TransD-based Multi-hop Meta Learning for Few-shot Knowledge Graph Completion.
IJCNN(2023)
摘要
Few-shot knowledge graph completion (FKGC), which aims to infer missing facts about a relation from only a few reference triples, has recently attracted great attention. The core of solving the FKGC task is to learn a vector representation for each few-shot relation using the corresponding entity representations. To this end, existing models generally enhance entity representations with their direct neighbors. However, a large number of entities have few direct neighbors. Hence, encoding only direct neighborhood is insufficient to obtain satisfactory entity representations. In addition, current models typically utilize static embeddings to represent entities, ignoring their diverse semantics, i.e., an entity may show distinct semantics within different few-shot relations. To address these issues, we propose a new FKGC framework, namely TransD-based Multi-hop Meta Learning (TDML). TDML consists of three main components: a multi-hop neighbor encoder to enhance entity representations by aggregating heterogeneous multi-hop neighbors, a transformer encoder to generate the relation meta representations, and a TransD-based relation representation updater that allows each entity to exhibit relation-specific semantics and tune the relation meta representations. Extensive experiments on two public datasets demonstrate that our model outperforms state-of-the-art FKGC methods.
更多查看译文
关键词
Knowledge Graph Completion,Few-shot Relation,Multi-hop Neighborhoods,Meta Learning
AI 理解论文
溯源树
样例
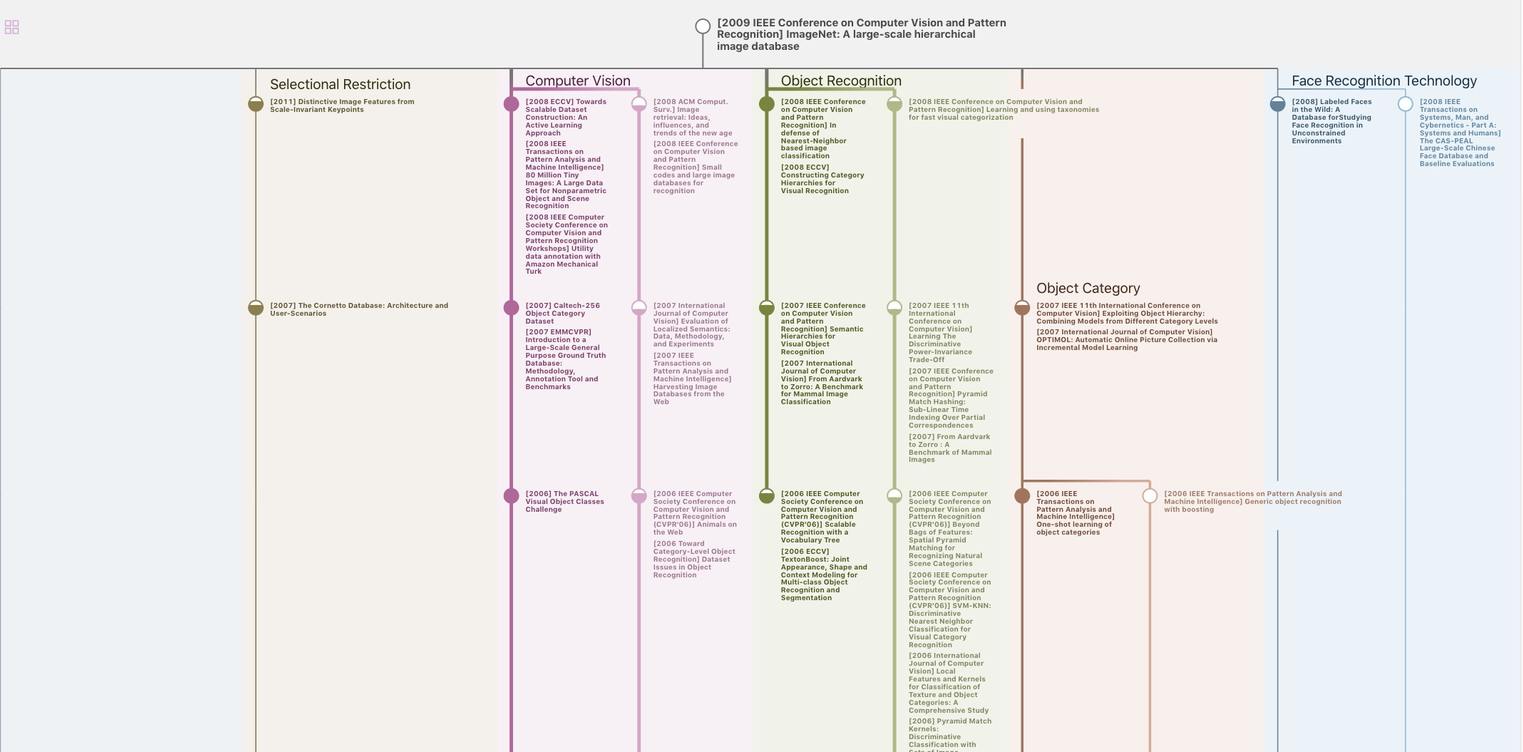
生成溯源树,研究论文发展脉络
Chat Paper
正在生成论文摘要