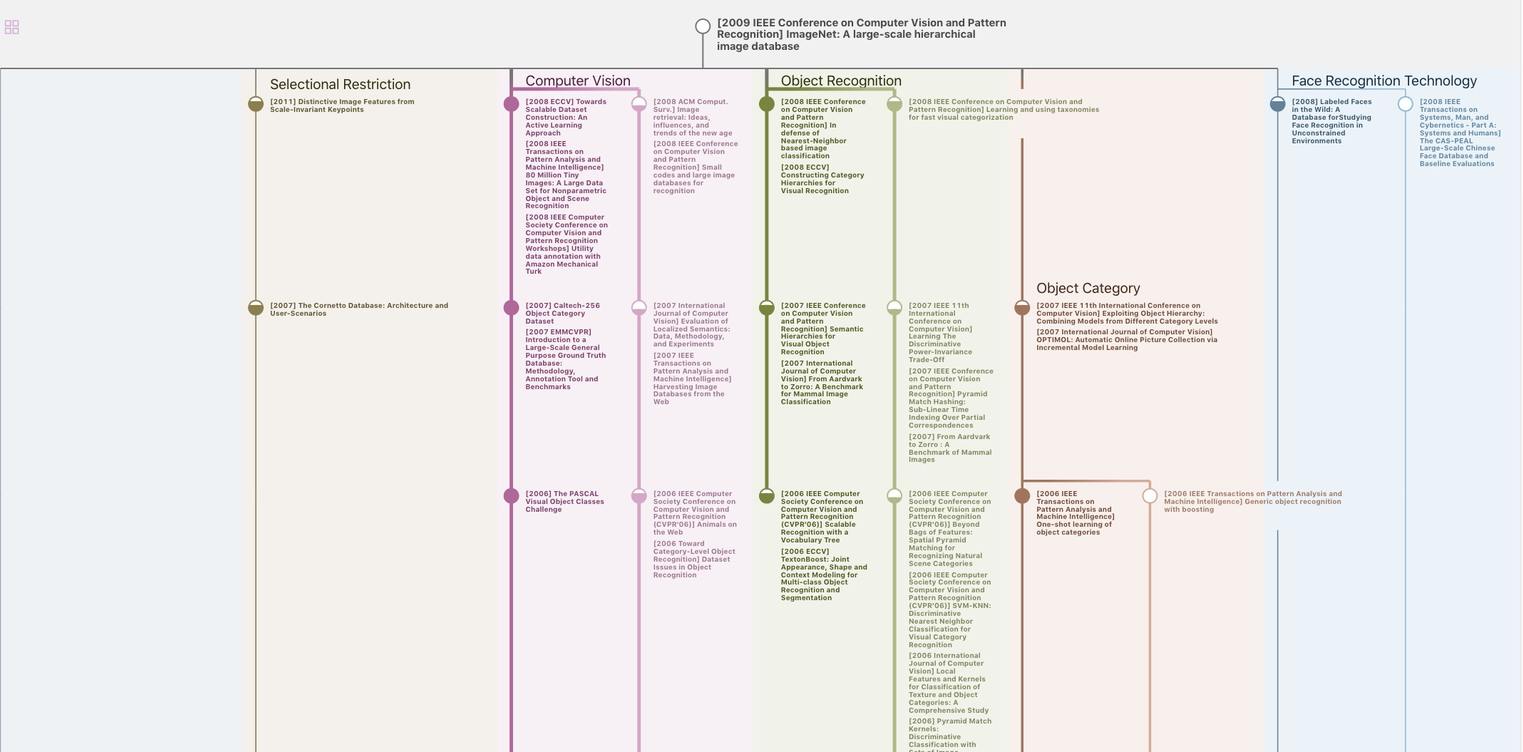
Generate MRT to find the research sequence of this paper
Chat Paper
Summary is being generated by the instructions you defined