Invariant and Sufficient Supervised Representation Learning
2023 INTERNATIONAL JOINT CONFERENCE ON NEURAL NETWORKS, IJCNN(2023)
摘要
Improving the generalization of neural networks under domain shift is an important and challenging task in computer vision. Obtaining an invariant representation across domains is a benchmark method in the literature. In this paper, we propose an invariant and sufficient supervised representation learning (ISSRL) approach to learn a domain invariant representation which is also preserving information used for downstream tasks. To this end, we formulate ISSRL by finding a nonlinear map g such that Y perpendicular to X|g(X) and (Y, g(X)) perpendicular to D at the population level, where D is the label of the domains and (X, Y) is the paired data sampled from domains with label. We use distance correlation to characterize the (conditional) independence. At the sample level, we construct a novel loss function through an unbiased empirical version of distance correlation. We train the representation map by parameterizing it with deep neural networks. Both simulation study and real data evaluation show that ISSRL outperforms the state-of-theart on out-of-distribution generalization. The PyTorch code for ISSRL is available at https://github.com/CaC033/ISSRL.
更多查看译文
关键词
domain generalization,representation learning,invariant,sufficient
AI 理解论文
溯源树
样例
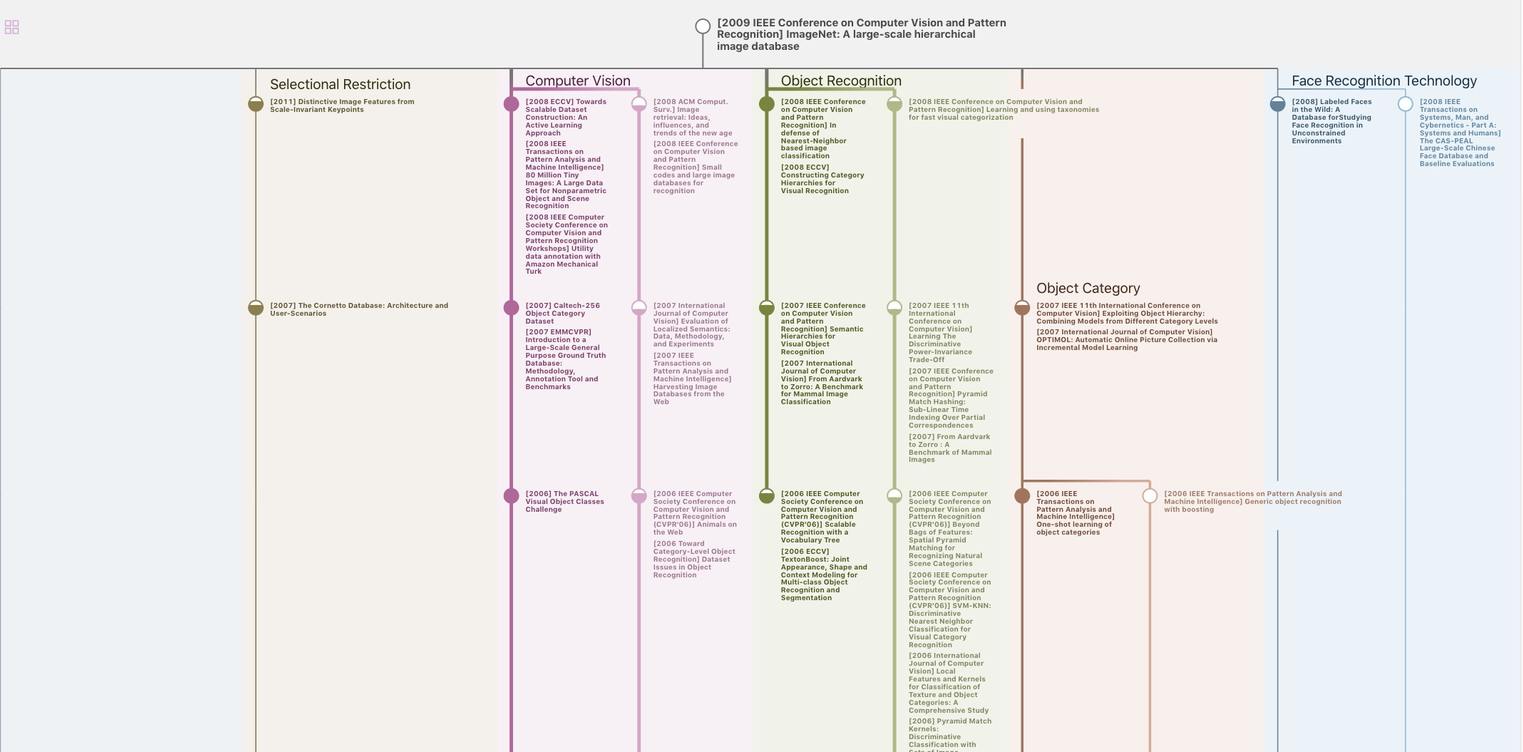
生成溯源树,研究论文发展脉络
Chat Paper
正在生成论文摘要