Speech Emotion Recognition Using Dual Global Context Attention and Time-Frequency Features.
IJCNN(2023)
摘要
Speech emotion recognition (SER) has become an important part of human-computer interaction. With the help of deep learning methods such as the attention mechanism, improved performance is achieved for SER. However, the emotional features of speech are still not fully extracted and utilized. In this paper, we propose a speech emotion recognition model based on dual global context attention (GCA) and time-frequency features. First, time-frequency features are extracted from 3D Log-Mel spectrograms of speech using parallel 2D CNNs. Then, a dual GCA architecture is designed to analyze the high-level features. The first GCA block, as well as a CNN, learns the high-level temporal and spectral features; the second one discovers the correlation between network layers, and further analyzes the fusion of high-level features to obtain global context information. Experimental results show that competitive SER performance is obtained. The proposed model achieves recognition accuracies of 70.08%, 86.67% and 93.27% on the IEMOCAP, RAVDESS and EMO-DB datasets, respectively. The model size is only 0.45M, which is small for SER tasks.
更多查看译文
关键词
speech emotion recognition,time-frequency features,global context attention
AI 理解论文
溯源树
样例
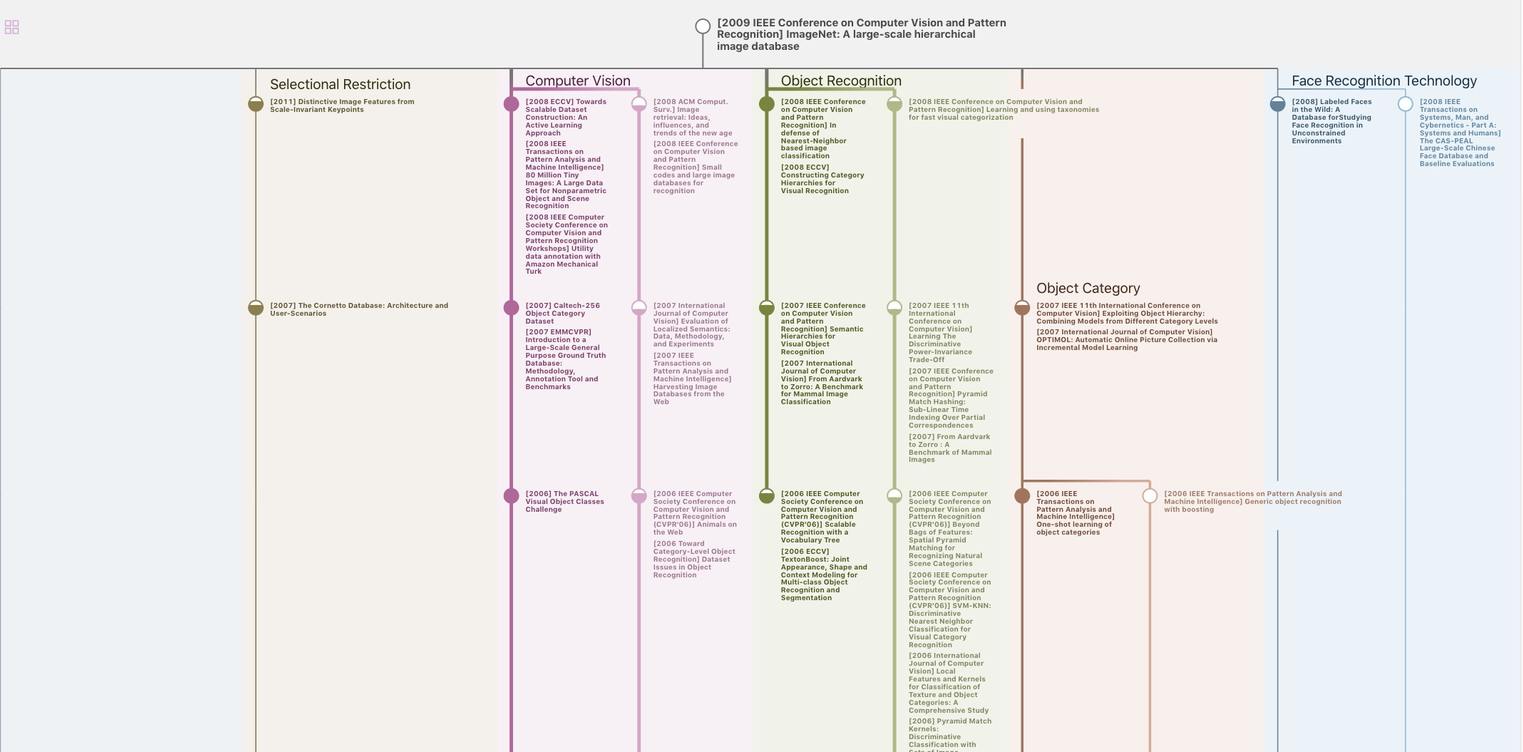
生成溯源树,研究论文发展脉络
Chat Paper
正在生成论文摘要