One-step Domain Adaptation Approach with Partial Label.
IJCNN(2023)
摘要
Unsupervised Domain adaptation (UDA) aims to train a target classifier by using massive accurate annotated source domain data and unlabeled target domain data. However, collecting massive accurate annotation can be labor-intensive, and even impractical especially when source domain training data shows label ambiguity. In this paper, we consider a novel domain adaptation setting where the model can be trained with partial-labeled source domain data, so that the cost of data labeling can be reduced. To alleviate the ambiguity induced by partial label, we propose a one-step domain adaptation approach trained from the partial-labeled source data and unlabeled target data. Our approach consists of two components: a feature extractor equipped with partial label loss that learns discriminative representation, and a domain classifier that learns domain-invariant representation across the source and target domains. Extensive experiments have shown that the proposed approach significantly outperforms a series of competitive baselines.
更多查看译文
关键词
machine learning,transfer learning,domain adaptation,partial label learning
AI 理解论文
溯源树
样例
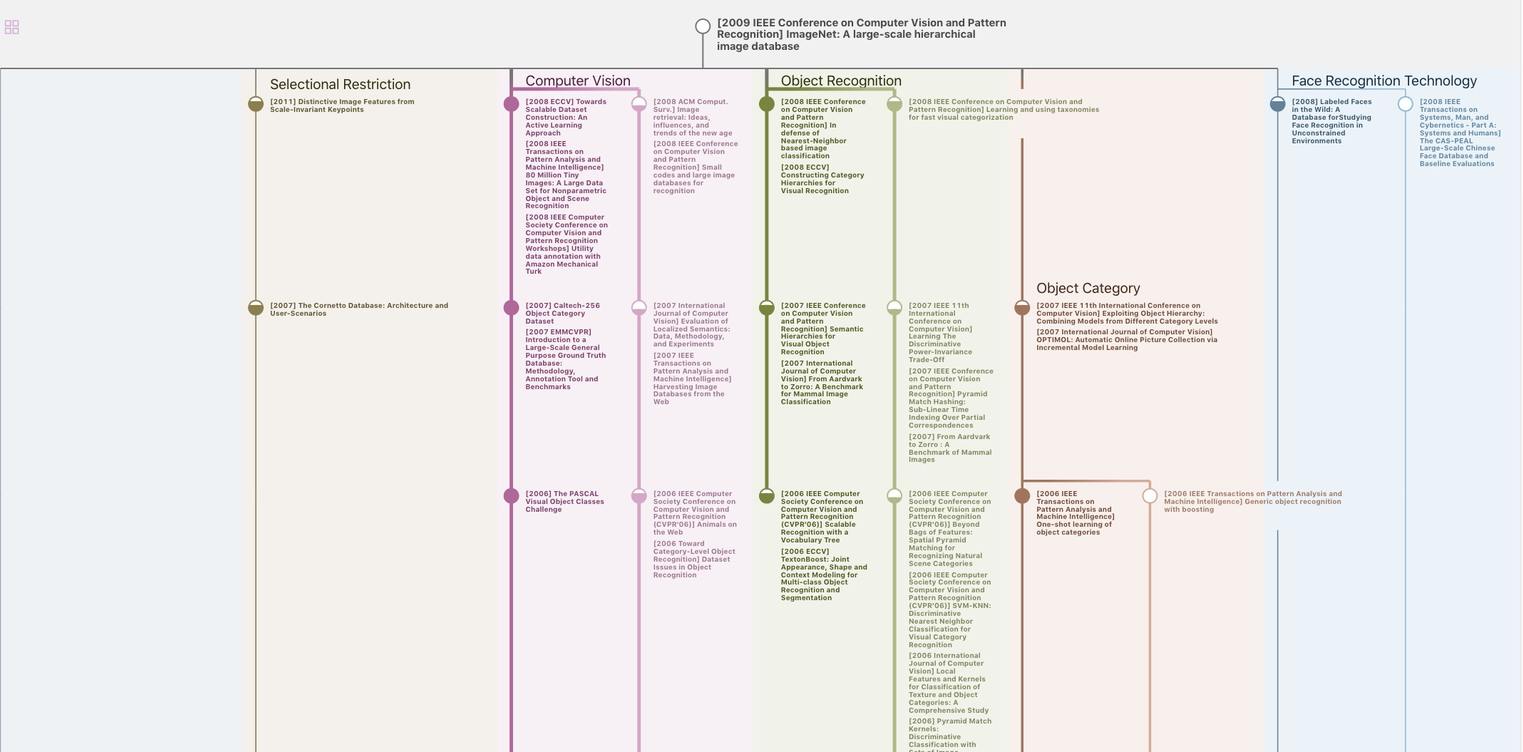
生成溯源树,研究论文发展脉络
Chat Paper
正在生成论文摘要