Document-level Relation Extraction with Entity Interaction and Commonsense Knowledge
IJCNN(2023)
Abstract
Document-Level Relation Extraction(DLRE) is a more challenging task than sentence-level relation extraction because of the characteristics such as more extended context, more interactions between entities, and the need for common sense to help the relation inference. In this paper, we propose an effective model to address the problems of complex entity interactions and the lack of commonsense knowledge. Specifically, we propose a Transformer-based entity interaction module instead of graph neural networks to model the correlation across entities, thus avoiding the information loss problem triggered by predefined edge-building rules. In addition, the initial word vector from the word embedding layer of a pre-trained language model is injected into entity representation to boost the performance in the extraction of relational facts that need commonsense knowledge. Experiments show that our model obtains competitive performance, especially compared with graph-based methods, which is faster and more effective. The source code, trained checkpoint files, and the commit results will be released to the public.
MoreTranslated text
Key words
relation extraction,entity interaction,common-sense knowledge,residual connection
AI Read Science
Must-Reading Tree
Example
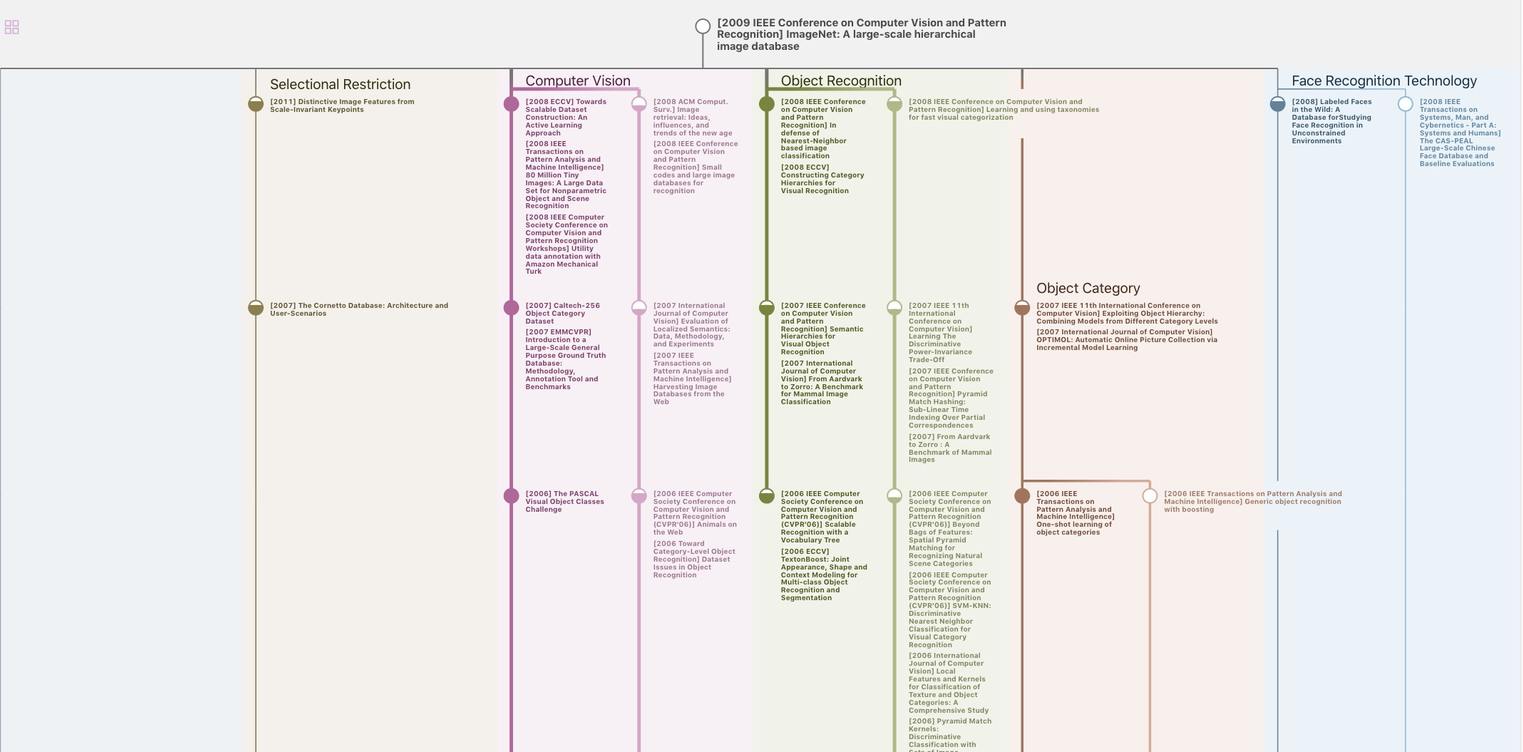
Generate MRT to find the research sequence of this paper
Chat Paper
Summary is being generated by the instructions you defined