Improving Relation Extraction by Entity-Level Contrastive Learning
IJCNN(2023)
摘要
Relation Extraction (RE) is a fundamental task in Natural Language Processing (NLP), which aims to extract relations between entity pairs mentioned in a given sentence. Recently, relation extraction has achieved remarkable process with the development of deep neural network. However, most of the recent works focus on using external knowledge to pretrain model or encoding entity related information with graph neural network. In this paper, we propose a BERT-based model with entity-level contrastive learning module based only on the original input sentences for relation extraction. By optimizing the contrastive learning and relation classification objectives jointly, our model is more effective in encoding relation representation between two entities in a given sentence and achieves better relation extraction performance. Experiments conducted on two mainstream RE datasets show that our model significantly outperforms the baselines' results on two benchmark datasets. Meanwhile, the results of our model are very competitive with the state-of-the-art models which employ extra training datas or information.
更多查看译文
关键词
relation extraction,contrastive learning,entity-level information,representation learning
AI 理解论文
溯源树
样例
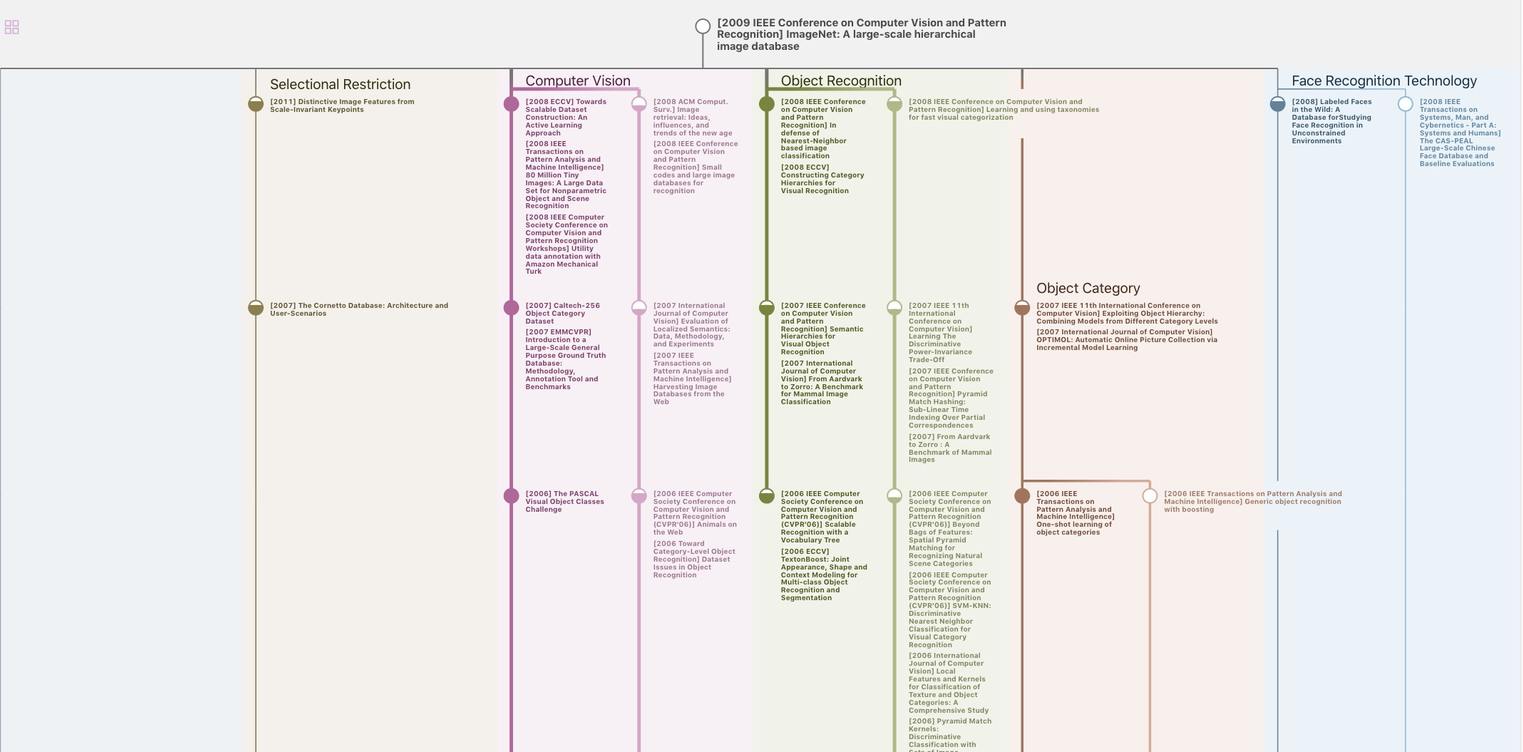
生成溯源树,研究论文发展脉络
Chat Paper
正在生成论文摘要