Self-calibrated U-Net for Document Segmentation.
IJCNN(2023)
摘要
Based on the need to digitalize identification documents per several institutions and companies, the segmentation task of textual information acquired importance. Commonly, Convolutional Neural Networks are applied to solve such problems. Vanilla convolution in deep learning models can provide sub-optimal performance in some learning tasks, such as semantic segmentation. Due to this, more specific proposals may be applied to better fit this context, such as self-calibrated convolutions, which consider unique characteristics of different feature maps. Considering this context, we propose a new fully convolutional network architecture based on U-Net for segmentation associated with self-calibrated convolution. We consider changing all vanilla convolution layers in this new neural network by self-calibrating convolutions. In this work, we evaluate our proposal in three different tasks of document segmentation. All experiments demonstrate an increase in the proposed neural network's segmentation performance compared with traditional U-Net in the analyzed context, but the inference time performance decreases.
更多查看译文
关键词
commonly networks,convolutional network architecture,convolutional neural networks,deep learning models,document segmentation,identification documents,self-calibrated U-Net,self-calibrating convolutions,semantic segmentation,textual information,vanilla convolution layers
AI 理解论文
溯源树
样例
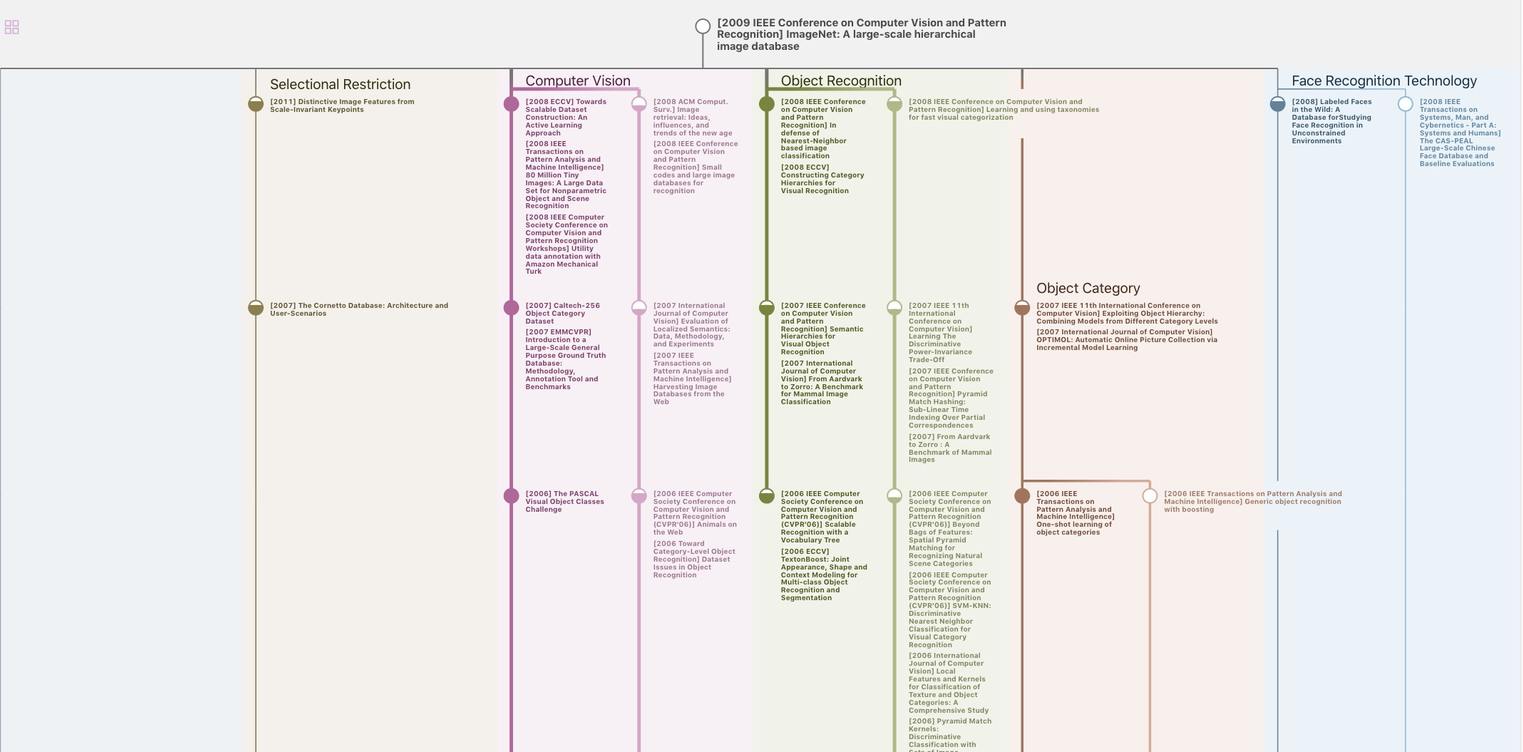
生成溯源树,研究论文发展脉络
Chat Paper
正在生成论文摘要