EEG Model Compression by Network Pruning for Emotion Recognition.
IJCNN(2023)
摘要
With the development of deep learning on EEG-related tasks, the complexity of learning models has gradually increased. Unfortunately, the insufficient amount of EEG data limits the performance of complex models. Thus, model compression becomes an option to be seriously considered. So far, in EEG-related tasks, although some models used lightweight means such as separable convolution in their models, no existing work has directly attempted to compress the EEG model. In this paper, we try to investigate the state-of-the-art network pruning methods on commonly used EEG models for the emotion recognition task. In this work, we make several surprising observations that contradict common beliefs. Training a pruned model from scratch outperforms fine-tuning a pruned model with inherited weights, which means that the pruned structure itself is more important than the inherited weights. We can ignore the entire pruning pipeline and train the network from scratch using the predefined network architecture. We substantially reduce the computational resource overhead of the model while maintaining accuracy. In the best case, we achieve a 62.3% reduction in model size and a 64.3% reduction in computing operations without accuracy loss.
更多查看译文
关键词
Model compression, Emotion BCI, Network pruning, EEG model
AI 理解论文
溯源树
样例
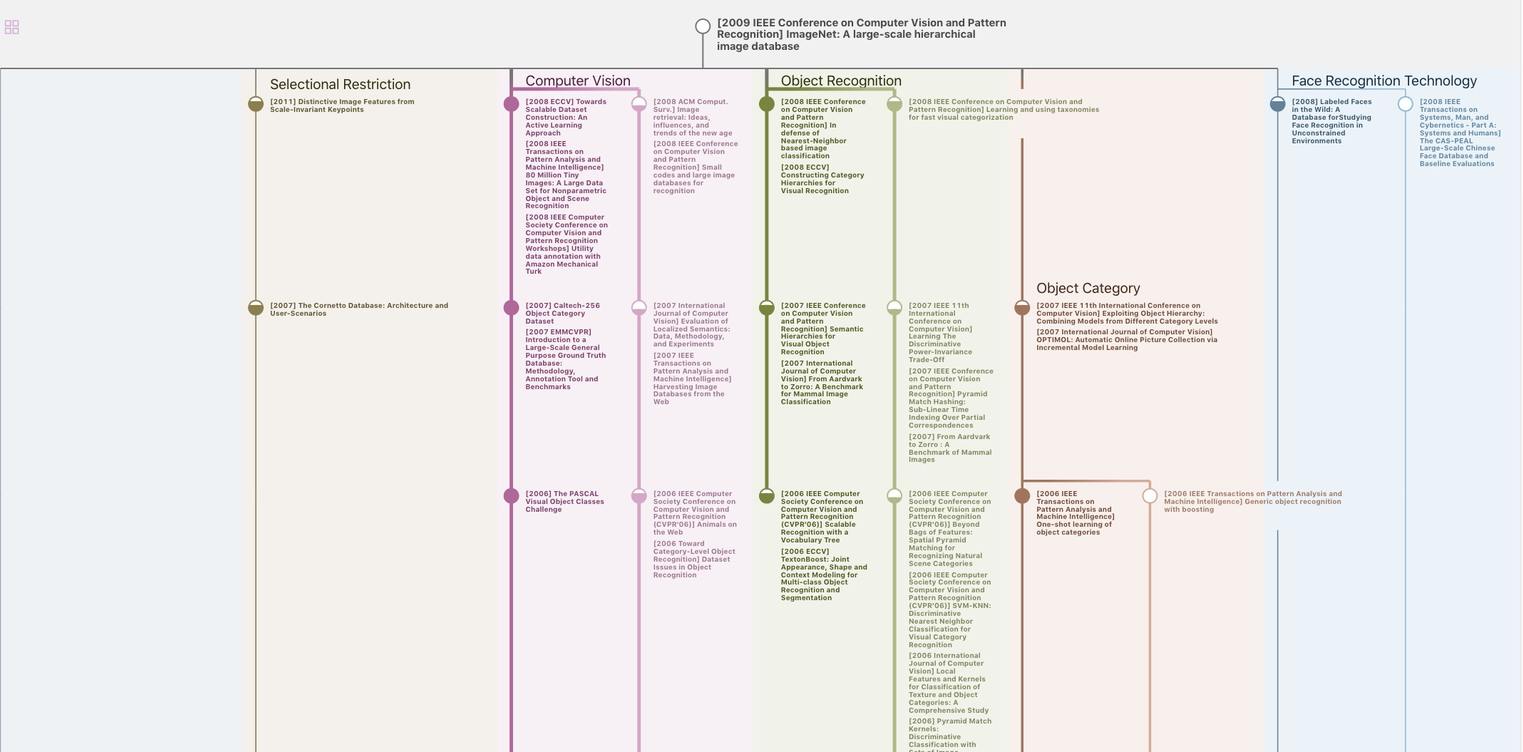
生成溯源树,研究论文发展脉络
Chat Paper
正在生成论文摘要